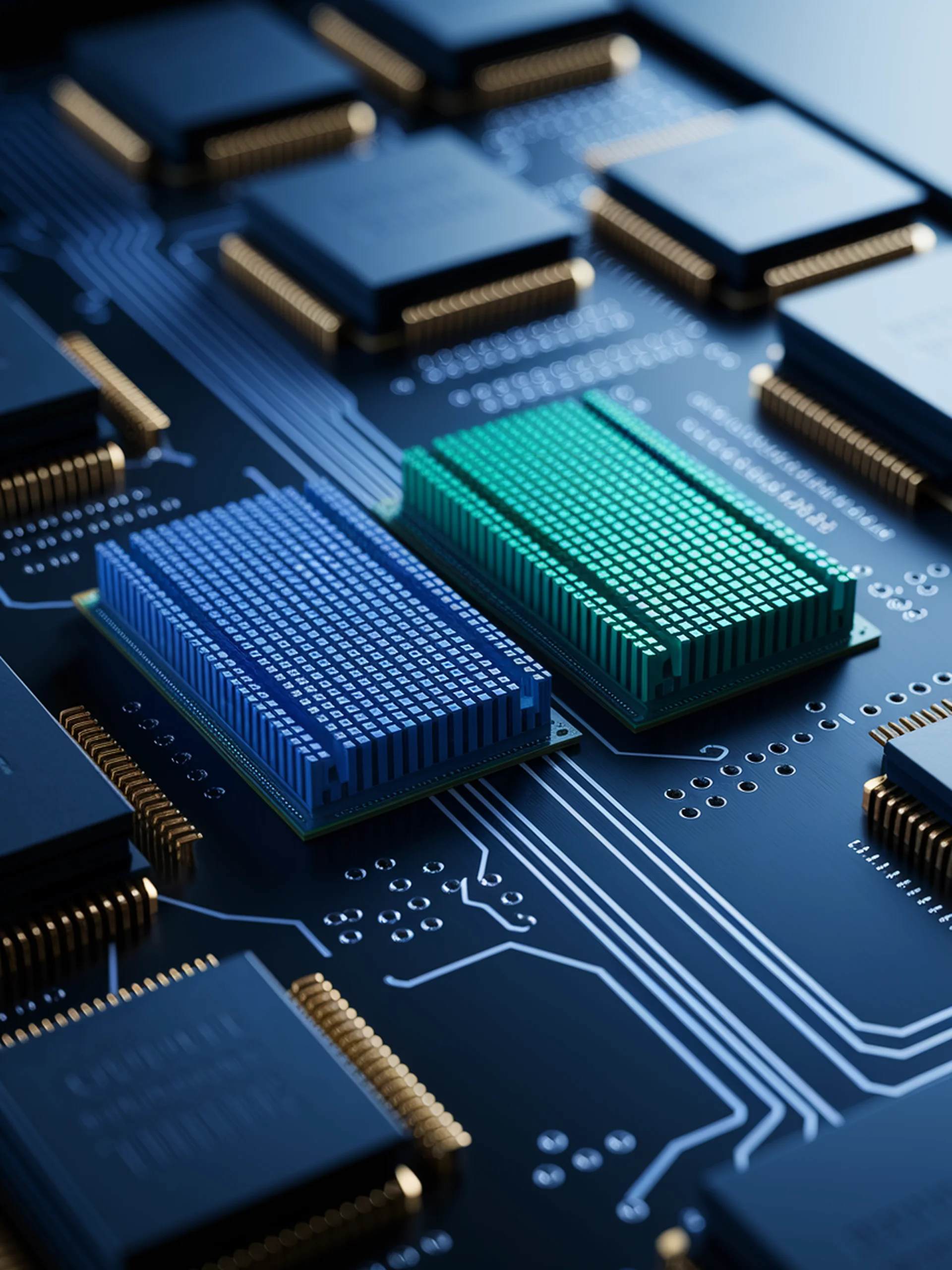
Smart Memory Compression for LLMs
Keys need more bits, values need fewer
This research introduces an adaptive quantization framework that significantly reduces memory requirements for LLMs while preserving performance.
- Key finding: Keys and values should be treated differently - keys need higher precision than values
- Memory reduction: Up to 8x compression of inference memory with minimal performance loss
- Novel approach: Information-aware quantization that adapts to the unique properties of different matrix types
- Practical implementation: Compatible with existing LLM architectures and deployment scenarios
This engineering breakthrough enables more efficient LLM deployment on resource-constrained devices and reduces operational costs for AI infrastructure.
More for Keys, Less for Values: Adaptive KV Cache Quantization