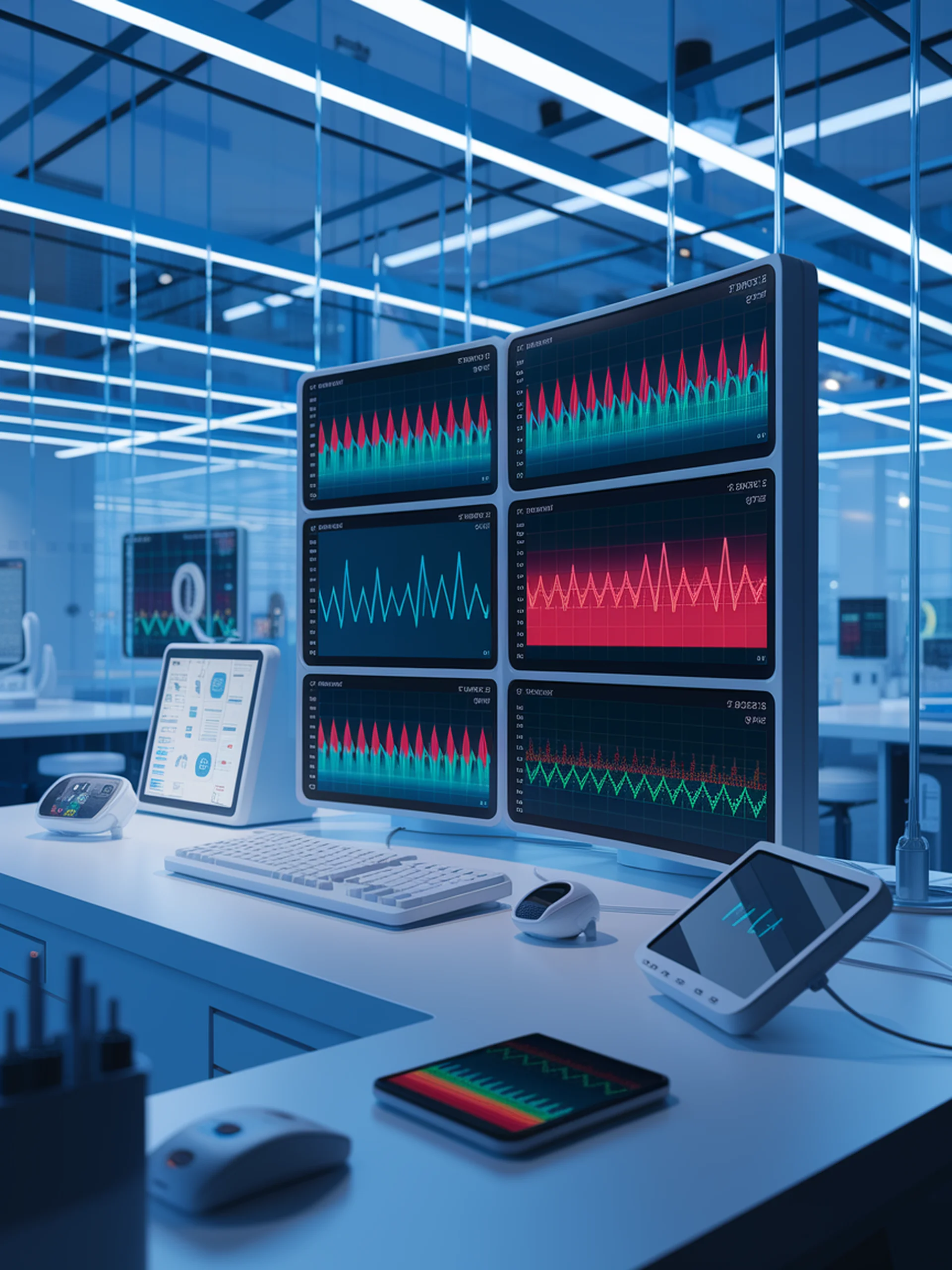
Enhancing Time Series Forecasting with LLMs
Leveraging temporal patterns and semantics for superior predictions
This research introduces LLM-PS, a novel approach that enhances Large Language Models' forecasting capabilities by incorporating temporal patterns and semantic understanding of time series data.
- Addresses key limitations of existing LLM-based forecasting by recognizing time series' unique characteristics
- Combines pattern extraction with semantic enrichment to improve prediction accuracy
- Demonstrates superior performance across multiple forecasting benchmarks compared to traditional methods
- Provides a framework adaptable to various time horizons and data types
For healthcare applications, this approach offers improved patient monitoring through more accurate predictions of vital signs, disease progression, and treatment responses—potentially enabling earlier intervention and personalized care.