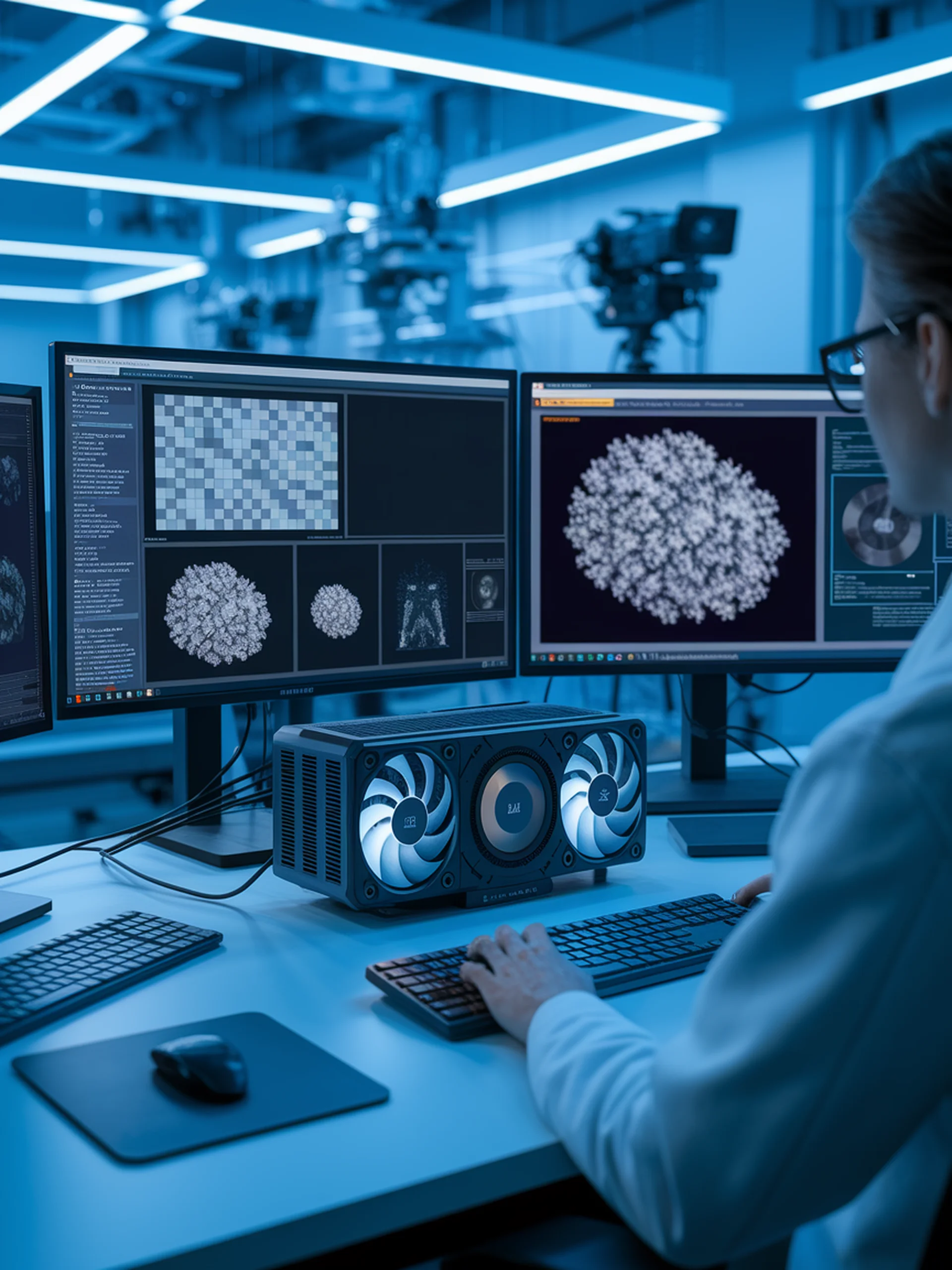
Supercharging Image Quality with Low-Rank Adaptation
Efficient CNN enhancement without expanding model architecture
DSCLoRA applies low-rank adaptation techniques from large language models to image super-resolution, boosting performance without increasing computational complexity.
- Introduces convolutional low-rank adaptation specifically designed for CNN-based image processing
- Employs knowledge distillation to effectively transfer learning from larger models
- Achieves superior image quality while maintaining lightweight model architecture
- Demonstrates 5.43% PSNR improvement with minimal parameter increase (only 5.77%)
For engineering teams, this research offers a practical approach to enhance image processing applications without the computational costs typically associated with performance improvements.
Distillation-Supervised Convolutional Low-Rank Adaptation for Efficient Image Super-Resolution