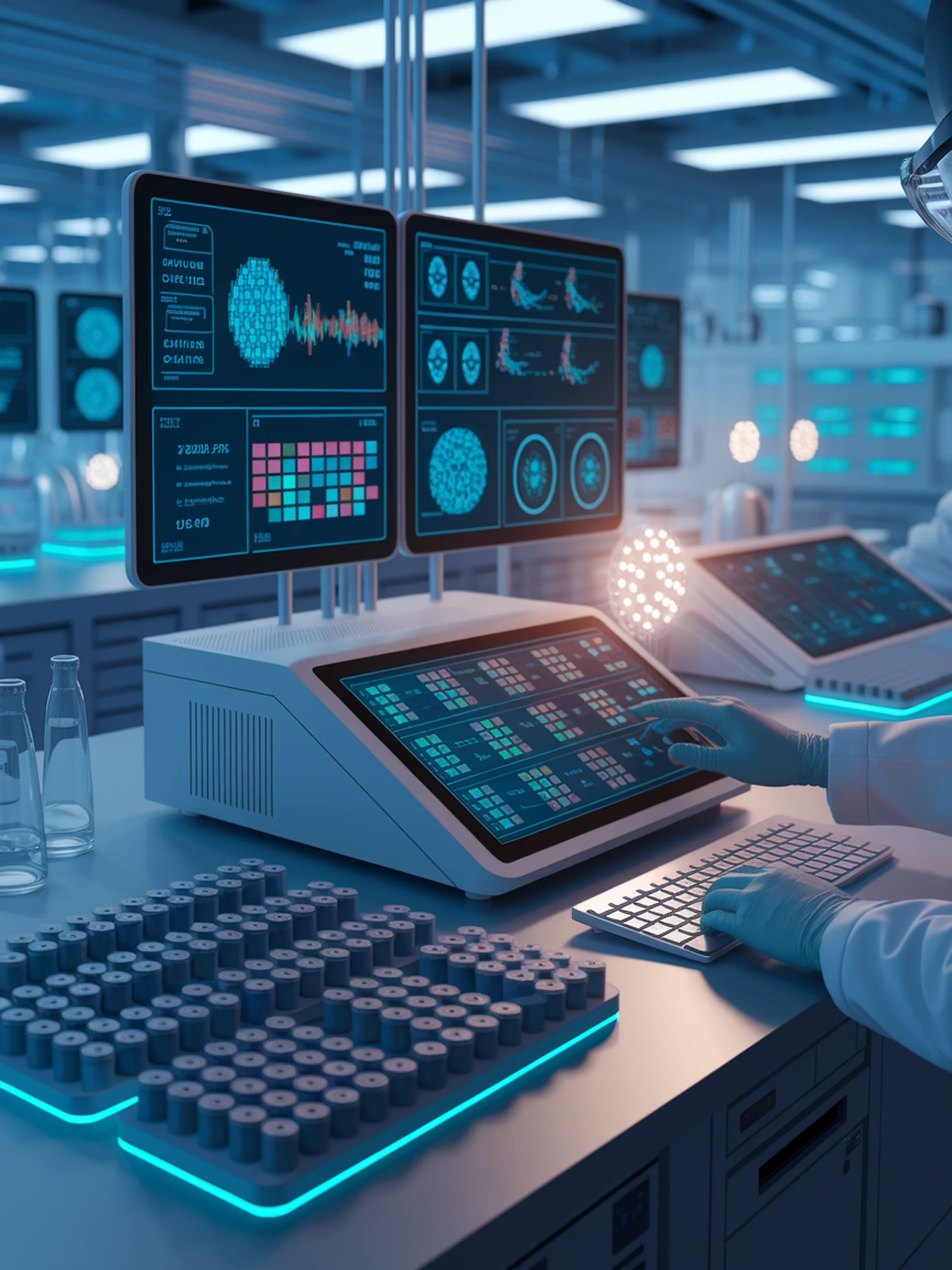
Smart Sample Selection for Medical LLMs
A choice-based greedy approach to enhance model performance while reducing training costs
This research introduces a novel incremental sample selection method for training Large Language Models that significantly improves efficiency and performance in medical applications.
- Evaluates training samples based on overall dataset value rather than individual quality
- Achieves better balance between diversity and efficiency in data selection
- Demonstrated effectiveness on large medical datasets with reduced computational overhead
- Provides a practical framework for optimizing medical LLM training without extensive data traversal
For healthcare organizations, this approach enables more cost-effective development of specialized medical AI systems while maintaining high performance standards.