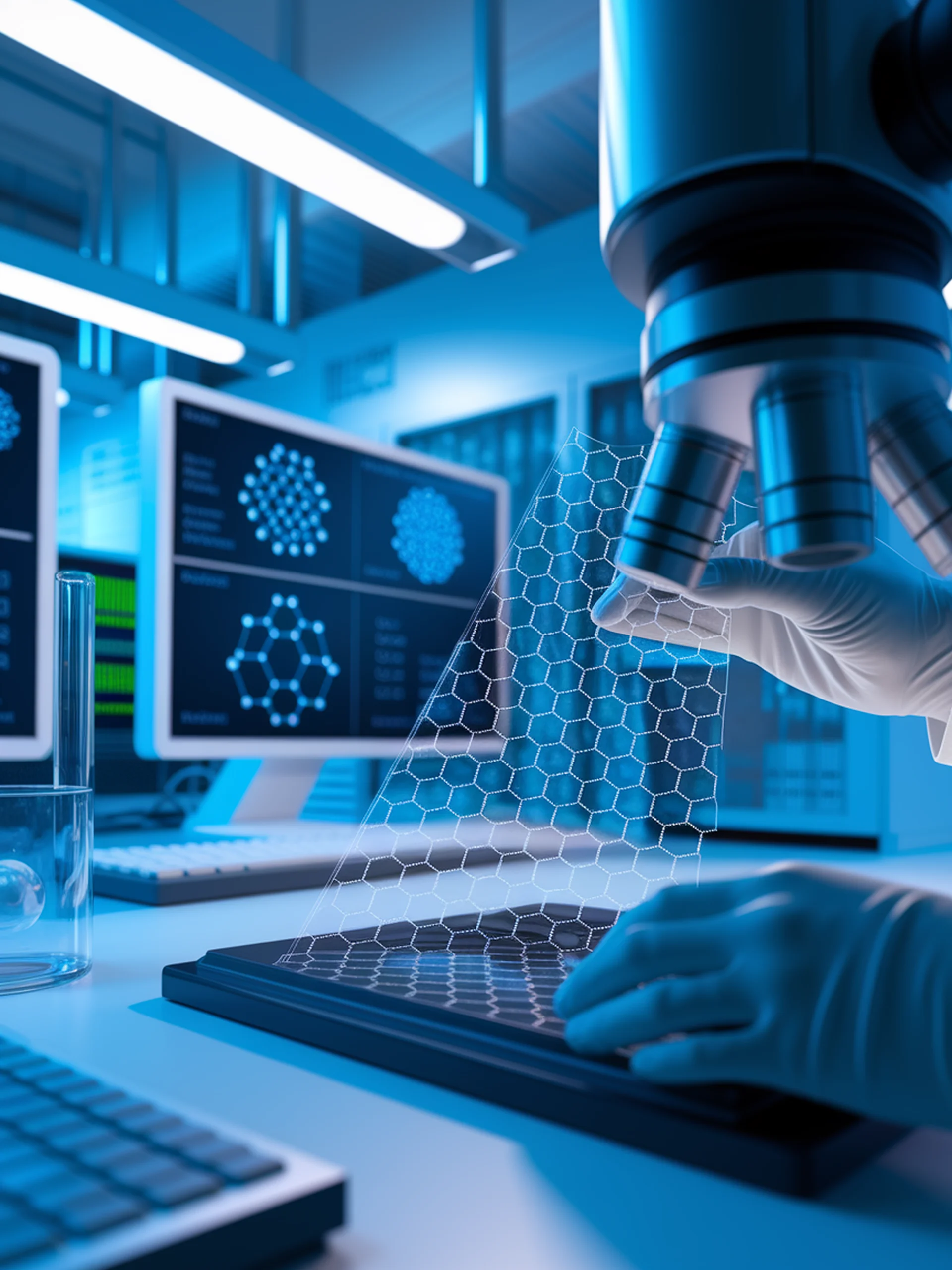
Overcoming Data Scarcity with LLMs
How Large Language Models are transforming materials engineering
This research demonstrates how Large Language Models can address critical data limitations in materials science, specifically for graphene synthesis optimization.
- Tackles the challenge of scarce experimental data in materials science by leveraging LLMs
- Enables efficient extraction and structuring of synthesis data from scientific literature
- Combines discrete and continuous features effectively for improved machine learning models
- Creates a pathway for accelerating materials discovery and optimization despite limited datasets
This approach significantly reduces the engineering bottlenecks caused by costly and time-consuming experiments, potentially accelerating innovation cycles in advanced materials development.