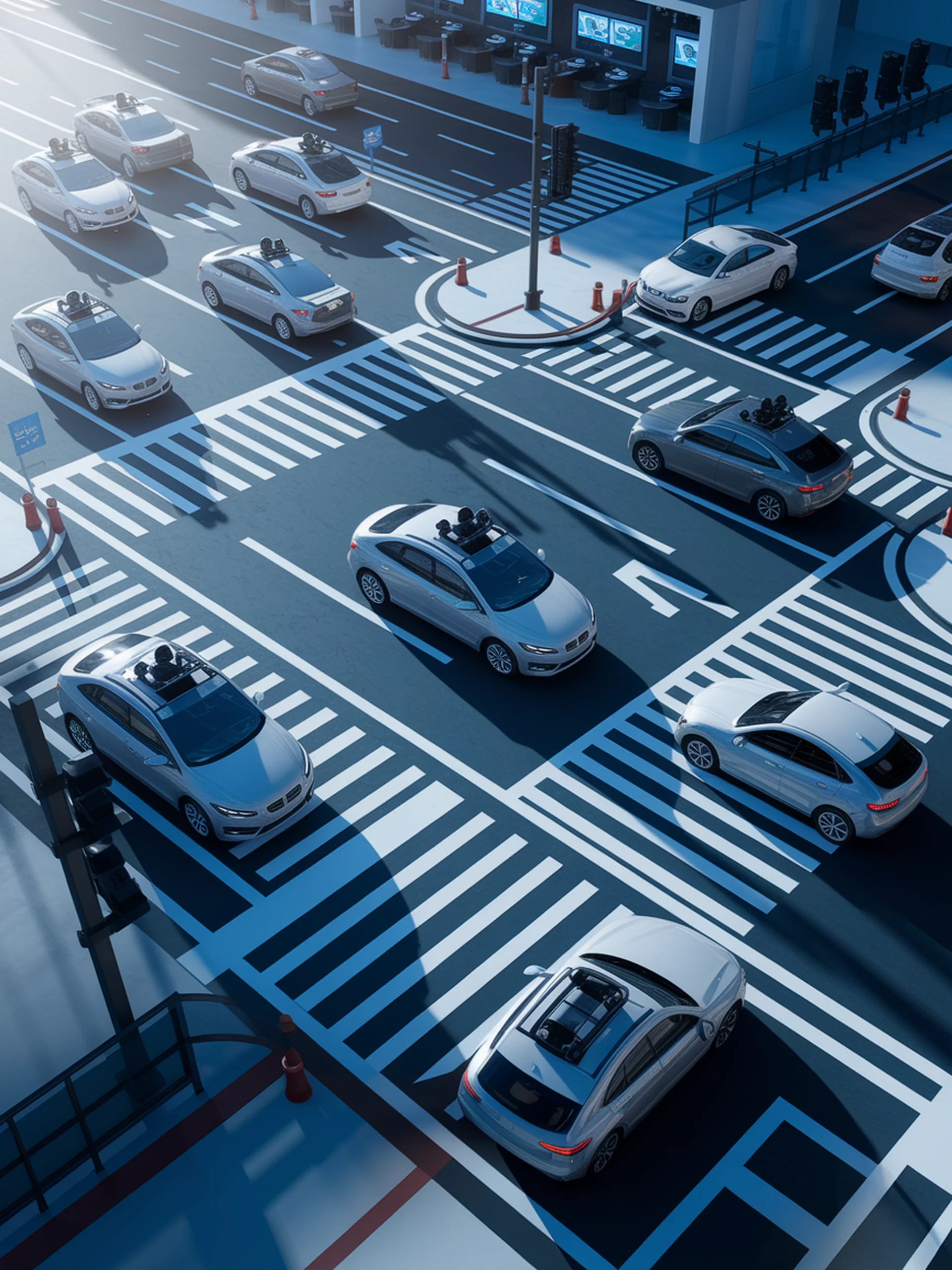
Reimagining Traffic Simulation Models
Closing the loop in tokenized traffic agent simulations
This research advances traffic simulation by addressing the covariate shift problem that occurs when tokenized multi-agent policies are deployed in closed-loop environments.
- Introduces a novel supervised fine-tuning approach specifically designed for closed-loop traffic simulation
- Leverages tokenized multi-agent policies inspired by large language models
- Demonstrates improved performance in the Waymo Sim Agent Challenge, creating more realistic traffic simulations
- Bridges the gap between open-loop training and closed-loop execution in autonomous driving scenarios
This innovation matters for engineering applications by enabling more realistic traffic simulations for autonomous vehicle testing, potentially reducing real-world testing requirements and accelerating AV development.
Closed-Loop Supervised Fine-Tuning of Tokenized Traffic Models