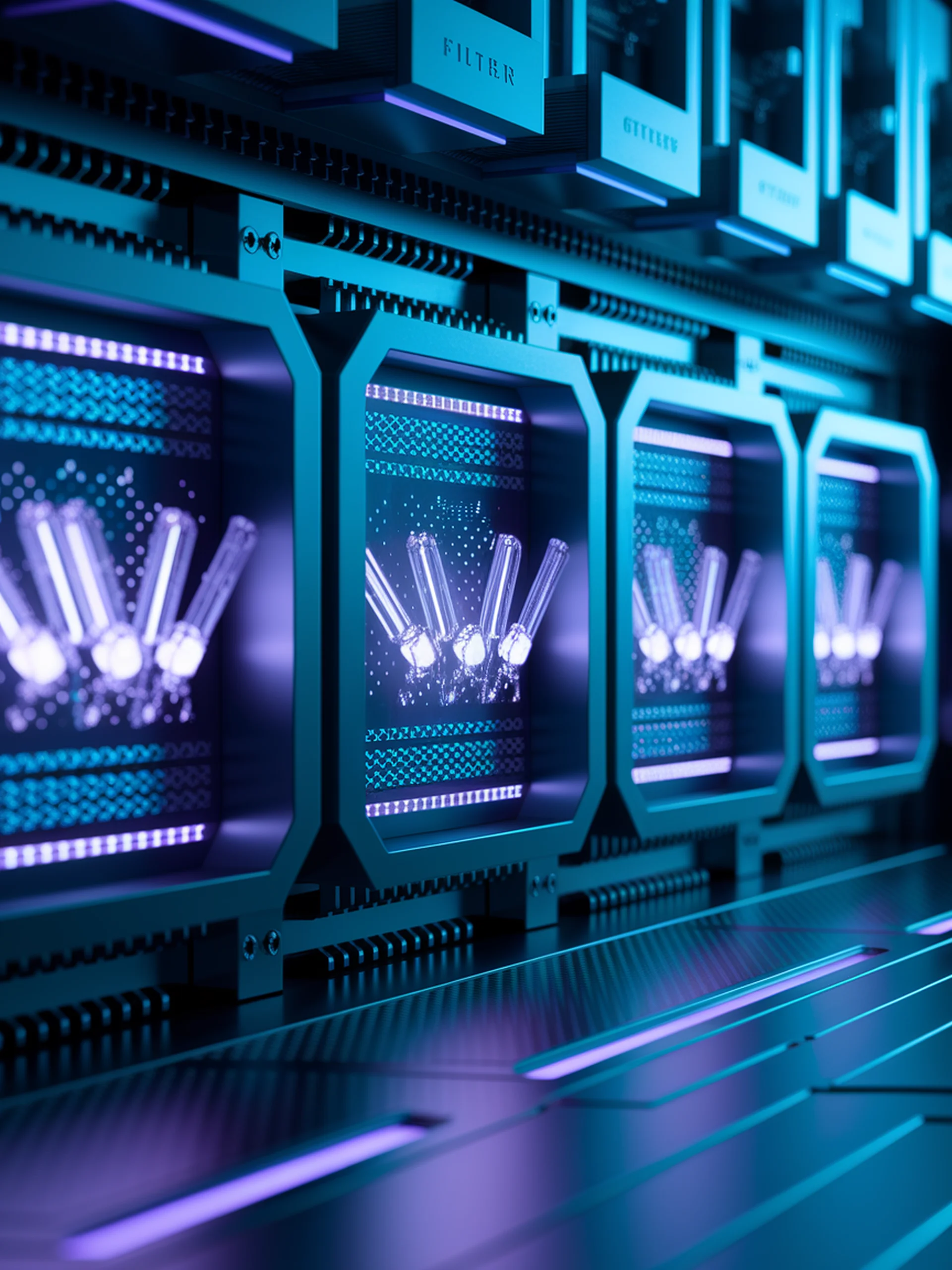
Accelerating Multimodal LLMs
Training-Free Token Reduction for Efficient MLLM Deployment
This research introduces a novel filter-correlate-compress framework that significantly reduces computational costs for multimodal LLMs without requiring retraining.
- Addresses the quadratic complexity problem that hampers real-world MLLM deployment
- Precisely identifies and filters redundant visual tokens while preserving essential information
- Enables more efficient inference without sacrificing model performance
- Offers a practical engineering solution for deploying MLLMs in resource-constrained environments
This advancement matters for engineering teams building multimodal AI applications, as it provides a straightforward approach to optimize existing models without the computational expense of retraining or fine-tuning.
Filter, Correlate, Compress: Training-Free Token Reduction for MLLM Acceleration