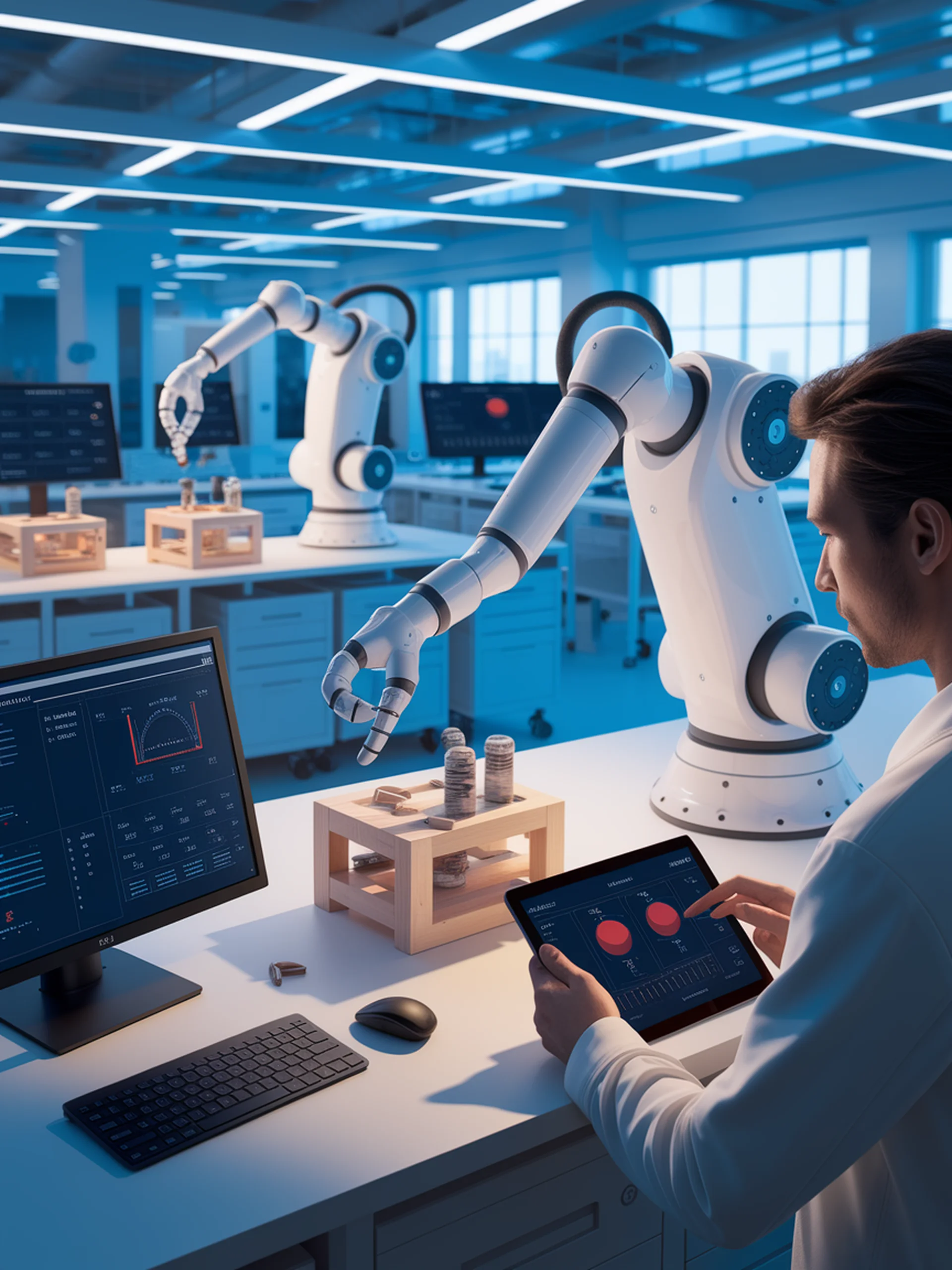
Teaching Robots to Generalize
Improving Robot Policy through Human Preferences
GRAPE introduces a novel framework that enables robots to better generalize their learned behaviors across diverse tasks through preference alignment.
- Combines behavior cloning with preference learning to create more adaptable robot policies
- Leverages a preference-aligned reward model that integrates human feedback
- Demonstrates superior performance on unseen tasks compared to traditional approaches
- Achieves better safety and efficiency in manipulation tasks
This research advances robotic engineering by addressing the critical challenge of generalizability, enabling robots to perform effectively in manufacturing environments even when faced with novel situations or objectives.