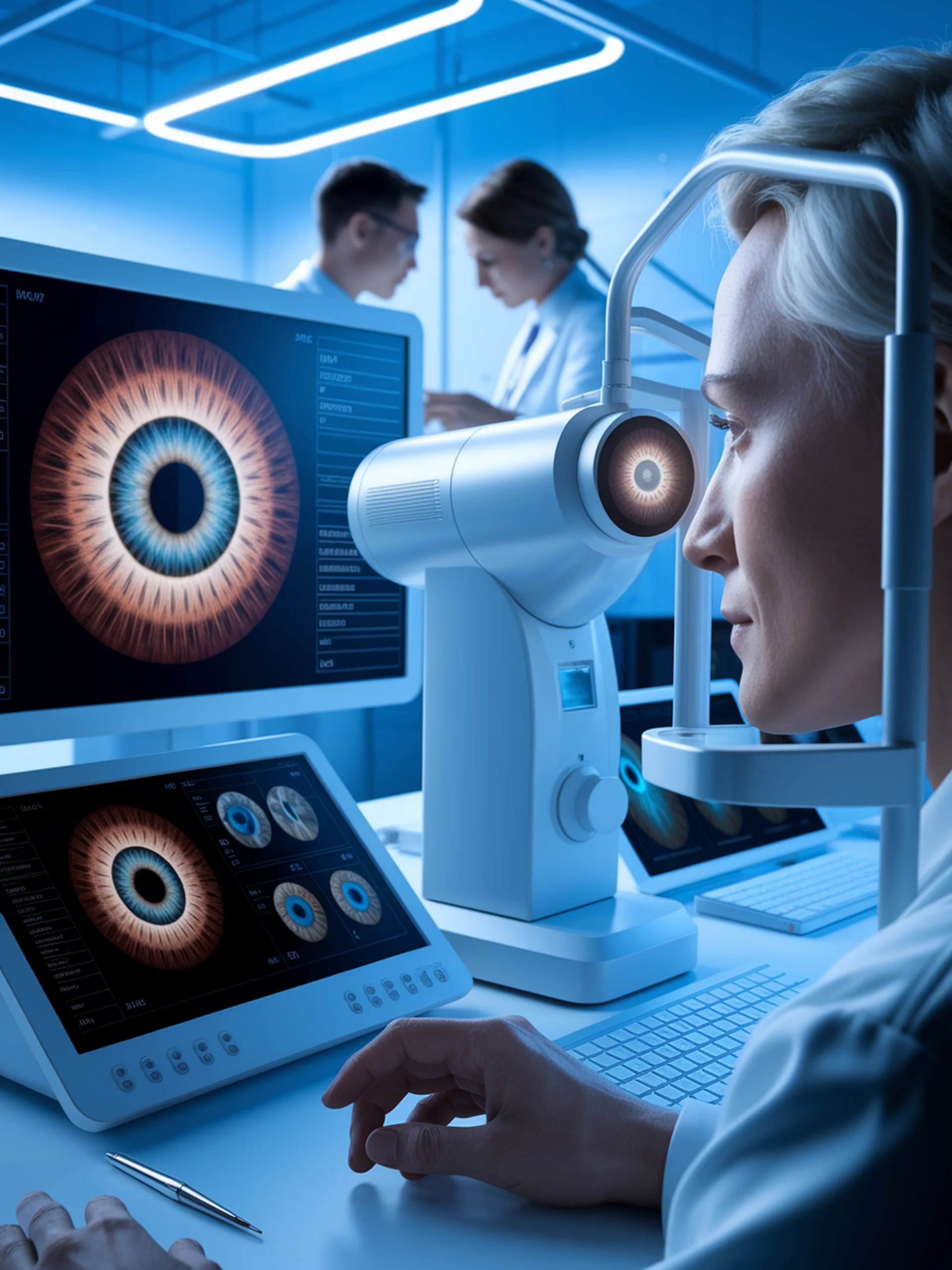
Next-Gen LLMs in Ophthalmology
Head-to-head evaluation reveals performance gaps in medical reasoning
This research presents a comprehensive evaluation of reasoning-focused Large Language Models in ophthalmology, testing four cutting-edge models across 5,888 medical questions and scenarios.
- DeepSeek-R1 demonstrated superior performance (86.8% accuracy), outperforming human ophthalmology residents
- All models showed strength in foundational knowledge but struggled with complex clinical reasoning
- Significant performance gaps exist between models, with OpenAI o1 trailing at 74.7% accuracy
- Models excelled in ophthalmology basics but showed weaknesses in clinical decision-making
These findings are crucial for medical implementations as they highlight both the promise and limitations of AI in specialized healthcare settings, suggesting careful validation is needed before clinical deployment.