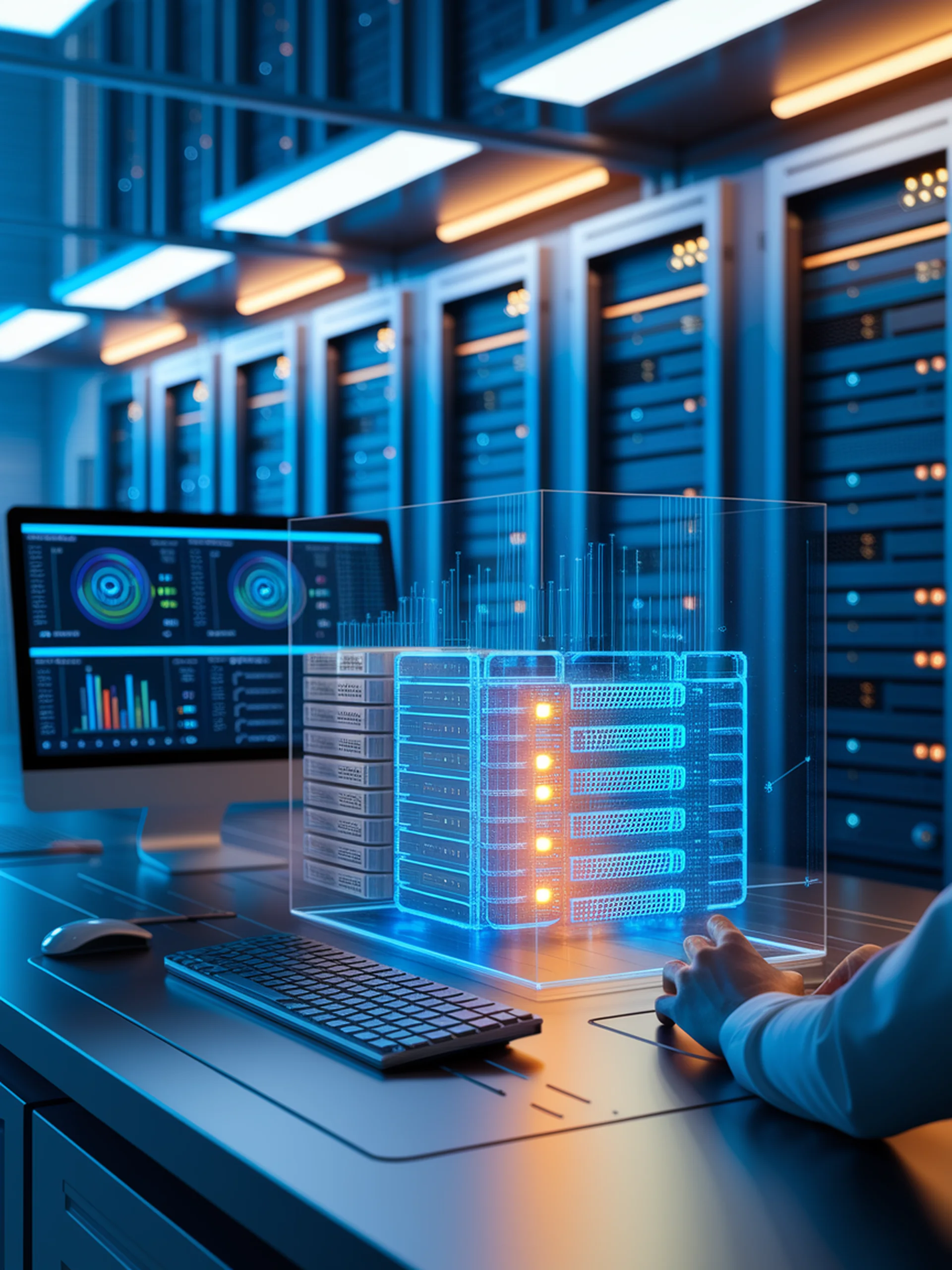
Optimizing AI Training with Virtual Accelerators
Reducing costs and improving efficiency through emulation
Maya introduces a novel approach to optimize deep learning training workloads through emulated virtual accelerators, dramatically reducing the costs of model deployment.
- Creates accurate performance predictions without requiring users to translate workloads into custom languages
- Enables efficient exploration of training configurations without expensive hardware
- Helps ML engineers avoid error-prone manual optimization on costly compute clusters
- Directly addresses the hundreds of millions of dollars spent on training large foundation models
This engineering breakthrough allows organizations to systematically optimize their AI deployment strategies before committing to expensive hardware resources, significantly reducing both costs and time-to-market.
Maya: Optimizing Deep Learning Training Workloads using Emulated Virtual Accelerators