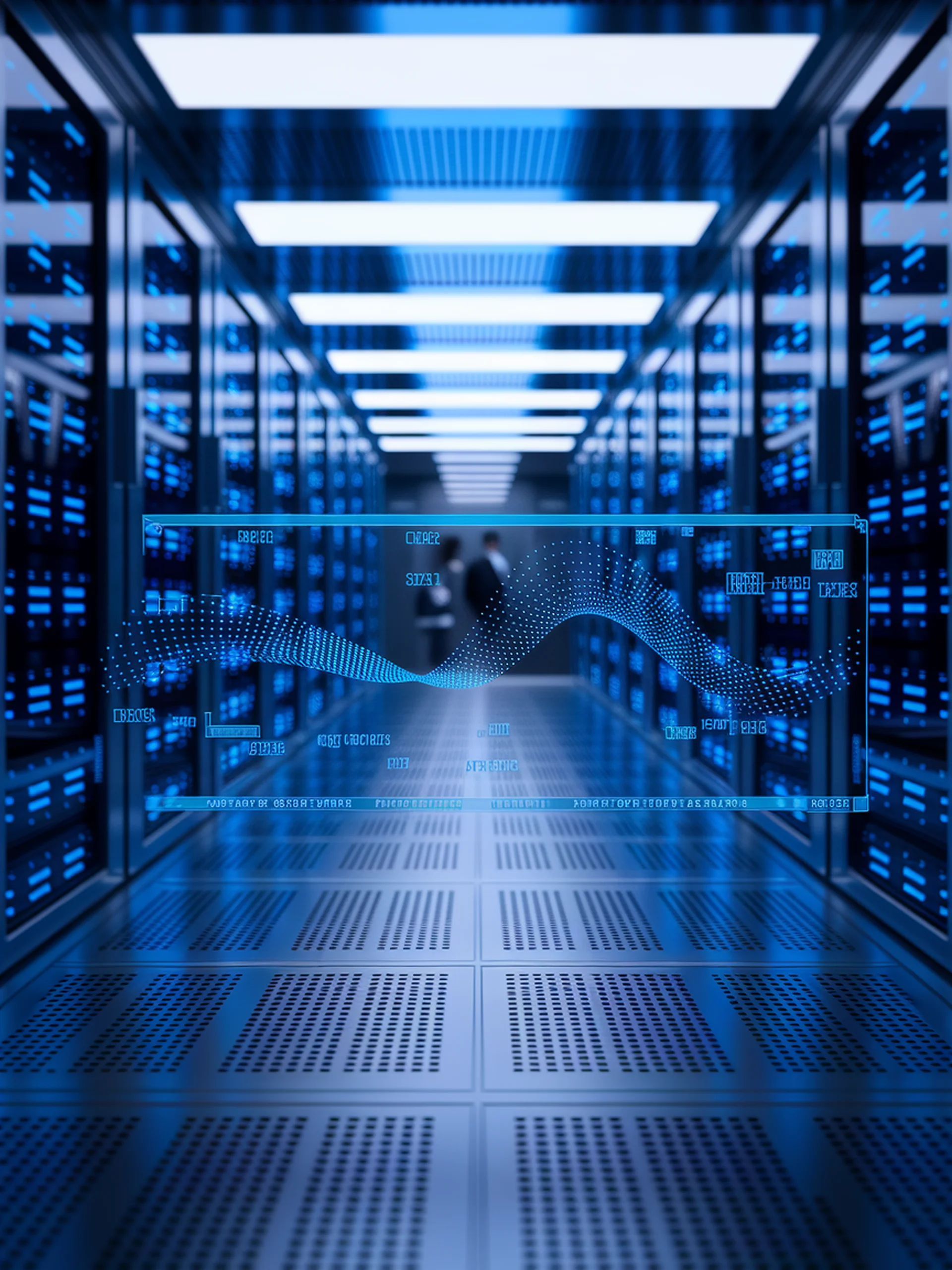
Smarter KV Cache Compression for LLMs
Leveraging Temporal Attention Patterns for Efficient Inference
AttentionPredictor introduces a novel approach to KV cache compression that considers temporal patterns in attention scores for more efficient LLM inference, particularly during long-context generation.
- Improves efficiency by predicting future attention patterns rather than relying solely on past attention scores
- Achieves up to 4× compression rate with minimal performance degradation
- Uses a lightweight MLP-based predictor that analyzes historical attention patterns
- Enables faster inference while maintaining output quality for long-context applications
This engineering advancement addresses a critical bottleneck in LLM deployment by reducing memory requirements and computational costs without sacrificing performance quality—essential for scaling AI systems in resource-constrained environments.
AttentionPredictor: Temporal Pattern Matters for Efficient LLM Inference