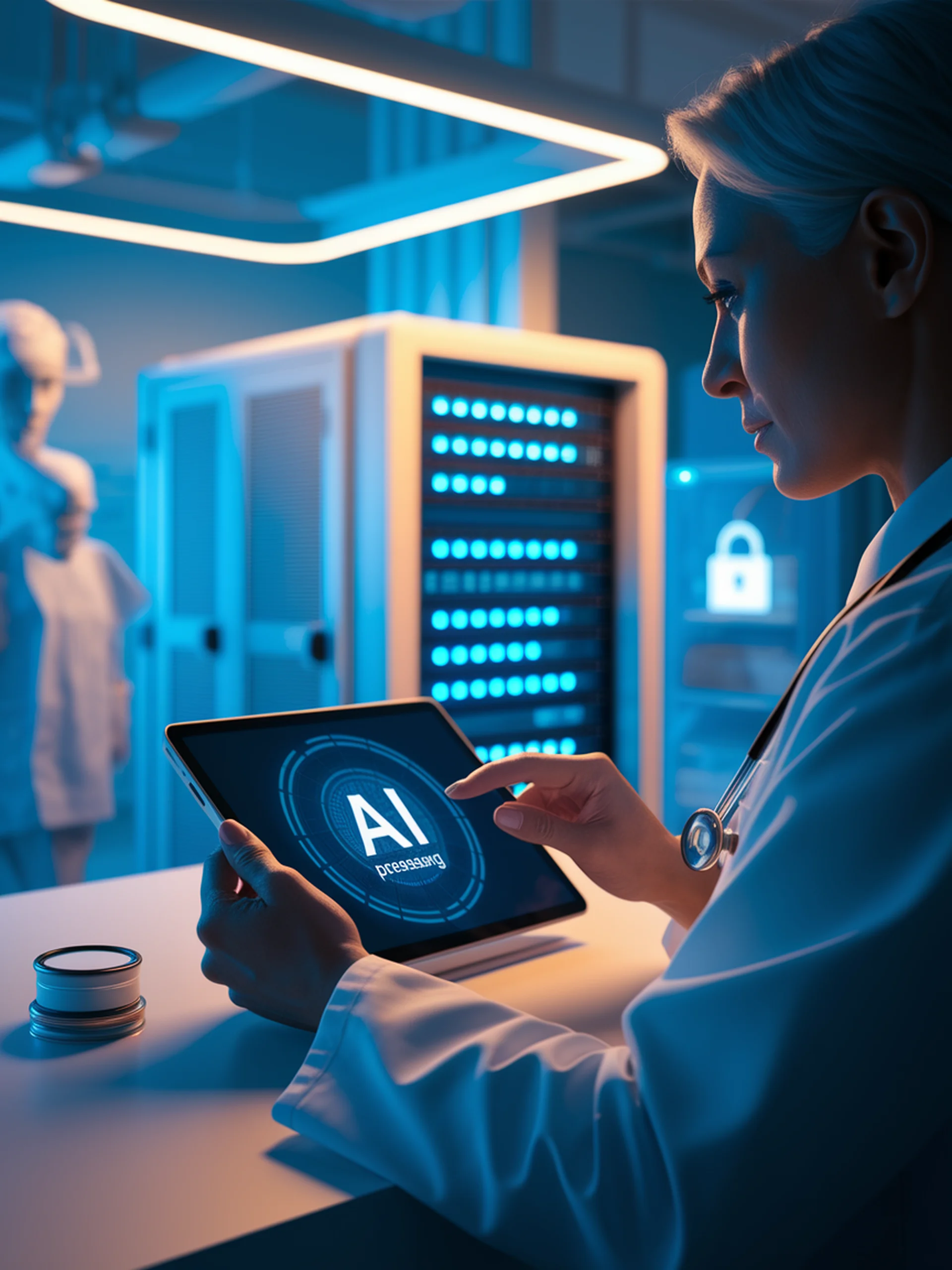
On-Device LLMs for Medical Privacy
Evaluating edge computing models for clinical reasoning without cloud dependence
This research evaluates the performance of on-device Large Language Models for clinical applications, offering solutions that prioritize data privacy and security.
- Benchmarks publicly available edge LLMs using the AMEGA clinical dataset
- Assesses both accuracy and computational efficiency in medical contexts
- Compares performance of medically fine-tuned models (Med42, Aloe) for on-device use
- Highlights privacy benefits of keeping sensitive health data local
This work matters for healthcare organizations seeking to leverage AI capabilities while maintaining strict patient privacy standards and reducing cloud service dependencies.
Medicine on the Edge: Comparative Performance Analysis of On-Device LLMs for Clinical Reasoning