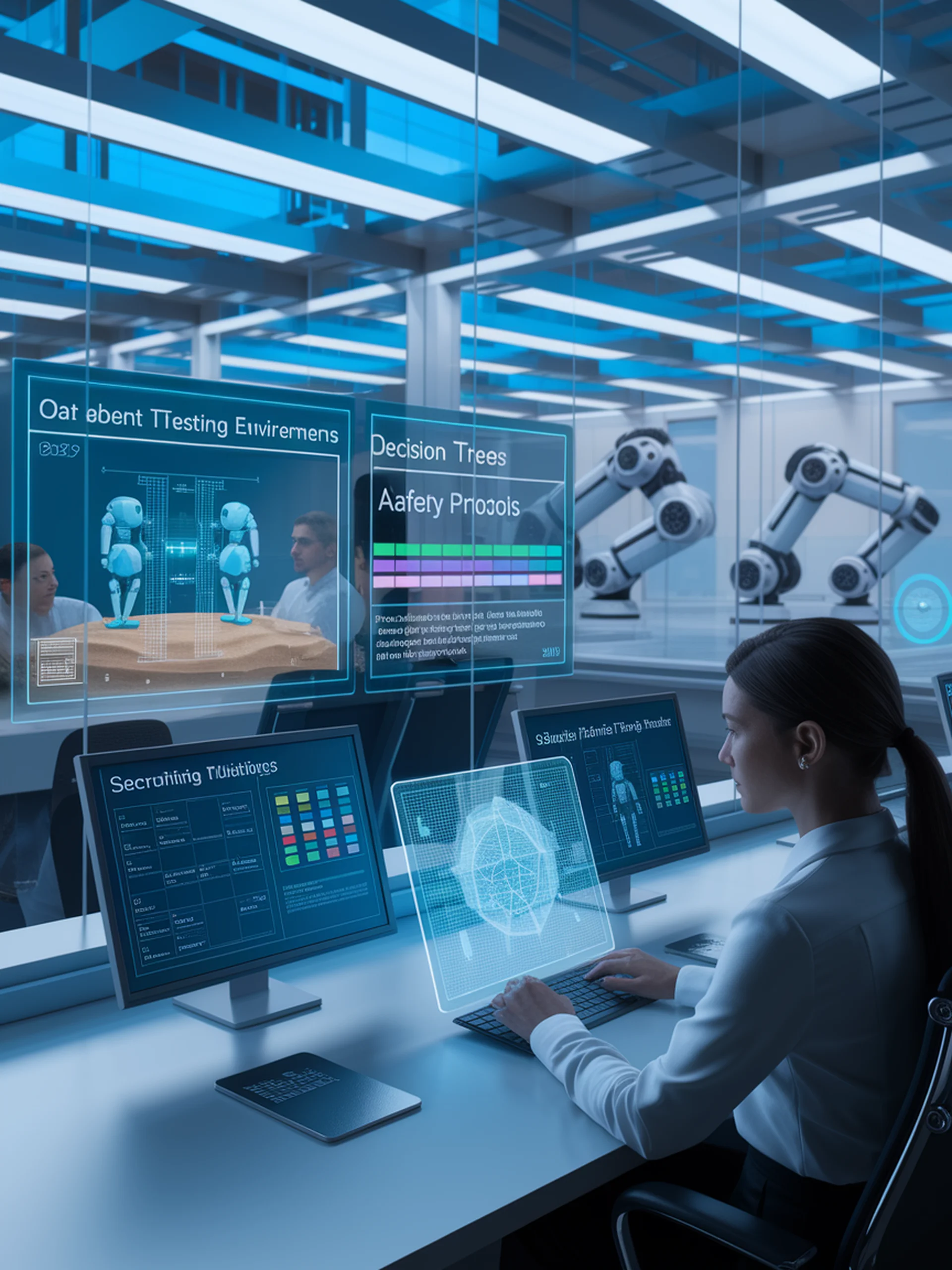
Robustness, Safety, and Ethics
Ensuring Reliable, Beneficial AI Agents
Critical Safety Challenges
- Preventing cascading errors in autonomous decision loops
- Avoiding reward hacking or unexpected optimization strategies
- Managing distributional shift when deployed conditions differ from training
- Ensuring robust performance across diverse scenarios
Technical Safety Research
- Formal verification mathematically proving system properties
- Sandboxed testing environments for safe experimentation
- Adversarial testing identifying potential failure modes
- Interpretability techniques revealing agent decision processes
Alignment with Human Values
- Value learning from human preferences and feedback
- Reinforcement learning from human feedback (RLHF) for alignment
- Constitutional AI approaches embedding ethical guidelines
- Nested optimization preventing pursuit of instrumental goals
Fairness and Ethical Frameworks
- Algorithmic fairness preventing harmful discrimination
- Transparency requirements for high-stakes decisions
- Accountability mechanisms when systems cause harm
- Cultural context sensitivity across diverse societies
Governance Through Design
- AI ethics by design principles integrated throughout development
- Fail-safe mechanisms ensuring safe degradation under uncertainty
- Human oversight interfaces at appropriate intervention points
- Audit trails and logging for retrospective analysis
"The paramount challenge for advanced AI agents isn't just capability, but alignment—ensuring these increasingly autonomous systems reliably pursue the goals we actually intend, avoid harmful strategies, and operate within ethical boundaries even as they become more powerful. Safety research isn't separate from capability research; it's an essential component."