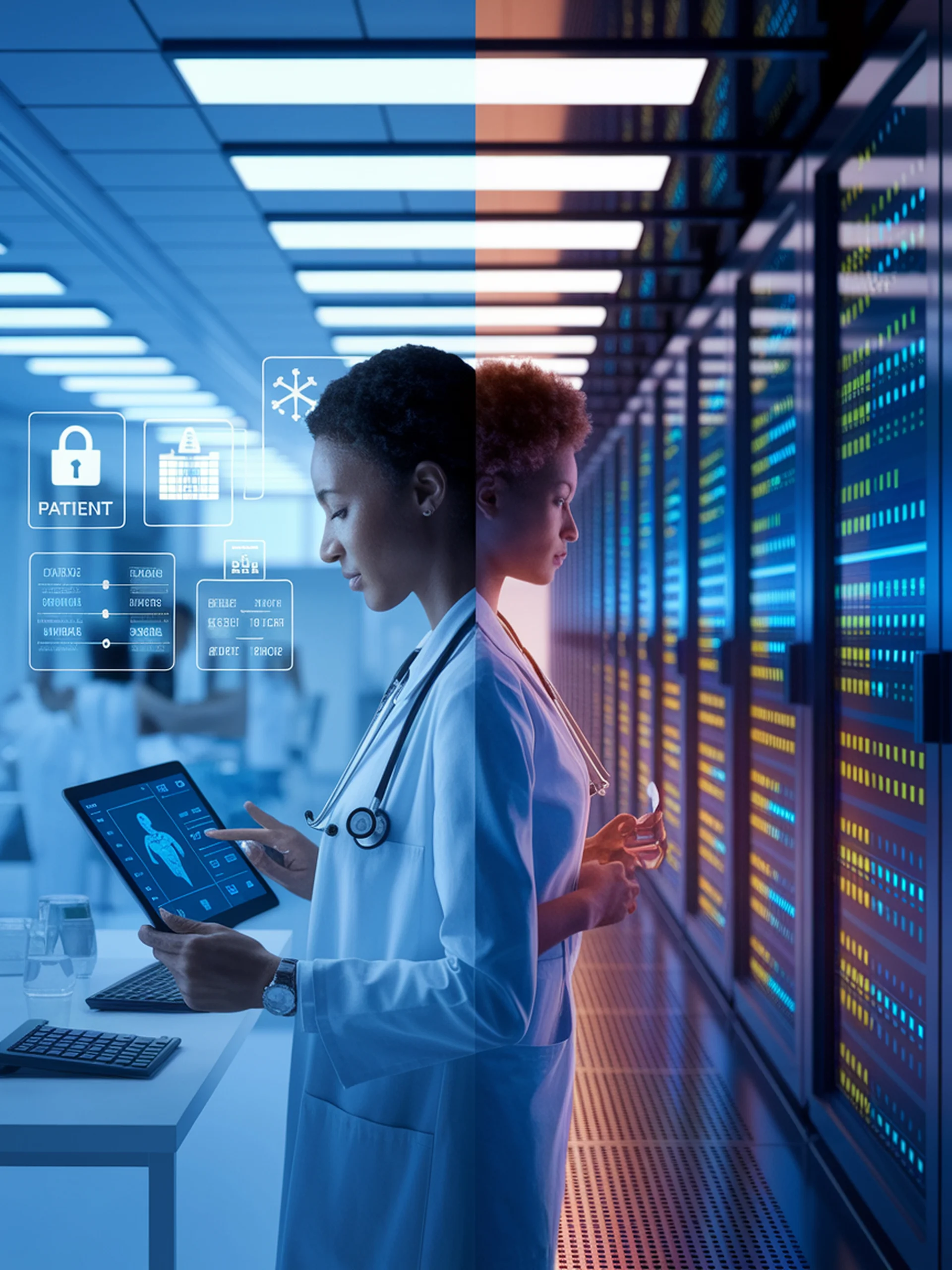
Ethical Concerns in Healthcare AI
Balancing innovation with patient welfare and trust
Patient Privacy and Data Security
- Protecting patient privacy while utilizing data for AI development is a delicate balance
- Concerns include potential data breaches and unauthorized data access
- Data solidarity concept suggests patients may support data use for common good if done transparently
- European initiatives like GAIA-X cloud aim to create trusted environments for health AI development
Bias and Fairness
- AI systems can perpetuate or amplify biases present in training data
- A biased AI could lead to health disparities across demographic groups
- EU projects emphasize obtaining diverse, representative datasets for AI development
- The EU AI Act will require transparency about training data and bias mitigation strategies
Transparency and Informed Consent
- Patients have the right to know when algorithms are involved in their care
- EU guidelines advise informing patients when AI assists in diagnosis or treatment
- Informed consent processes are being updated to cover AI tools
- The principle of human oversight ensures AI doesn't make autonomous decisions
Liability and Accountability
- Unclear responsibility if AI causes errors creates accountability challenges
- Currently, the accountable person is usually the physician using the AI's advice
- The teamwork model promotes treating AI as a tool under clinical supervision
- Hospitals often have guidelines stating clinicians must validate AI outputs
Ethical Use of LLMs
- LLMs can produce incorrect but authoritative-sounding answers (hallucinations)
- Many hospitals establish ethics committees to evaluate LLM applications
- There's concern about balancing AI efficiency with maintaining human empathy
- European approach emphasizes AI augmenting rather than replacing human care