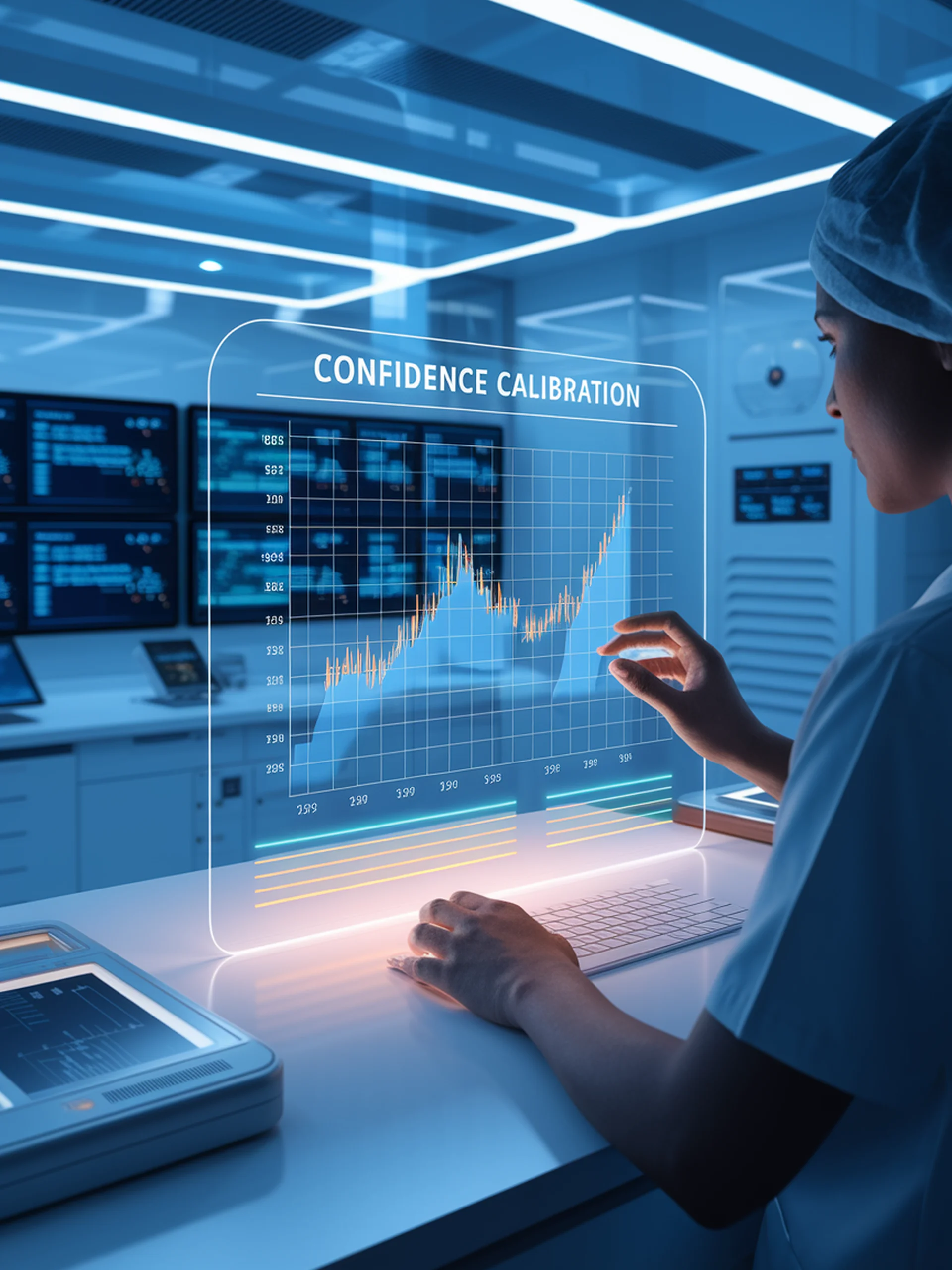
Improved Confidence in AI Decision-Making
New calibration techniques for language model reliability in security applications
This research introduces a novel approach to calibrate confidence scores in generative question-answering systems, making AI decisions more reliable and interpretable for critical applications.
- Moves beyond average-case calibration to provide more meaningful confidence scoring
- Develops specialized calibration techniques for security-critical contexts
- Enables more trustworthy AI deployments where incorrect decisions could have serious consequences
- Particularly valuable for security applications requiring reliable decision-making under uncertainty
For security professionals, this research offers a pathway to deploy AI systems with better safeguards against overconfident but incorrect answers, reducing risks in sensitive environments.