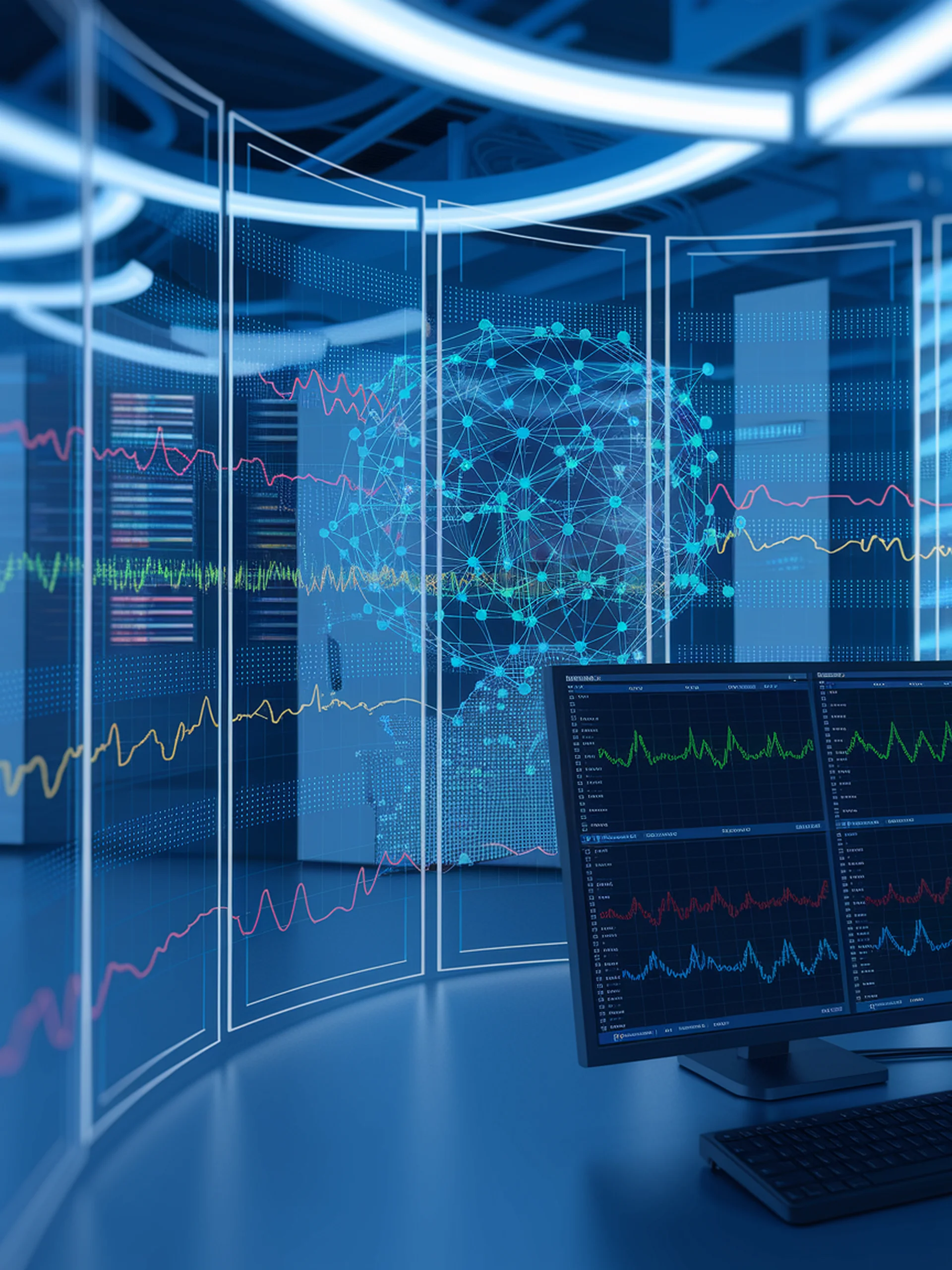
Unlocking Time Series Potential with Synthetic Data
How Foundation Models Are Revolutionizing Time Series Analysis
This research explores how synthetic data generation can overcome limitations in time series analysis, particularly when leveraging foundation models for complex systems.
- Foundation models (TSFMs and TSLLMs) enable task-agnostic learning for time series data
- Data constraints (regulatory, diversity, quality, quantity) limit the effectiveness of these models
- Synthetic data provides a promising solution to address these limitations
- Applications span engineering systems for predictive maintenance, anomaly detection, and complex systems modeling
For engineering professionals, this research provides frameworks to generate high-quality synthetic time series data that can enhance model performance while addressing data scarcity and privacy concerns.