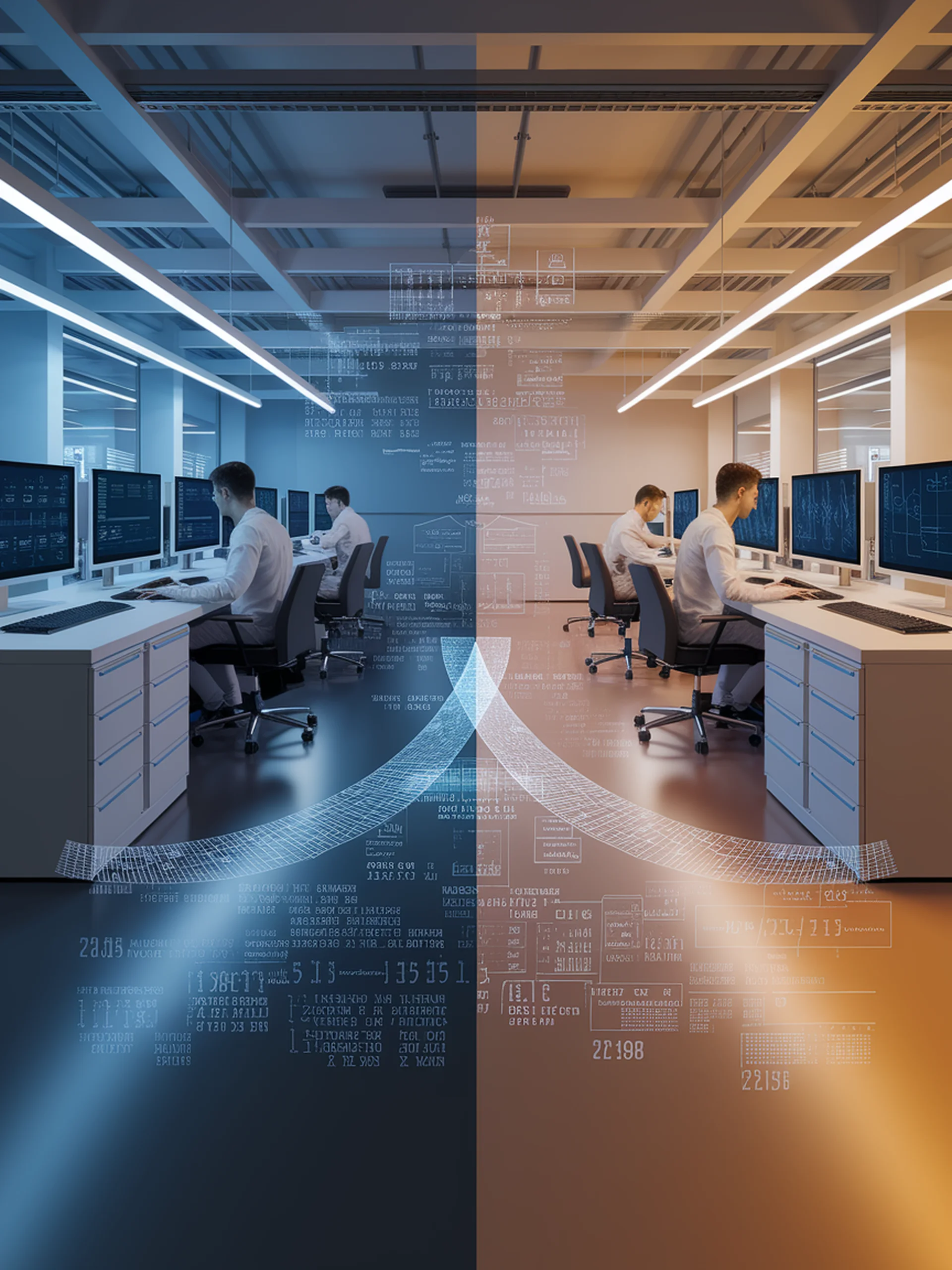
Bridging NLP and Optimization Modeling
A scalable framework for synthetic data that enhances LLM capabilities
OptMATH addresses a critical gap in AI: the shortage of high-quality datasets for training language models to translate natural language into mathematical optimization models.
- Creates a bidirectional data synthesis framework that generates diverse, high-quality training examples
- Enables LLMs to better understand and formulate optimization problems from natural language descriptions
- Significantly improves the ability of AI systems to model complex engineering problems that require mathematical optimization
- Provides a scalable solution to the data scarcity challenge that has limited previous approaches
For engineering teams, this research opens new possibilities for automating the conversion of business requirements into precise mathematical models, potentially accelerating solution development and reducing expertise barriers.
OptMATH: A Scalable Bidirectional Data Synthesis Framework for Optimization Modeling