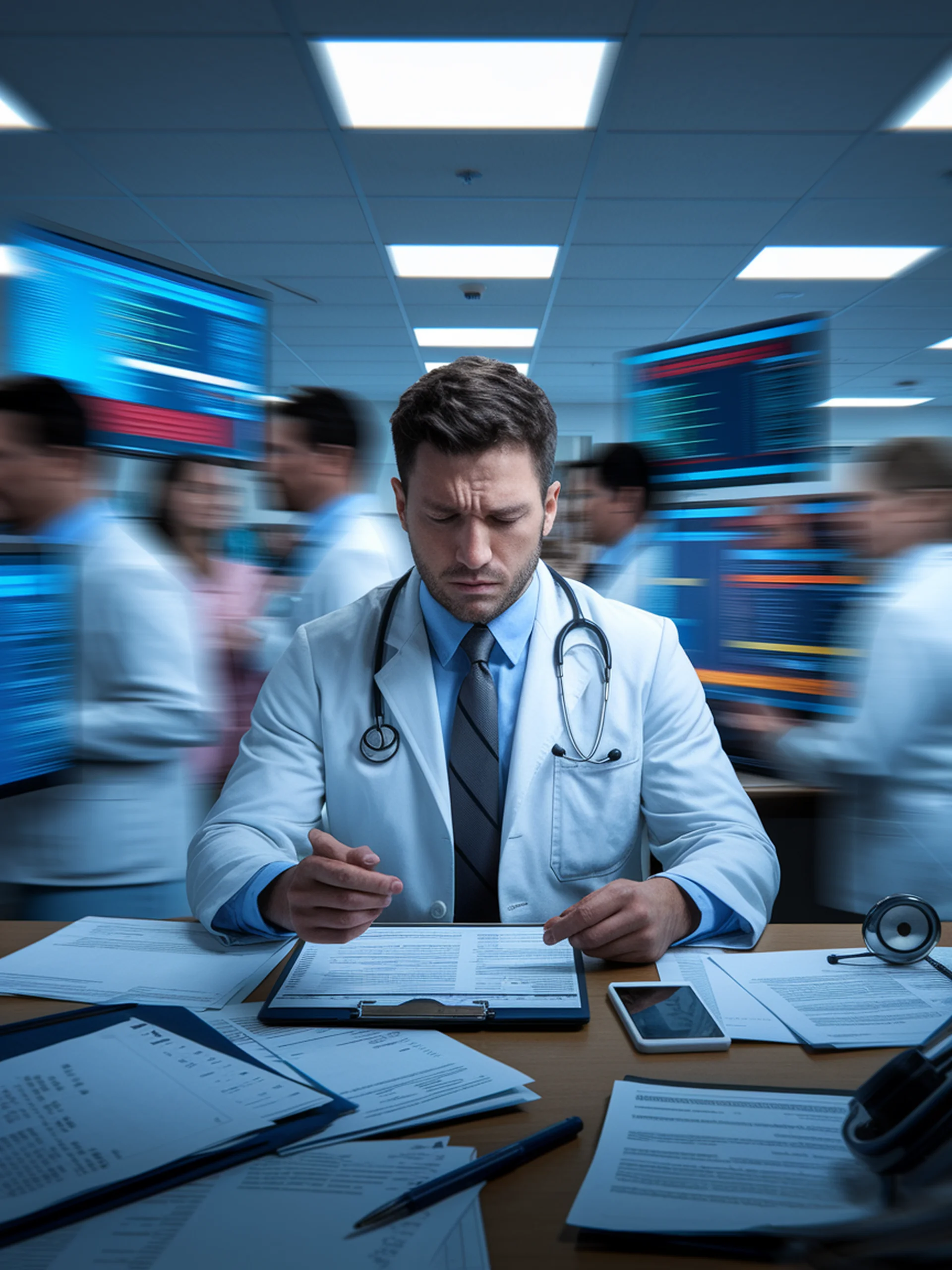
The Distracted Doctor Problem
How noise and irrelevant information impair medical LLMs
This research reveals how extraneous information significantly degrades LLM performance in medical contexts, especially with ambient dictation technologies.
- LLMs show up to 20% performance drop when clinical questions include irrelevant information
- Models struggle to distinguish between relevant clinical data and distractions
- Both open and closed-source models (including fine-tuned medical models) are vulnerable
- Performance deteriorates as the ratio of irrelevant to relevant information increases
Implications for healthcare: As medical environments adopt ambient dictation and automated note generation, these vulnerabilities could impact patient care and safety if not addressed in model design.