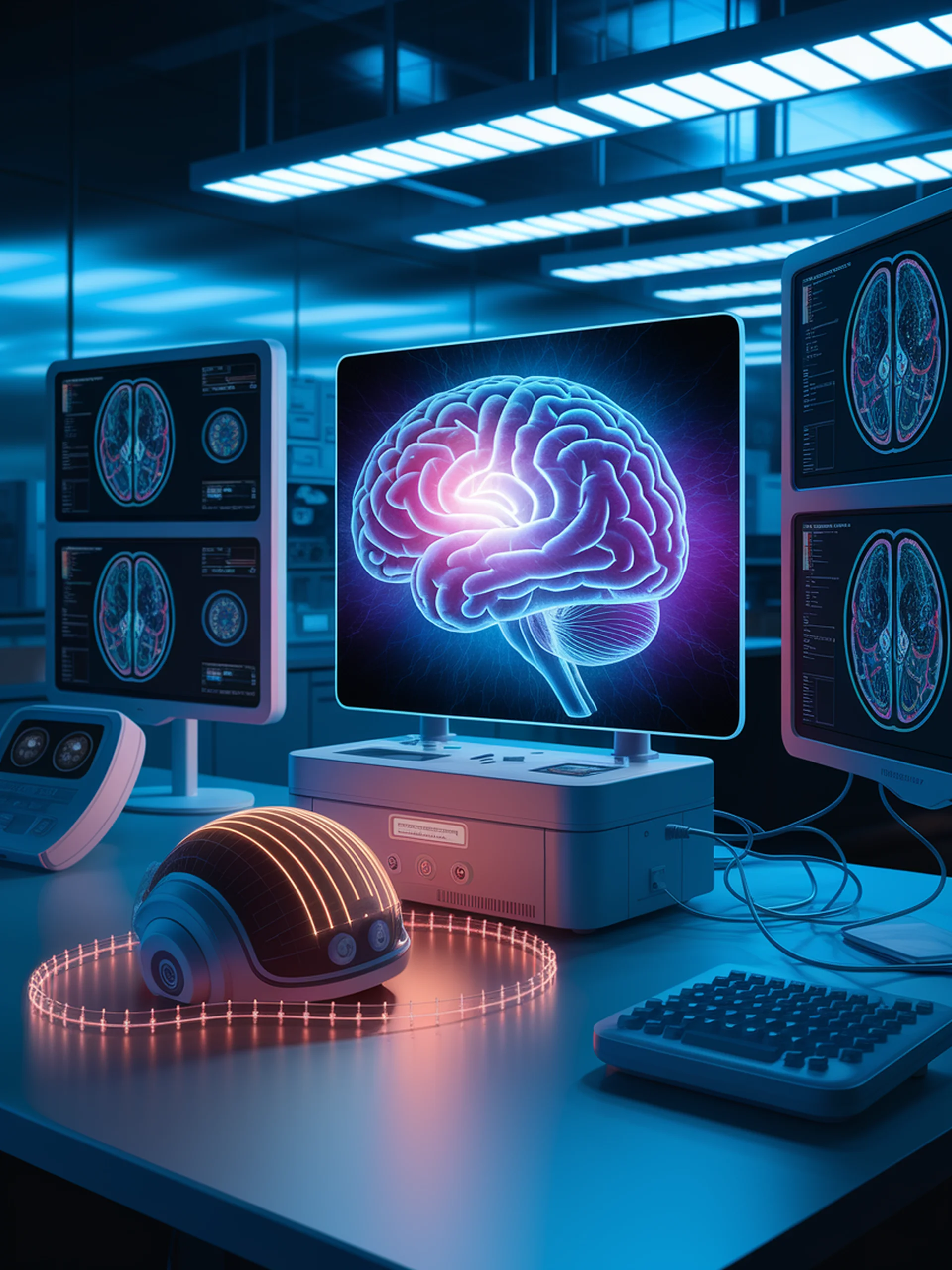
Decoding Visual Hallucinations with AI
Using LLMs to analyze subjective experiences of light-induced visual phenomena
This research combines topic modeling and large language models to analyze subjective reports of stroboscopic light-induced visual hallucinations, creating a novel framework for computational phenomenology.
- Developed MOSAIC methodology to classify 862 sentences from 422 subjective reports
- Applied LLMs to extract and interpret patterns in visual hallucination descriptions
- Created an interpretable framework for analyzing subjective experiences across participants
- Demonstrated how AI can bridge quantitative analysis with qualitative, experiential data
This approach offers significant medical applications by providing structured analysis of neurological experiences, potentially aiding in understanding altered states of consciousness and their neurological underpinnings.