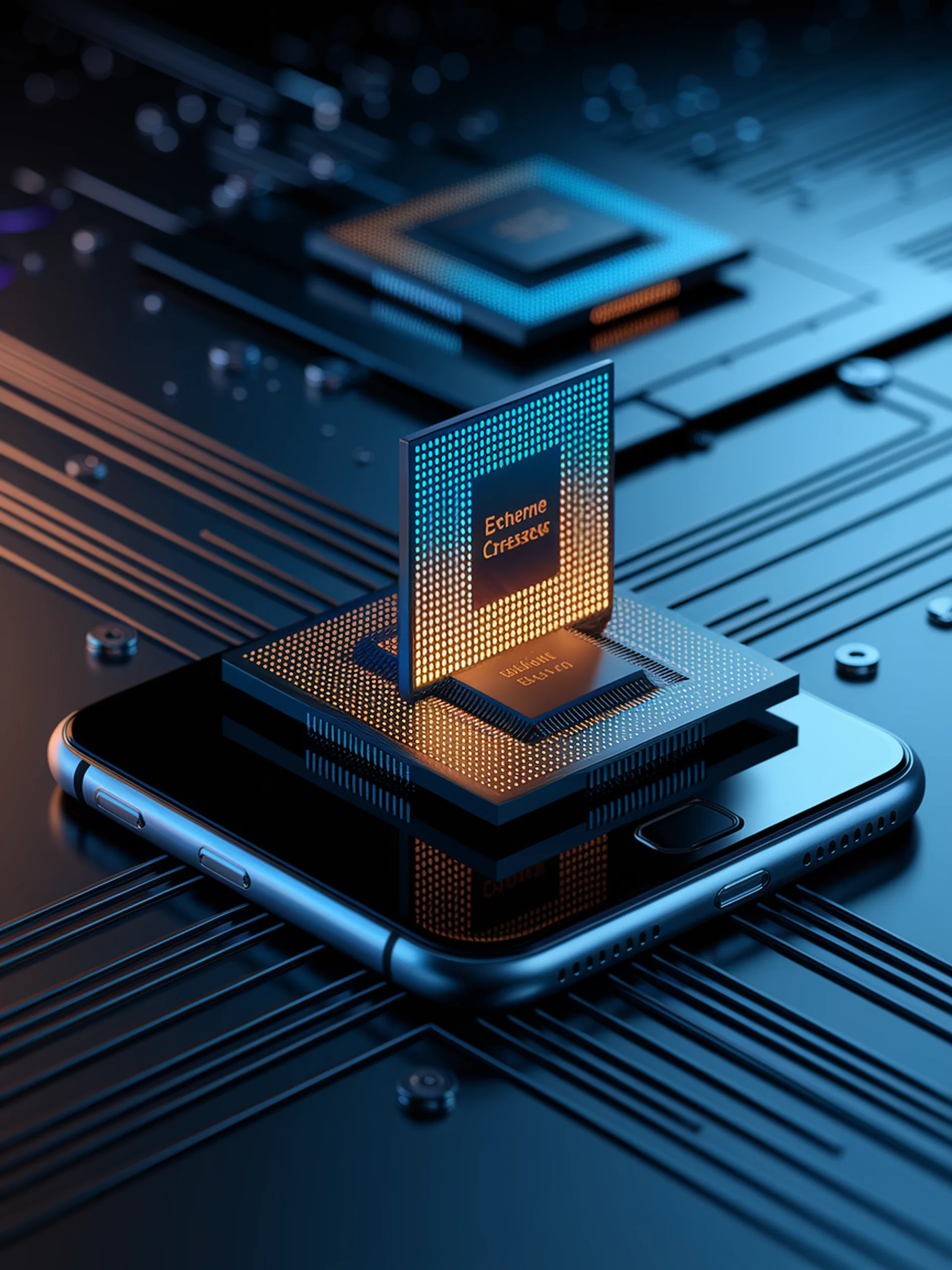
Boosting Ultra-Low-Bit LLMs
A breakthrough in 2-bit model performance with RILQ
RILQ (Rank-Insensitive LoRA-based Quantization) overcomes fundamental limitations in compressed large language models, enabling high-accuracy 2-bit LLMs for resource-constrained environments.
- Identifies and addresses key limitations of existing LoRA-based quantization error compensation
- Introduces rank-insensitivity to significantly improve performance in 2-bit quantized models
- Delivers substantial accuracy gains while maintaining minimal memory footprint
- Enables practical deployment of highly compressed LLMs on edge devices and limited hardware
This engineering advancement represents a critical step toward democratizing AI by allowing powerful language models to run efficiently on diverse hardware, from mobile devices to IoT applications.