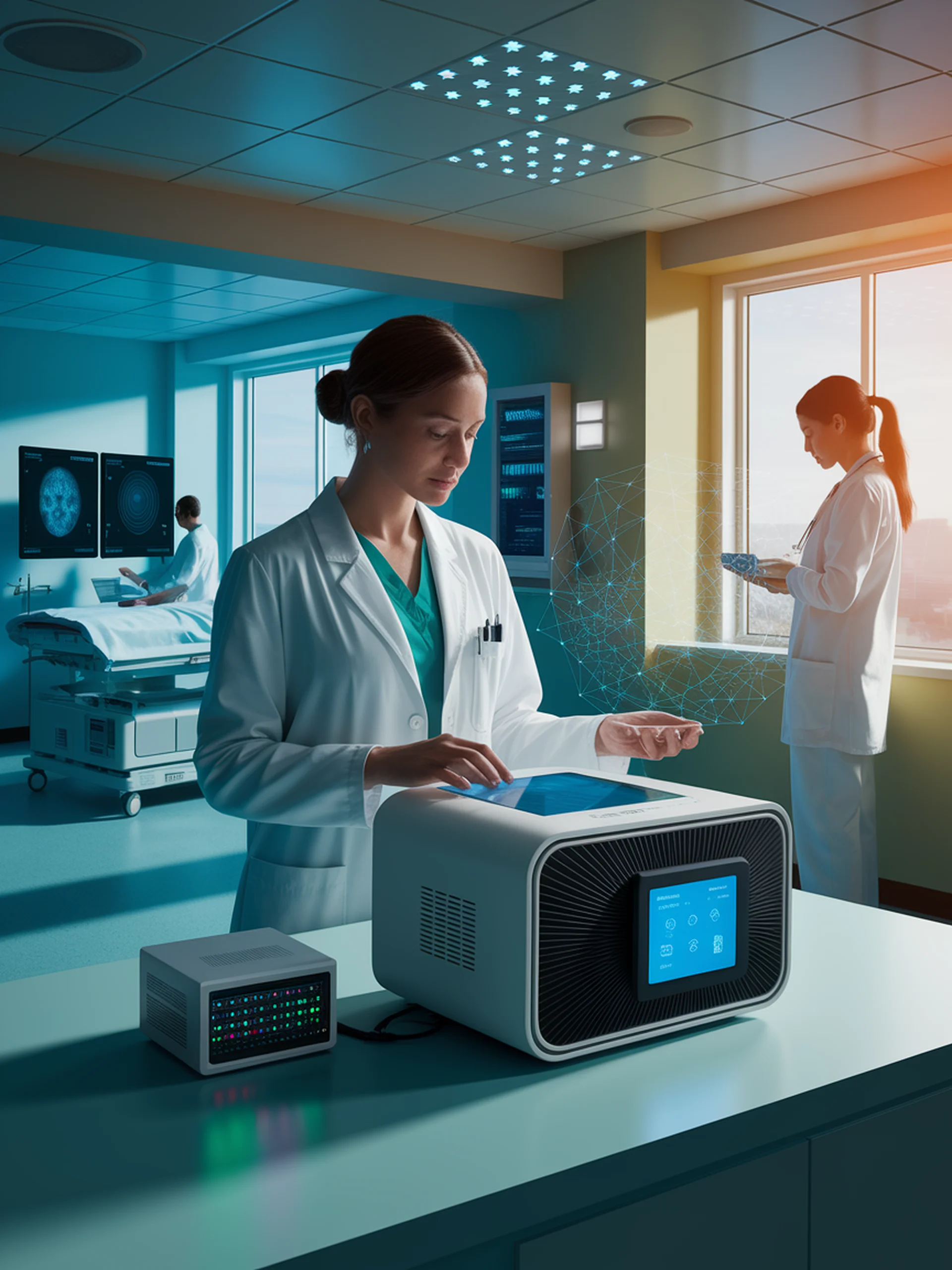
Efficient Multimodal AI for Resource-Constrained Settings
Domain adaptation through contrastive learning for healthcare applications
This research introduces a novel approach to adapt powerful multimodal models for deployment in computationally-limited environments like healthcare facilities.
- Combines foundational models with supervised ML to enable automated diagnosis and treatment planning
- Uses contrastive learning techniques to efficiently adapt multimodal embeddings to specific domains
- Addresses the critical challenge of limited onsite computational resources in healthcare settings
- Demonstrates how complex AI systems can be optimized for real-world medical applications
This research is particularly valuable for medical institutions seeking to implement advanced AI capabilities without requiring massive computational infrastructure, potentially democratizing access to cutting-edge diagnostic tools.
Efficient Domain Adaptation of Multimodal Embeddings using Constrastive Learning