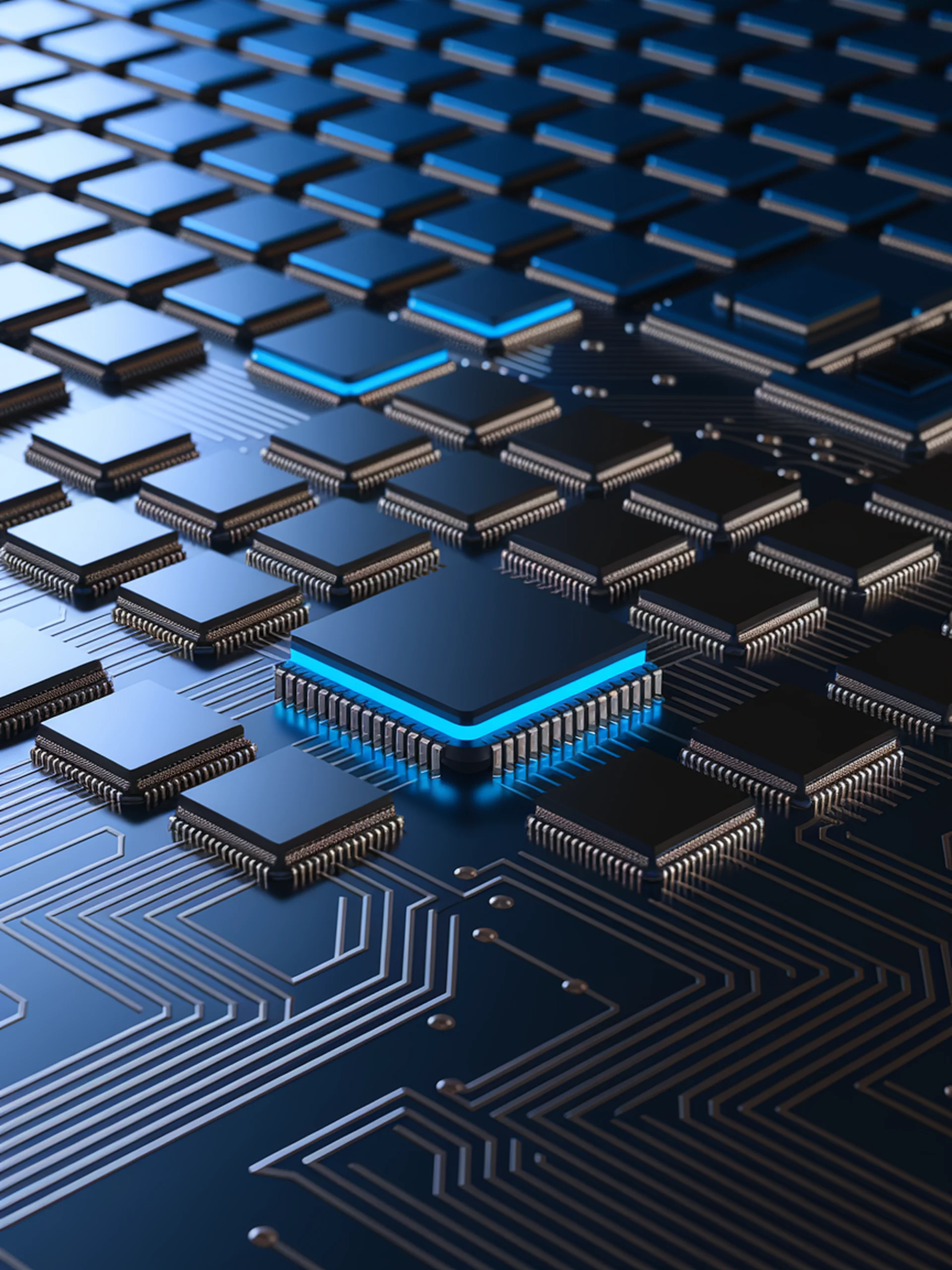
Optimizing MoE Models with Smart Quantization
Structure-aware compression for large language models
This research introduces QuantMoE-Bench, the first comprehensive framework for evaluating post-training quantization techniques specifically designed for Mixture-of-Experts (MoE) language models.
- Achieves up to 4x compression while maintaining model performance
- Demonstrates that structure-aware quantization outperforms uniform quantization
- Proposes adaptive bit allocation methods that consider the unique sparse activation patterns in MoE models
- Establishes a standardized benchmark for evaluating MoE quantization techniques
For engineering teams, this research offers practical methods to deploy massive MoE language models with significantly reduced memory requirements, making advanced AI more accessible and cost-effective for production environments.
QuantMoE-Bench: Examining Post-Training Quantization for Mixture-of-Experts