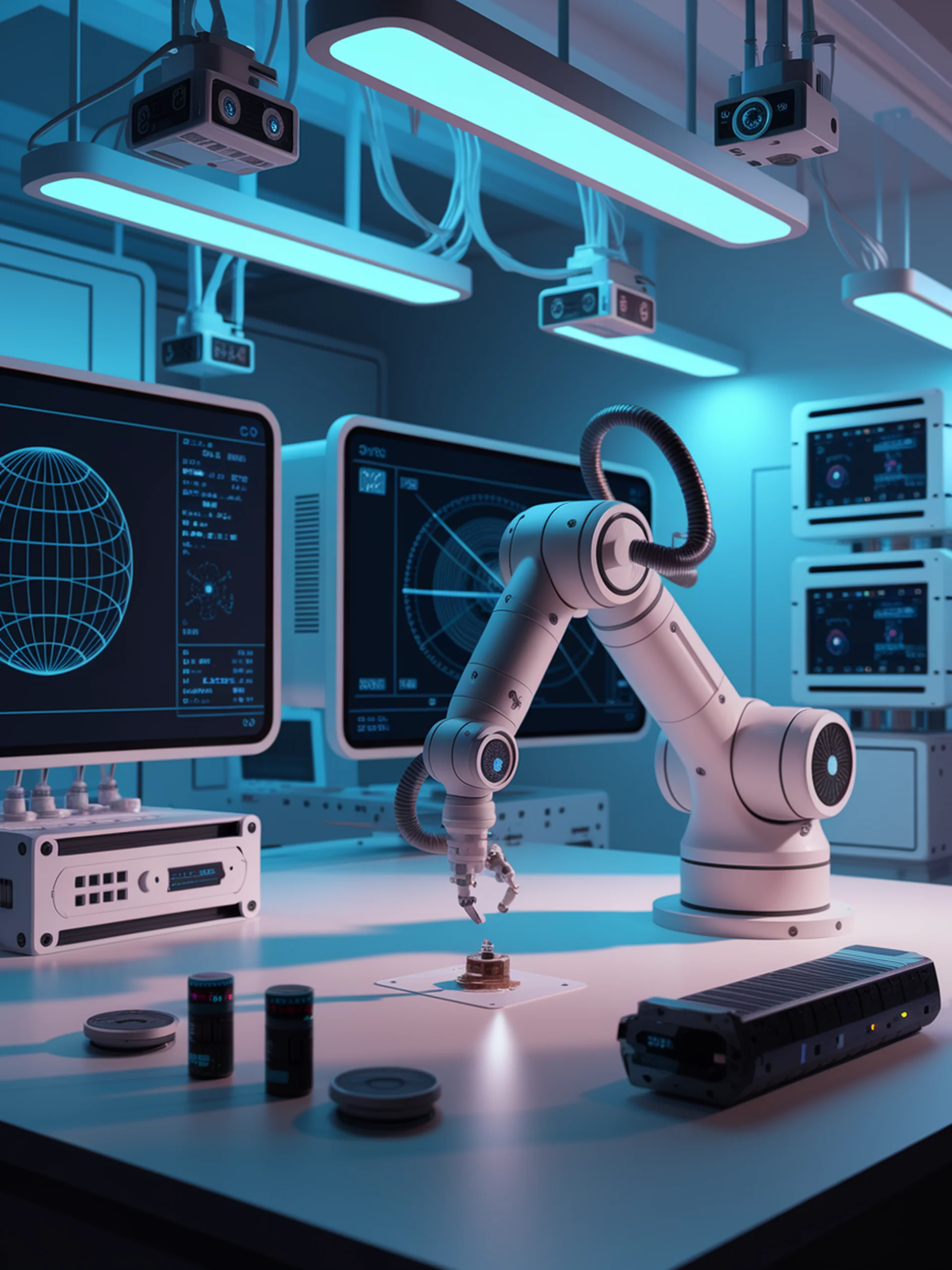
Bridging the Gap in Robot Learning
Foundation Models for Physical Agency Through Procedural Generation
This research creates foundation models for robots that simultaneously handle physical contact dynamics and environmental semantics, advancing robot capabilities for real-world applications.
- Combines generative modeling, photorealistic rendering, and procedural generation to create robots that understand both contact dynamics and environment context
- Generates contact-rich trajectories with accurate physics while maintaining awareness of environmental semantics
- Creates a pathway for robots to develop physical agency in complex environments
- Demonstrates how simulation environments can be leveraged to train more capable robotic systems
This breakthrough matters for engineering by enabling robots that can navigate and manipulate real-world environments with a unified understanding of both physics and context, potentially transforming industrial automation, service robotics, and human-robot interaction.
Proc4Gem: Foundation models for physical agency through procedural generation