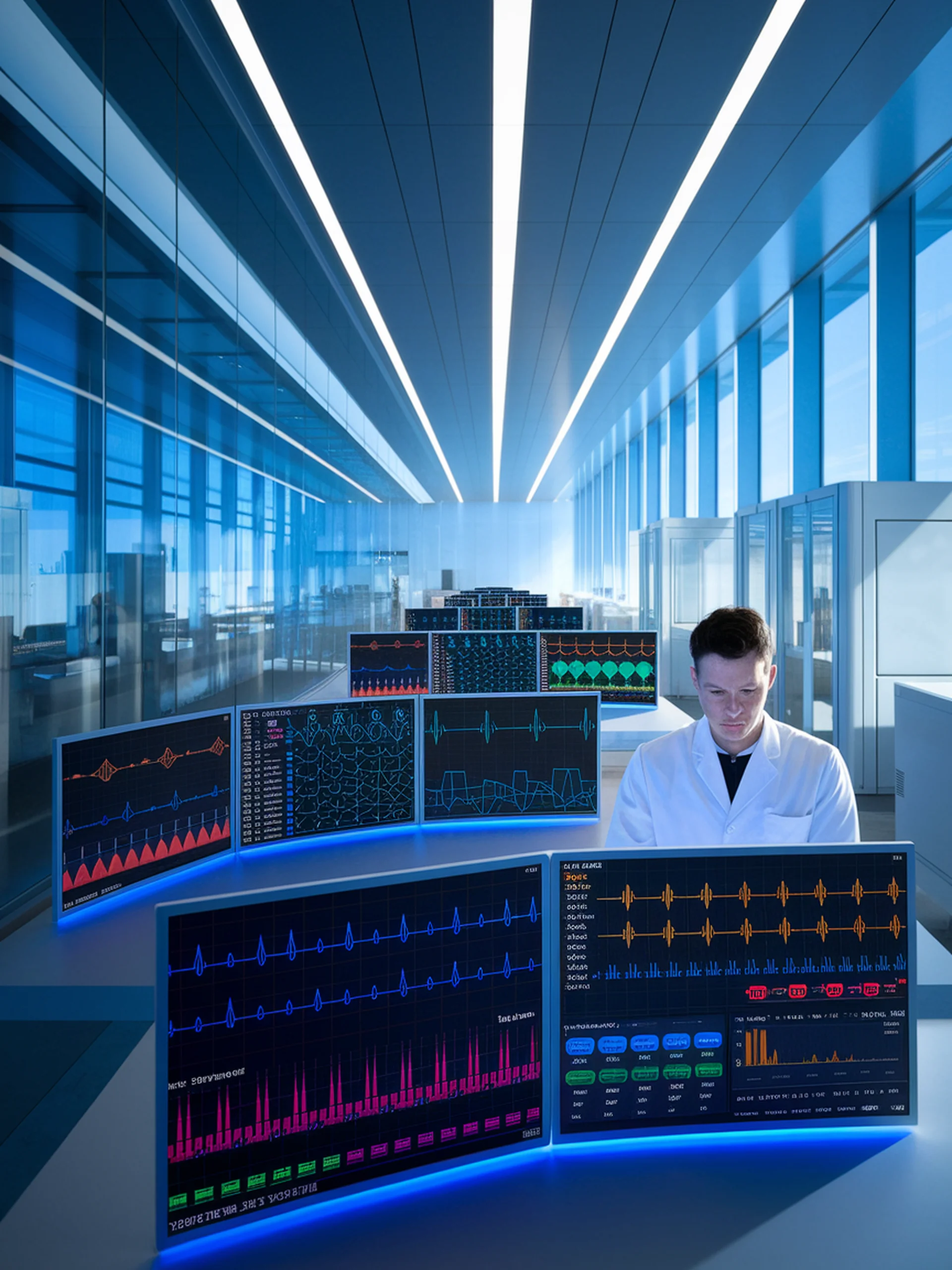
Bridging the Gap in Time Series Analysis
Advancing foundation models for diverse time series data across domains
This research addresses the challenge of applying foundation models to time series data by tackling the inherent heterogeneity across different domains.
- Develops a novel approach for handling varying characteristics in time series data (variate count, sampling rates)
- Enables cross-domain generalization similar to breakthroughs in NLP and computer vision
- Creates foundation models capable of handling diverse tasks across medical, financial, and other technical fields
- Proposes methods to overcome the complexity barriers that have limited previous time series analysis
For the medical domain, this research offers significant potential for improved patient monitoring systems, diagnostic tools, and predictive healthcare analytics by enabling the transfer of knowledge across different medical time series applications.
Towards Generalisable Time Series Understanding Across Domains