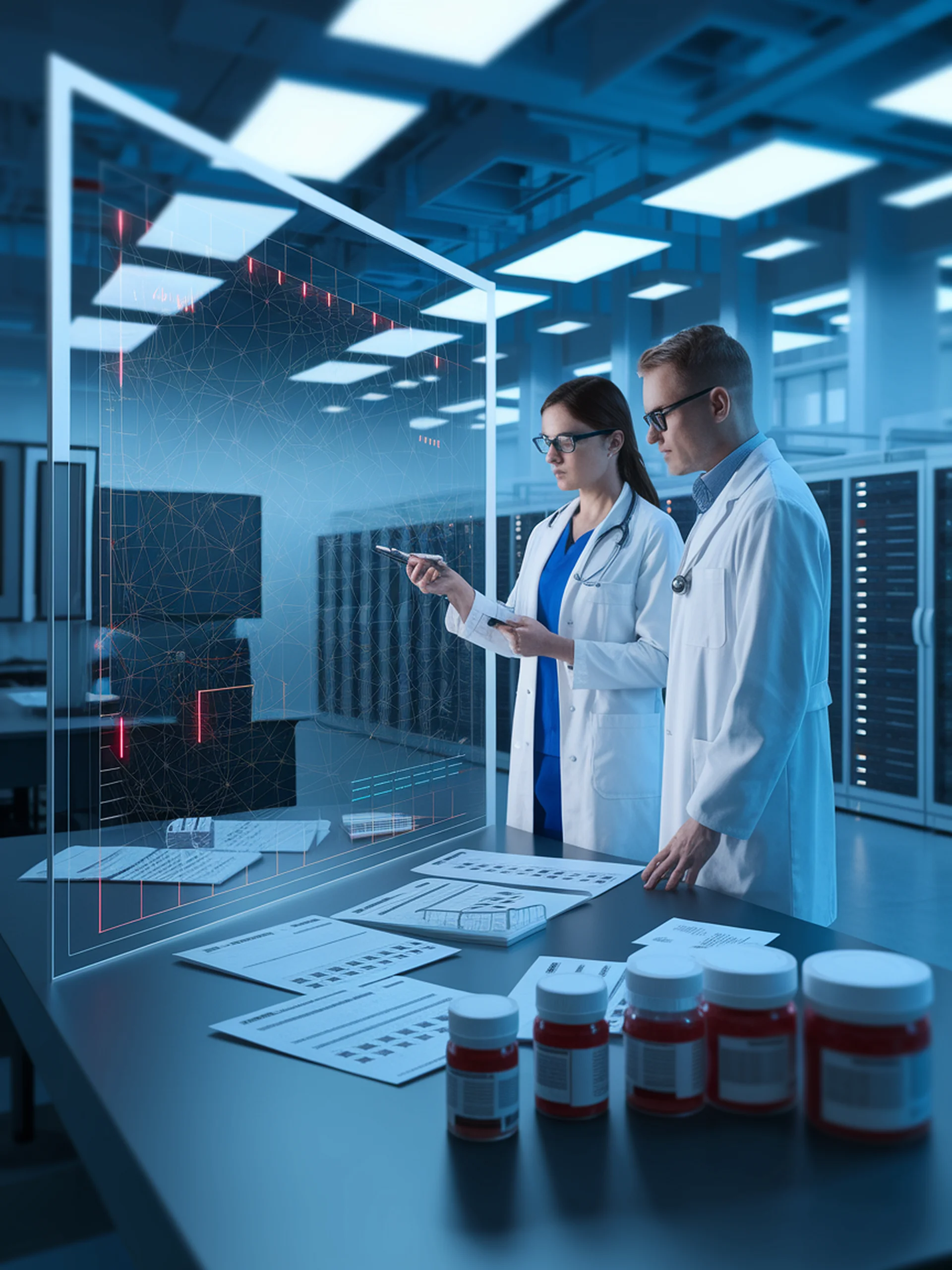
The Hidden Bias in Medical AI
How Patient Non-Adherence Distorts ML Models in Healthcare
This research reveals how patient medication non-adherence creates implicit bias in clinical machine learning models, potentially leading to flawed treatment recommendations.
- Study analyzed EHR data from 3,623 hypertension patients, extracting adherence information from clinical notes
- Non-adherence to prescribed treatments fundamentally distorts both causal inference and predictive modeling
- ML systems trained on incomplete adherence data may recommend inappropriate or potentially harmful treatments
- Demonstrates the critical need for adherence-aware ML model development in clinical settings
Why it matters: For healthcare organizations implementing AI, this work highlights a significant but often overlooked source of bias that could compromise patient outcomes and undermine trust in medical AI systems.