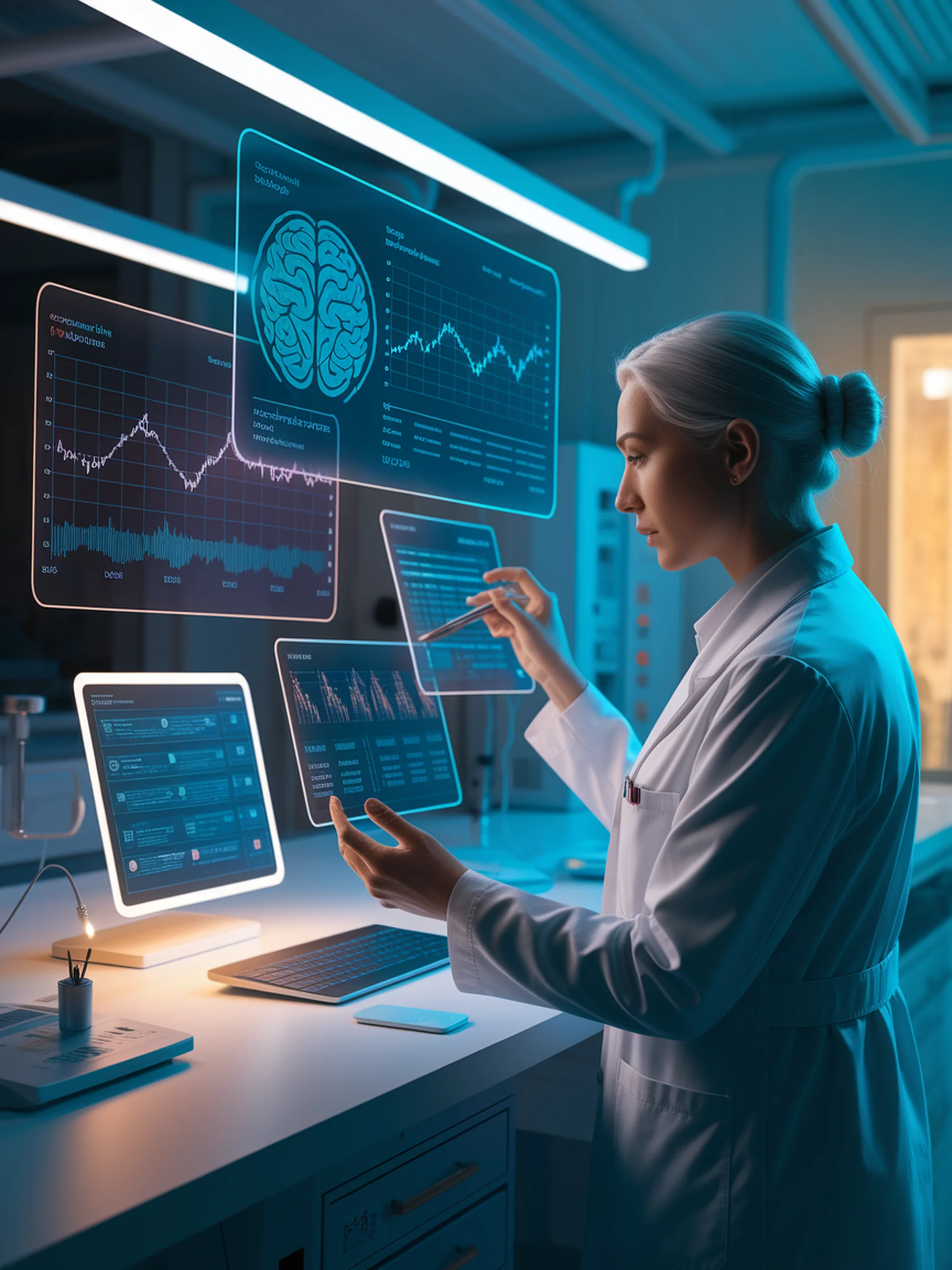
Multi-Dimensional Uncertainty in LLMs
Beyond Semantic Similarity for More Reliable AI Systems
This research proposes a novel approach to uncertainty quantification (UQ) in large language models by examining multiple knowledge dimensions within responses.
- Introduces a multi-dimensional framework for assessing LLM response reliability
- Evaluates uncertainty across factual accuracy, logical coherence, and content relevance dimensions
- Provides more comprehensive reliability metrics than traditional semantic similarity methods
- Demonstrates improved uncertainty detection in high-stakes domains like healthcare
For medical applications, this approach significantly enhances safety by identifying when LLMs may provide unreliable information for clinical decision-making, reducing potential harm from AI-assisted healthcare systems.
Uncertainty Quantification of Large Language Models through Multi-Dimensional Responses