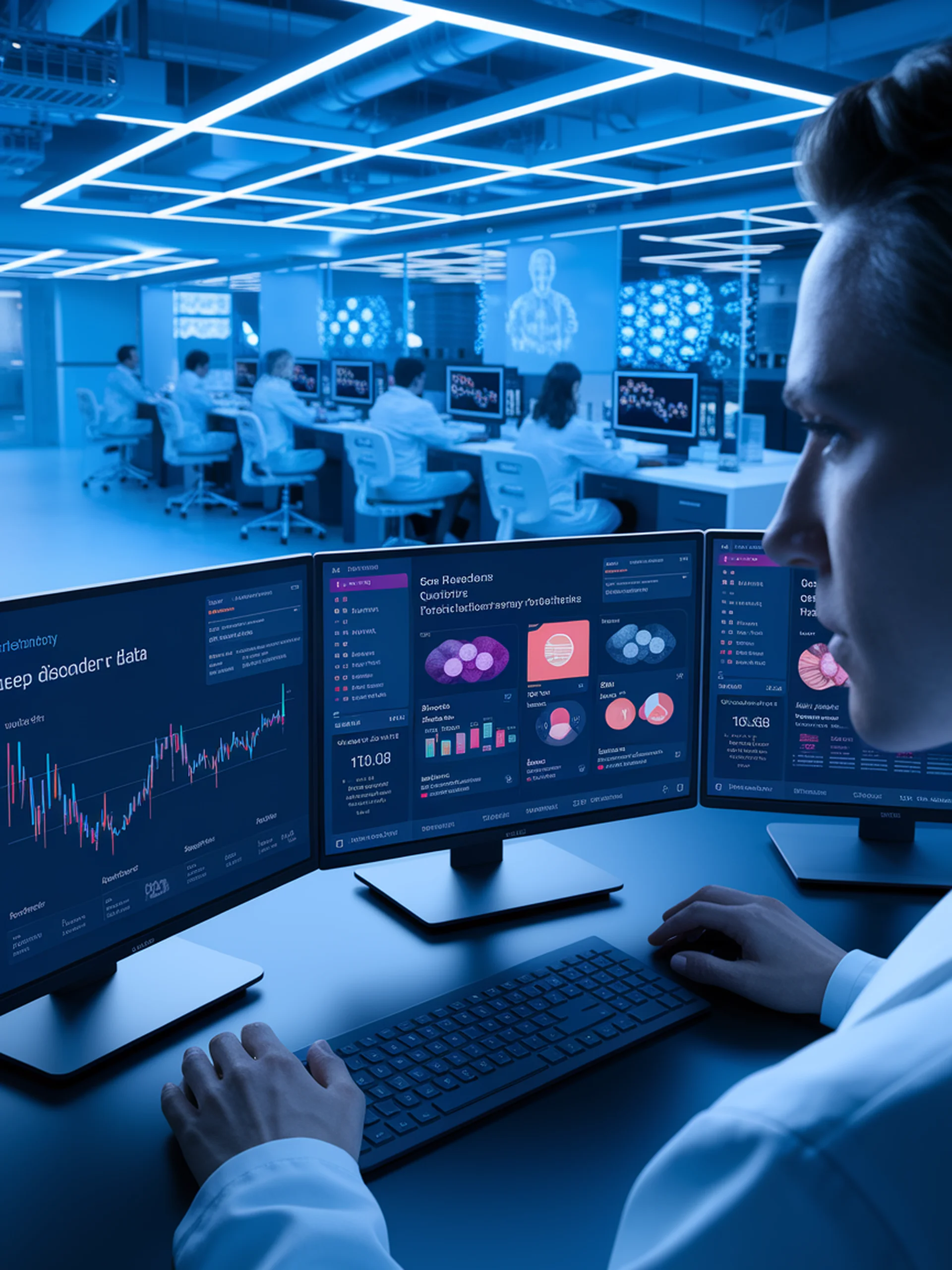
Zero-Shot LLMs vs. Crowdsourcing for Public Health
Enhancing efficiency and accuracy in social media health data labeling
This research evaluates how large language models can transform public health data annotation compared to traditional crowdsourcing approaches.
- Zero-shot learning with LLMs offers comparable or superior accuracy to crowdsourced methods
- Significant cost and time reduction when using LLMs for health-related social media data labeling
- Applied successfully to identify patterns in sleep disorders, physical activity, and sedentary behavior
- Provides a scalable solution for processing large volumes of health data from social platforms
This advancement matters for medical research by enabling more efficient analysis of population health trends through social media, potentially leading to faster insights and more responsive public health interventions.