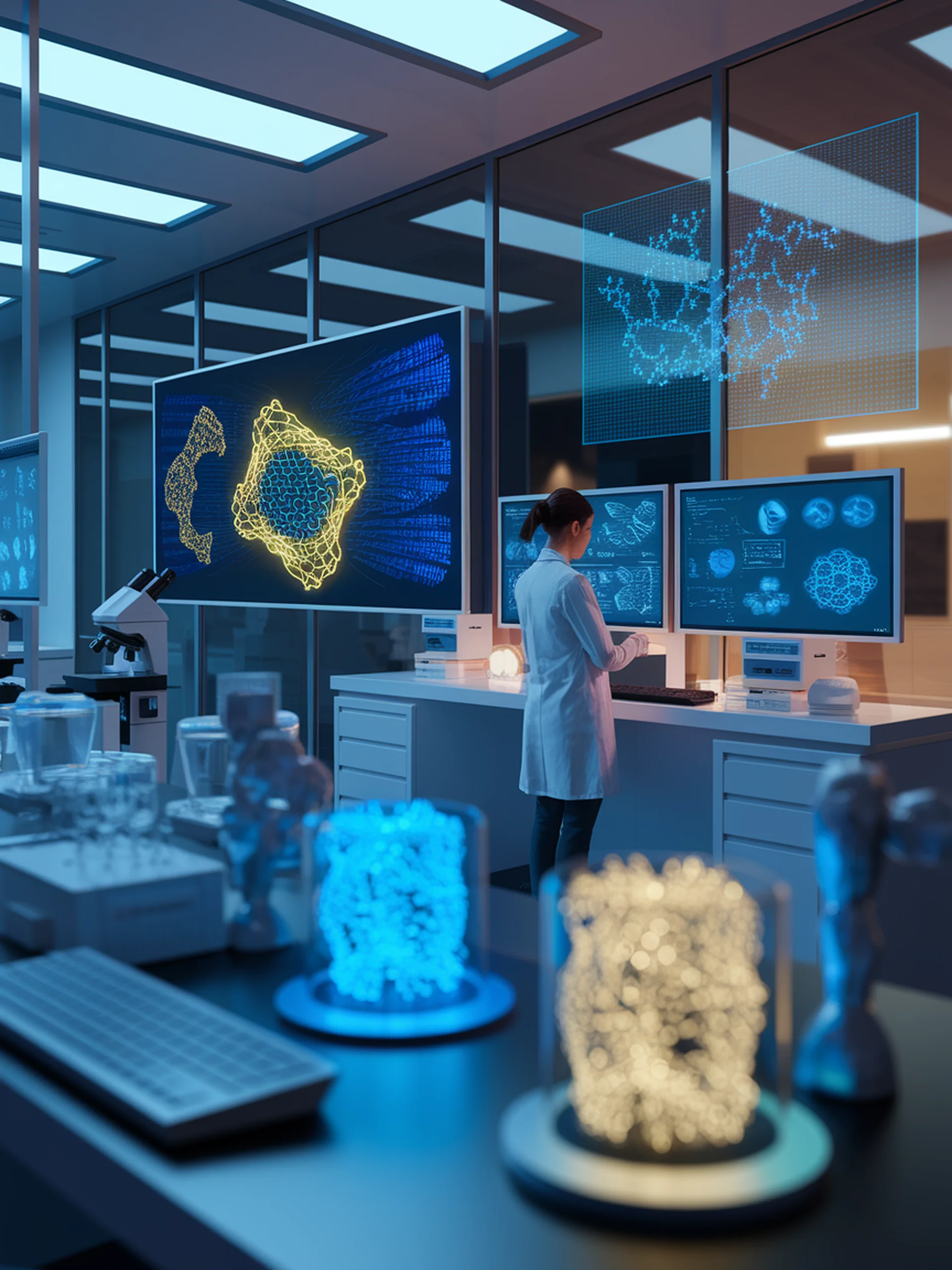
Unlocking Multi-Modal Protein Intelligence
Advancing biology with bidirectional hierarchical learning across protein sequences and structures
This research introduces a novel bidirectional hierarchical framework that integrates both protein sequence and structural information for enhanced biological predictions.
- Combines strengths of protein language models (pLMs) with graph neural networks (GNNs) to capture complete protein characteristics
- Implements innovative hierarchical fusion to bridge sequence and structural representations
- Demonstrates superior performance across enzyme classification, protein-ligand binding, and protein-protein interaction tasks
- Establishes a new state-of-the-art approach for multi-modal protein representation
Why It Matters: This breakthrough enables more accurate protein function prediction critical for drug discovery, understanding disease mechanisms, and advancing precision medicine applications.
Original Paper: Bidirectional Hierarchical Protein Multi-Modal Representation Learning