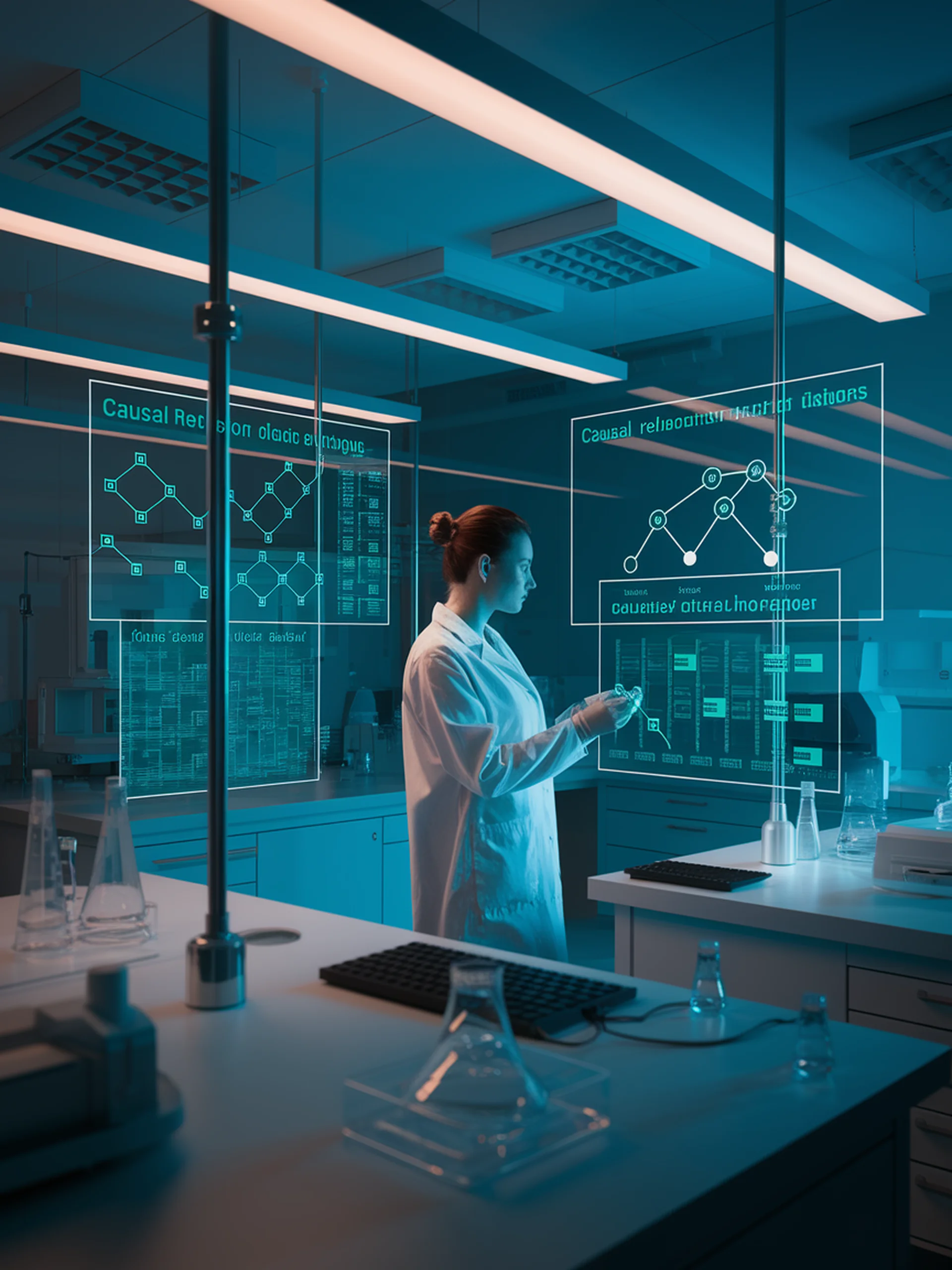
Rethinking LLM Causal Inference
Why Causal Order Outperforms Direct Graph Construction
This research introduces a more effective approach for using Large Language Models as causal inference experts by focusing on causal order rather than direct graph construction.
- LLMs struggle to distinguish between direct and indirect causal effects when asked about pairwise relationships
- Using causal order as the output interface produces more reliable results than graph-based approaches
- The proposed method handles imperfect expert knowledge more gracefully, reducing error propagation
- Novel engineering approach improves stability and accuracy in causal inference tasks
This advancement matters for medical research and engineering applications by providing more reliable causal inference tools, allowing for better understanding of complex relationships in data without requiring perfect expert knowledge.
Causal Order: The Key to Leveraging Imperfect Experts in Causal Inference