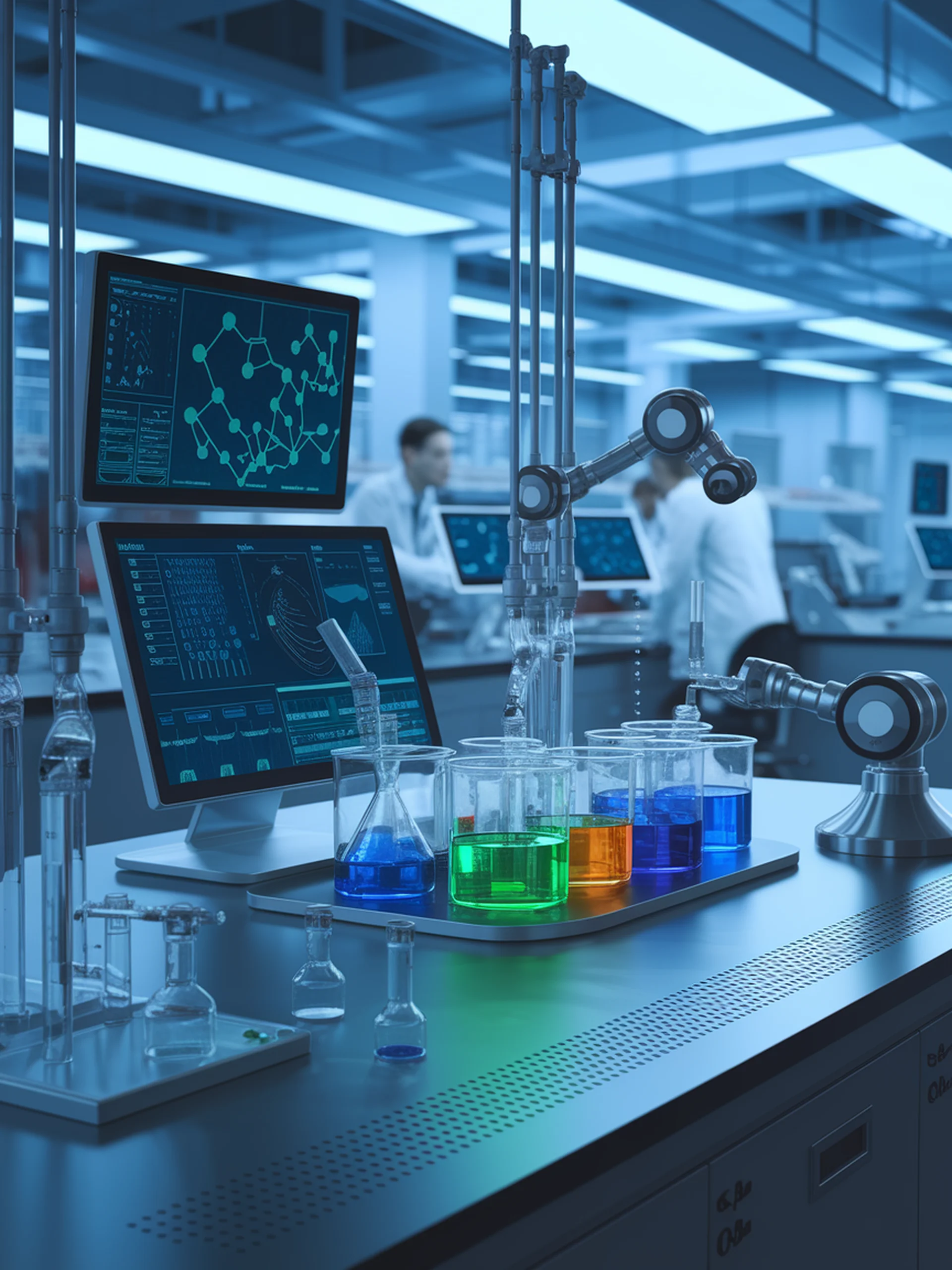
Enhancing Chemical Reaction Optimization with LLMs
Leveraging Language Models to Improve Bayesian Optimization in Low-Data Settings
This research demonstrates how Large Language Models (LLMs) can extract and utilize quantitative chemical knowledge to accelerate Bayesian optimization of chemical reactions, especially when limited experimental data is available.
- Combines LLM capabilities with traditional Bayesian optimization techniques
- Enables more efficient transfer learning from pre-existing chemical information
- Significantly improves optimization performance in low-data regimes
- Creates a pathway for LLMs to contribute quantitative value in chemical engineering applications
This innovation matters for Engineering as it provides a practical approach to overcome data limitations in chemical process optimization, potentially reducing experimental costs and accelerating discovery timelines for new materials and reactions.