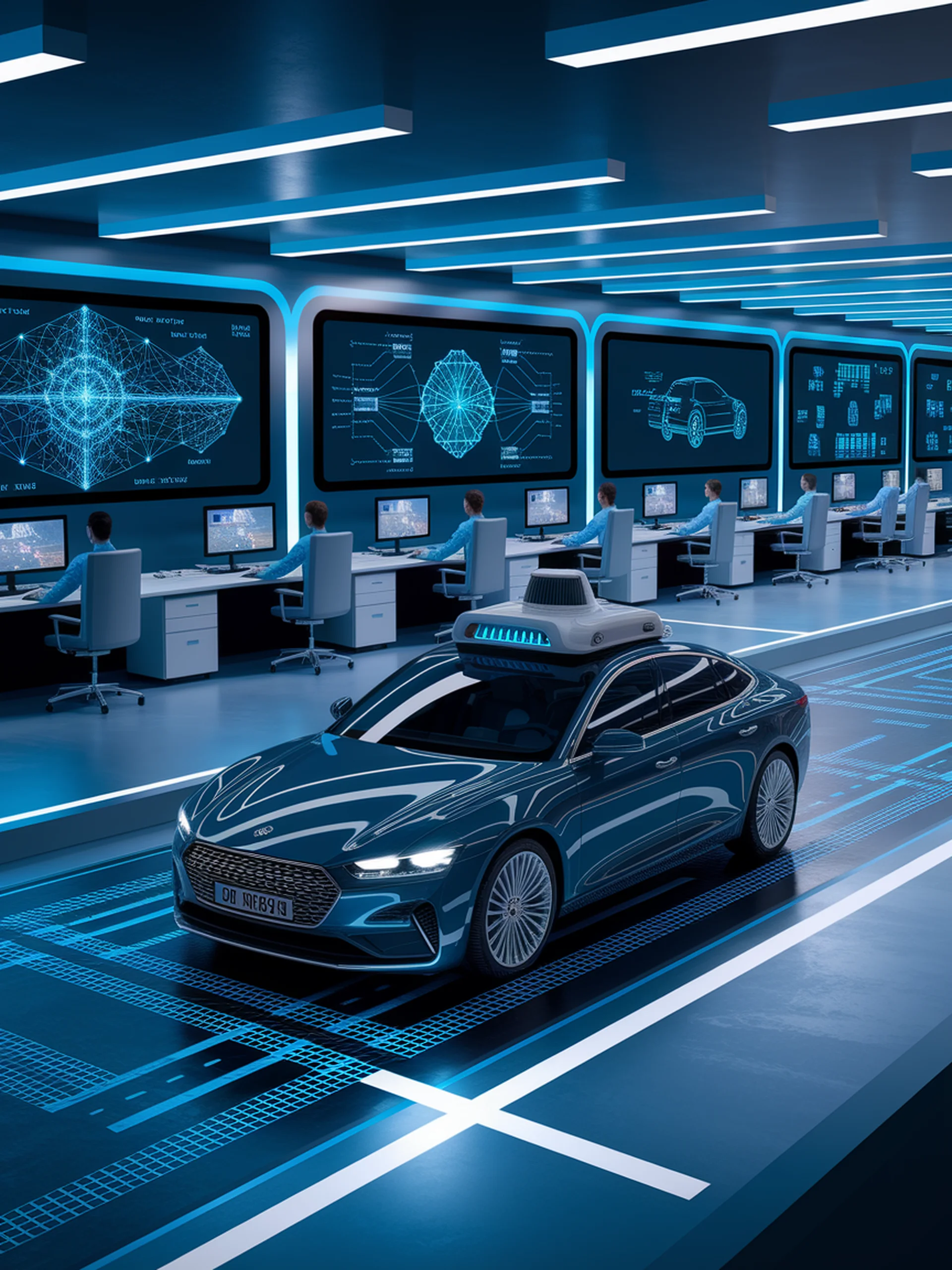
Smart Hybrid Driving: LLMs Meet Reinforcement Learning
Combining AI approaches for safer, more efficient autonomous vehicles
TeLL-Drive combines the planning capabilities of Large Language Models with the real-time performance of Deep Reinforcement Learning to create more effective autonomous driving systems.
- Creates a teacher-student framework where LLMs guide reinforcement learning policies
- Incorporates risk metrics and historical scenario retrieval to enhance safety
- Achieves better decision-making while maintaining real-time performance
- Addresses DRL's sample efficiency limitations through LLM guidance
This research matters for engineering autonomous systems that can both understand complex driving contexts and execute decisions efficiently—bringing us closer to reliable self-driving technology that can handle real-world complexity.
TeLL-Drive: Enhancing Autonomous Driving with Teacher LLM-Guided Deep Reinforcement Learning