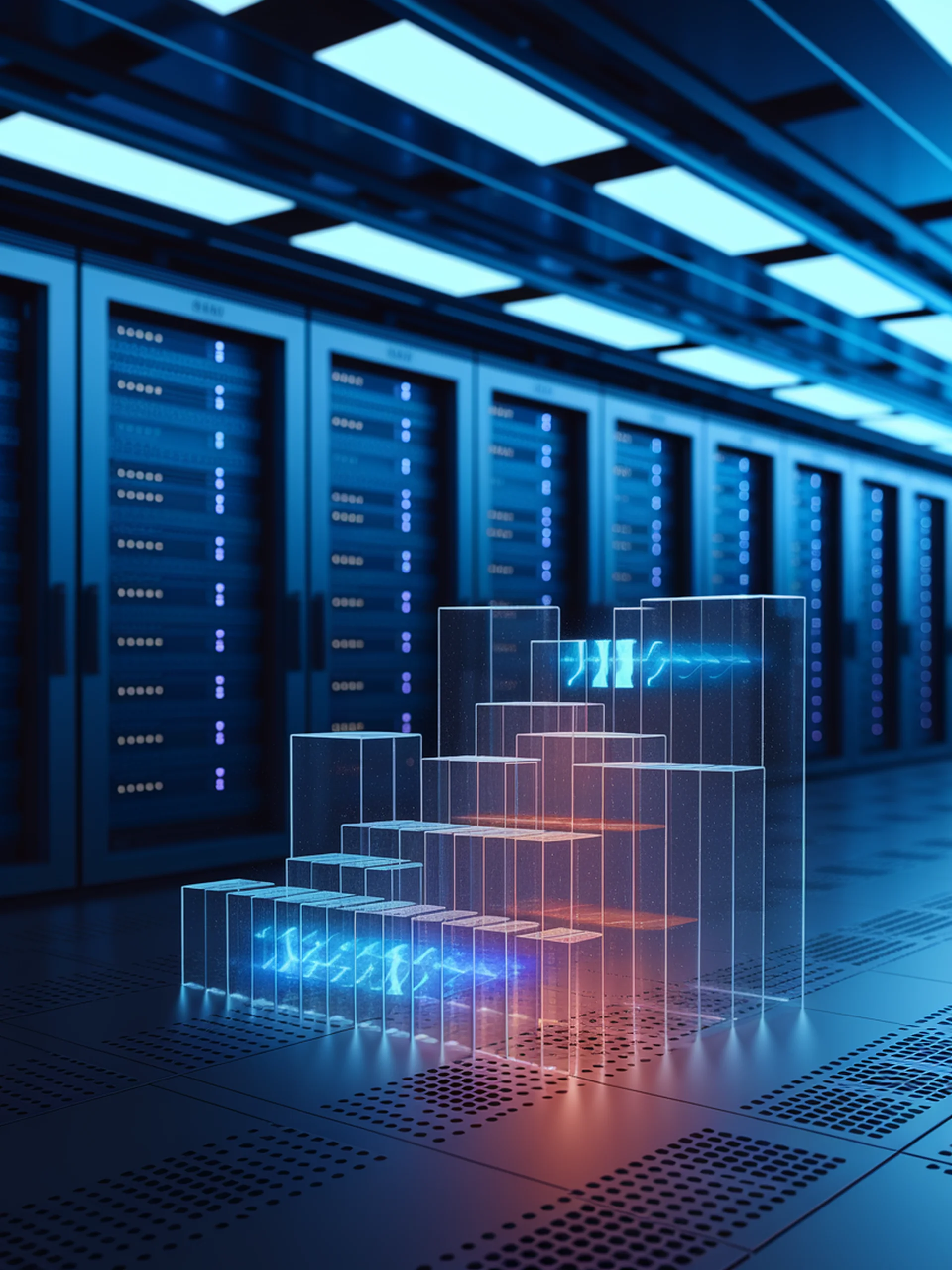
Smarter Memory for Multimodal AI
Dynamic optimization for long-context AI processing
MEDA introduces an intelligent approach for managing memory in multimodal large language models, dramatically improving efficiency for processing long text, images, and videos.
- Adapts memory allocation based on attention patterns across neural network layers
- Achieves 1.75-2.17x speedup for long-context inference without accuracy loss
- Eliminates the need for uniform or progressive reduction strategies common in existing methods
- Enables resource-efficient processing of complex multimodal inputs
This advancement directly addresses critical engineering challenges in deploying multimodal AI systems at scale, where memory constraints often limit practical applications in real-world scenarios.
MEDA: Dynamic KV Cache Allocation for Efficient Multimodal Long-Context Inference