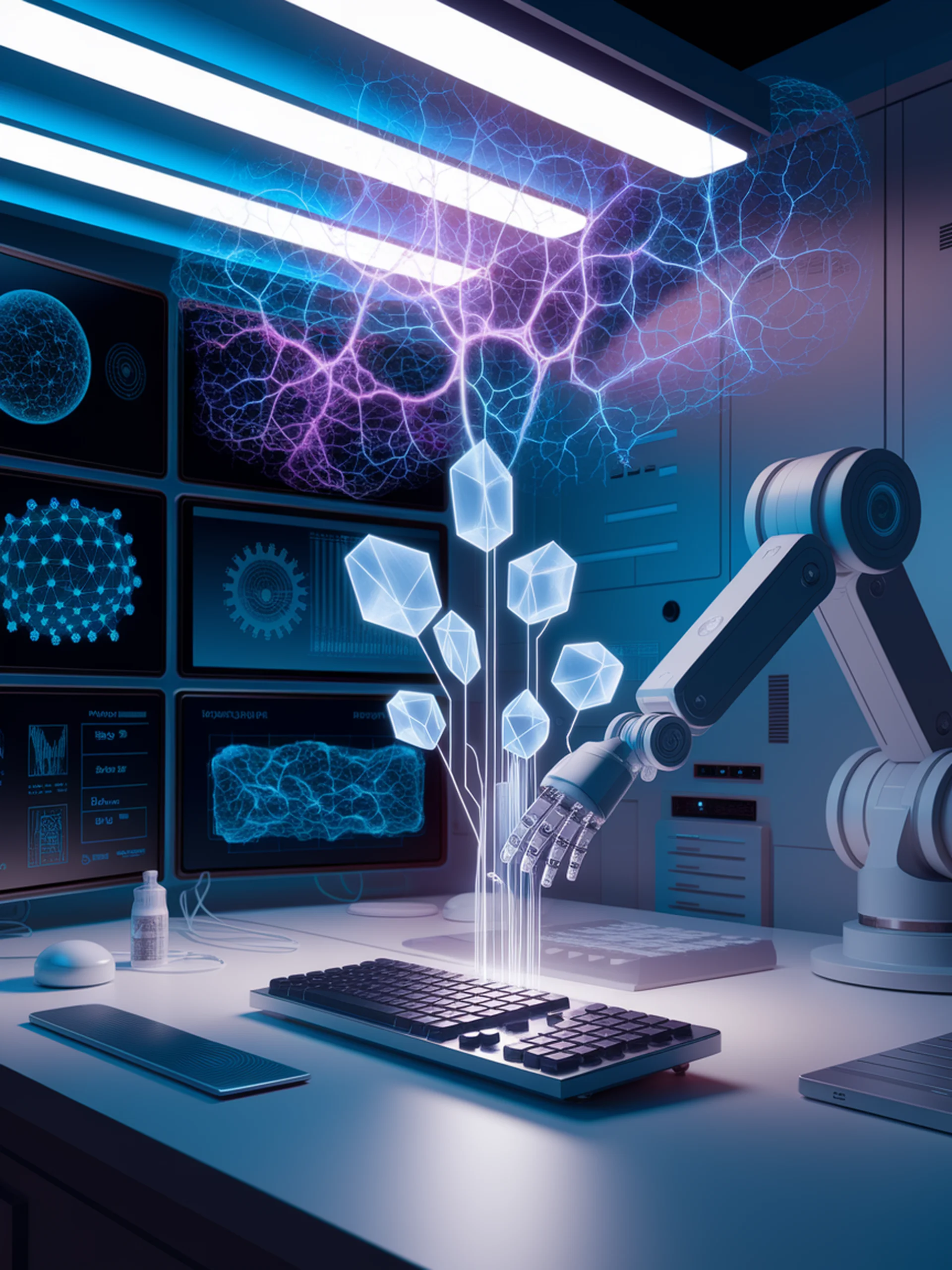
Advanced Reasoning and Adaptability
Making AI Agents Smarter and More Flexible
Current Limitations
- Today's agents struggle with complex long-term planning
- Limited common-sense reasoning about physical and social worlds
- Difficulty adapting to situations that differ from training data
- Computational intensity restricting deployment scenarios
Neuro-symbolic Approaches
- Combining neural networks with symbolic reasoning
- Integrating structured knowledge representations
- Enabling logical deduction and inference
- Supporting causal reasoning about events and actions
Continuous Learning Capabilities
- Lifelong learning without catastrophic forgetting of previous knowledge
- Online reinforcement learning improving from real-world interaction
- Meta-learning or "learning to learn" for faster adaptation
- Transfer learning applying knowledge across different domains
Efficiency Improvements
- Model sparsity reducing computational requirements
- Distillation of large models into smaller, deployable versions
- Neuromorphic computing approaches mimicking brain efficiency
- Algorithm optimization for resource-constrained environments
Research Milestones to Achieve
- Agents that can reliably solve multi-step problems requiring planning
- Context retention across extended interactions
- Self-correction when facing errors or unexpected outcomes
- Computational models of causality supporting "what if" reasoning
"Truly autonomous AI will need big leaps in contextual reasoning abilities and far more robust handling of edge cases than we have today. This isn't just about bigger models—it requires fundamental advances in how AI represents and reasons about the world."