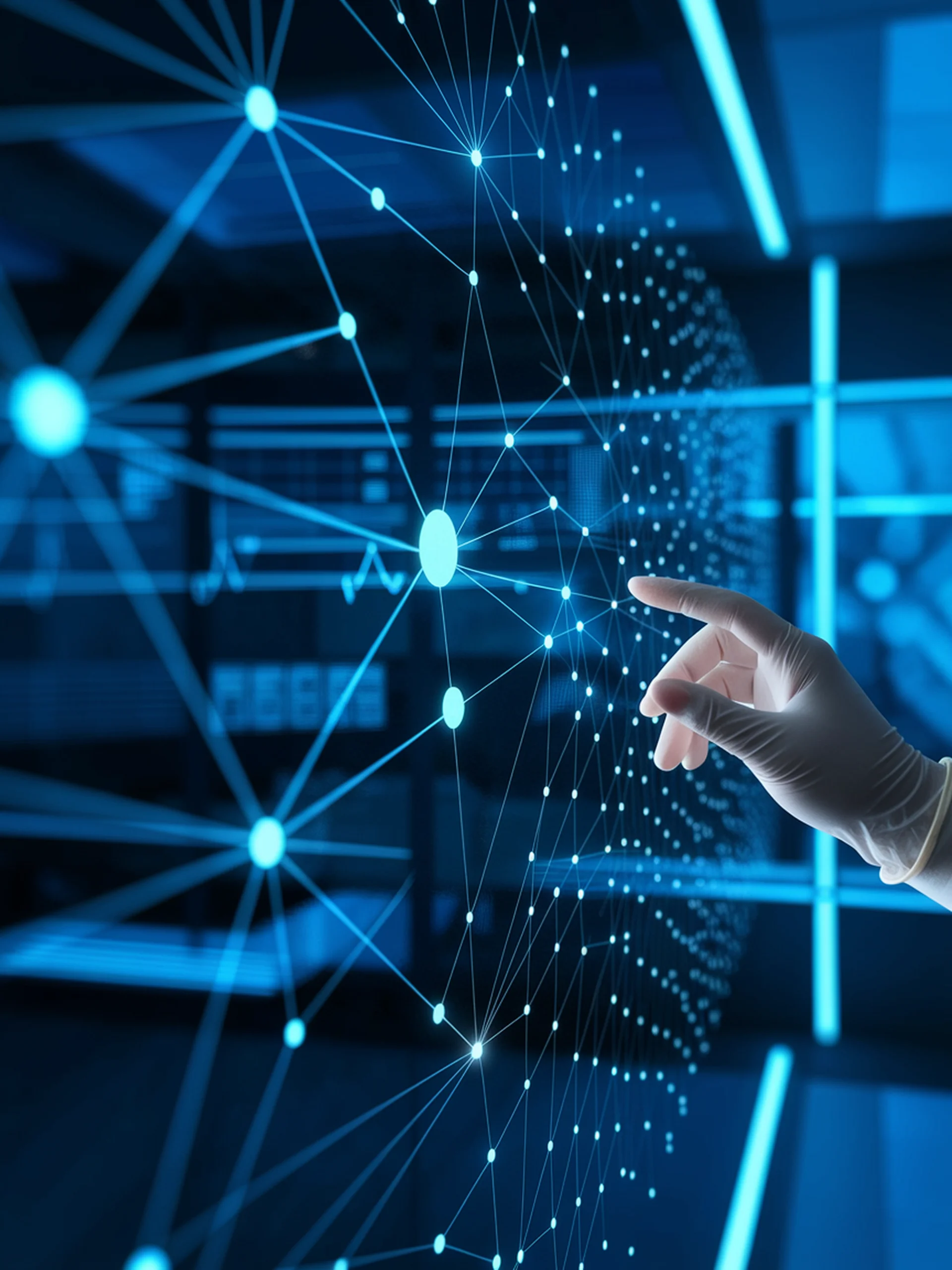
Unlocking Graph Data Potential with LLMs
A democratized approach for augmenting graph data through latent knowledge graphs
This research introduces a novel black-box LLM approach for graph data augmentation that leverages latent knowledge graphs without requiring direct access to LLM weights or features.
- Creates high-quality synthetic graph data to address data scarcity challenges
- Captures contextual information from datasets that traditional methods miss
- Demonstrates superior performance in healthcare data scenarios
- Makes advanced LLM-based graph augmentation accessible without technical barriers
This innovation is particularly valuable for medical applications with electronic health records (EHRs), where data quality and privacy are critical concerns. The approach enables healthcare organizations to enhance their graph-based analysis while maintaining data security and improving representational learning.
Democratizing Large Language Model-Based Graph Data Augmentation via Latent Knowledge Graphs