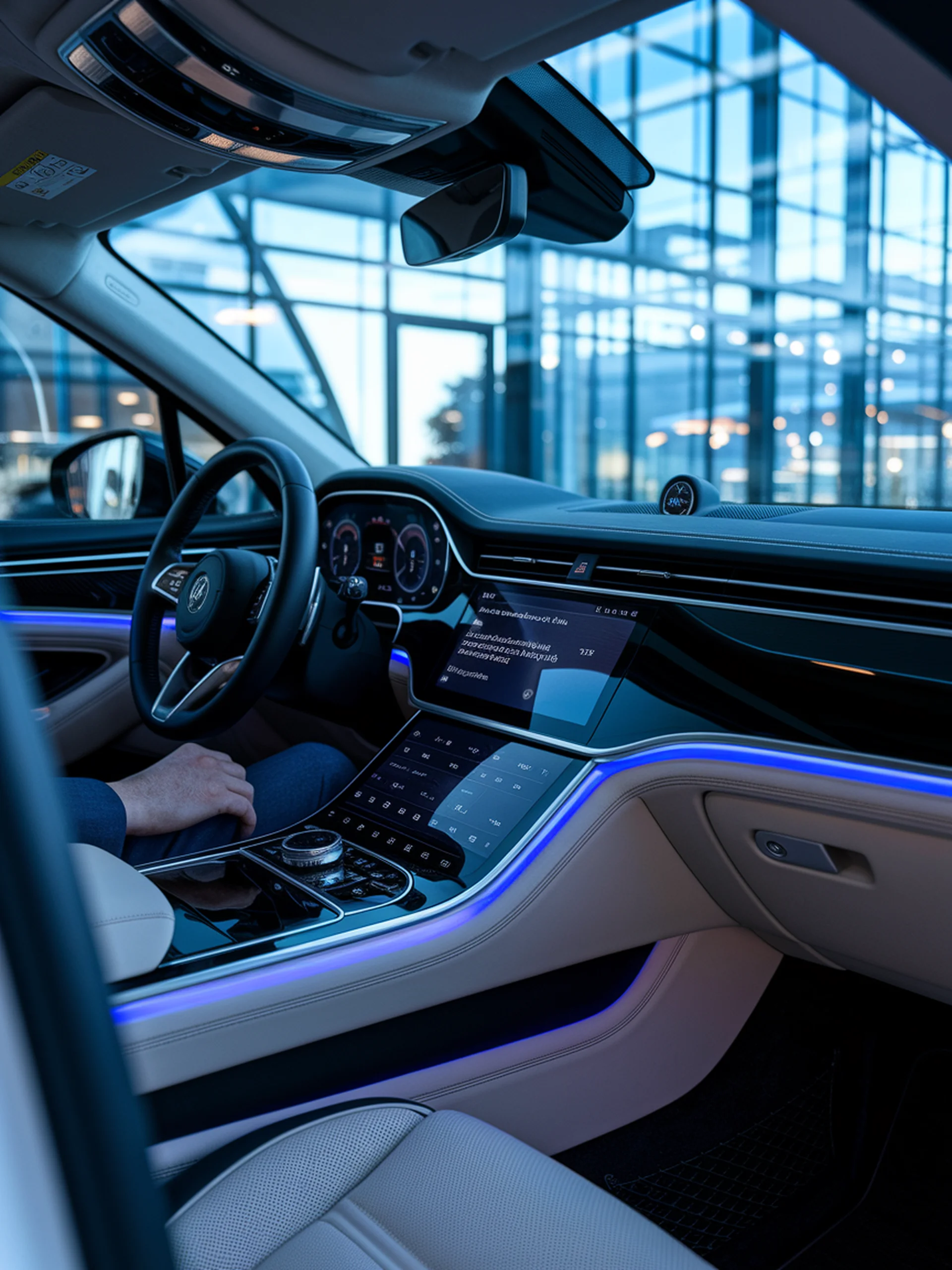
Improving In-Car AI Conversations
Automated Benchmarking to Reduce AI Hallucinations
This research introduces a novel LLM-based methodology for automatically evaluating the factual accuracy of in-vehicle conversational systems.
- Addresses the critical problem of AI hallucinations in automotive interfaces
- Employs five LLM-based methods and ensemble techniques to benchmark factual correctness
- Creates a systematic way to evaluate and improve in-car AI assistants
- Helps engineering teams develop more reliable conversational systems for vehicles
This approach enables automotive engineers to identify and address factual errors before deployment, significantly improving user trust and safety in conversational automotive interfaces.
Automated Factual Benchmarking for In-Car Conversational Systems using Large Language Models