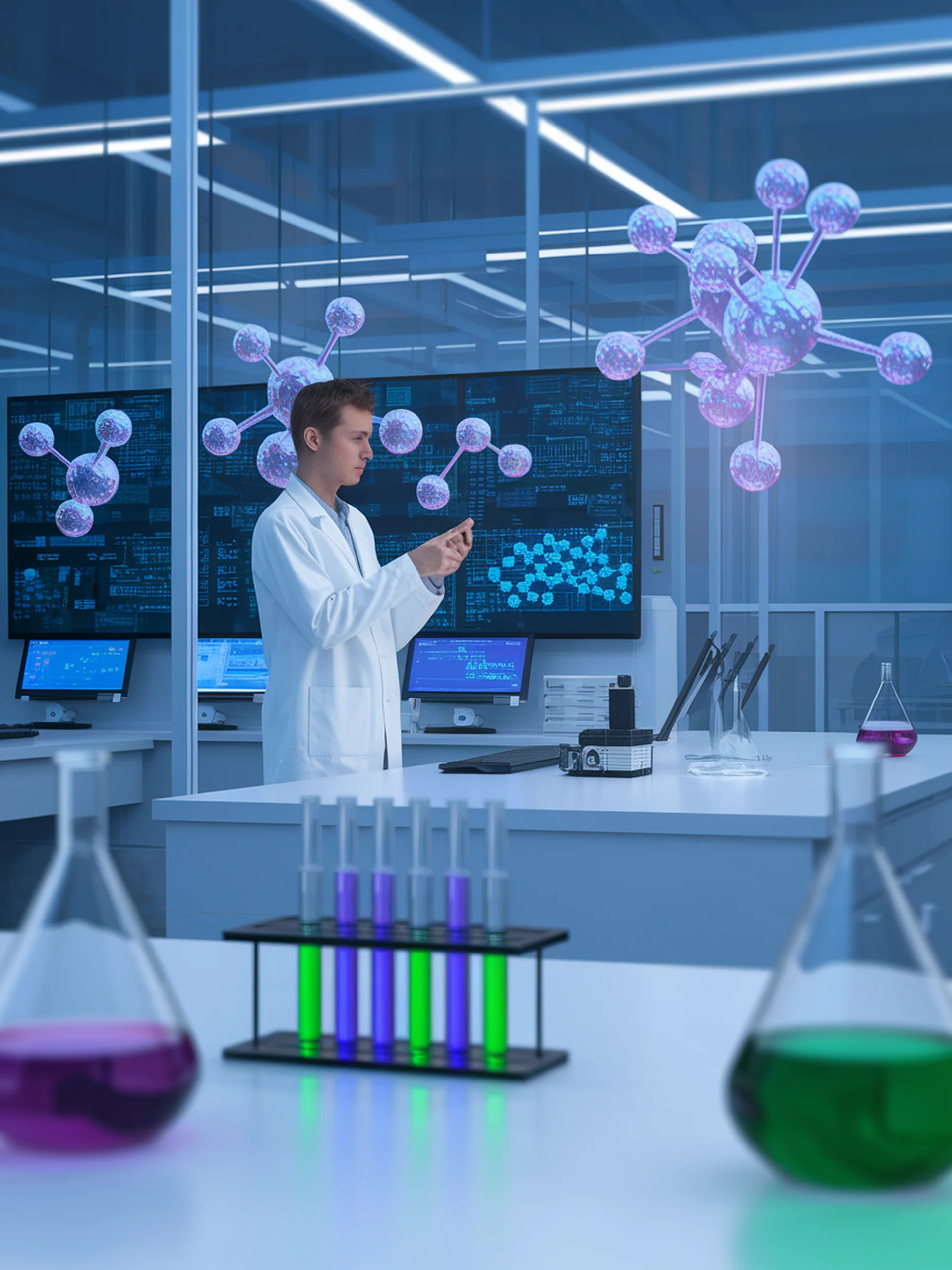
Smarter Drug Discovery with LLMs
Enhancing AI-driven molecule generation through entropy-reinforced planning
This research introduces a novel approach that combines large language models with entropy-reinforced planning to generate valid and effective drug molecules.
- Addresses key limitations in current LLM-based drug discovery by balancing exploration and exploitation
- Improves success rates in generating pharmaceutically viable compounds
- Outperforms existing methods when tested on targets including SARS-CoV-2 and cancer cell proteins
- Provides a framework that reduces the generation of invalid molecules due to token errors
This advancement matters for medical research by accelerating the drug discovery pipeline, potentially reducing development time and costs for new therapeutics targeting critical diseases.
Entropy-Reinforced Planning with Large Language Models for Drug Discovery