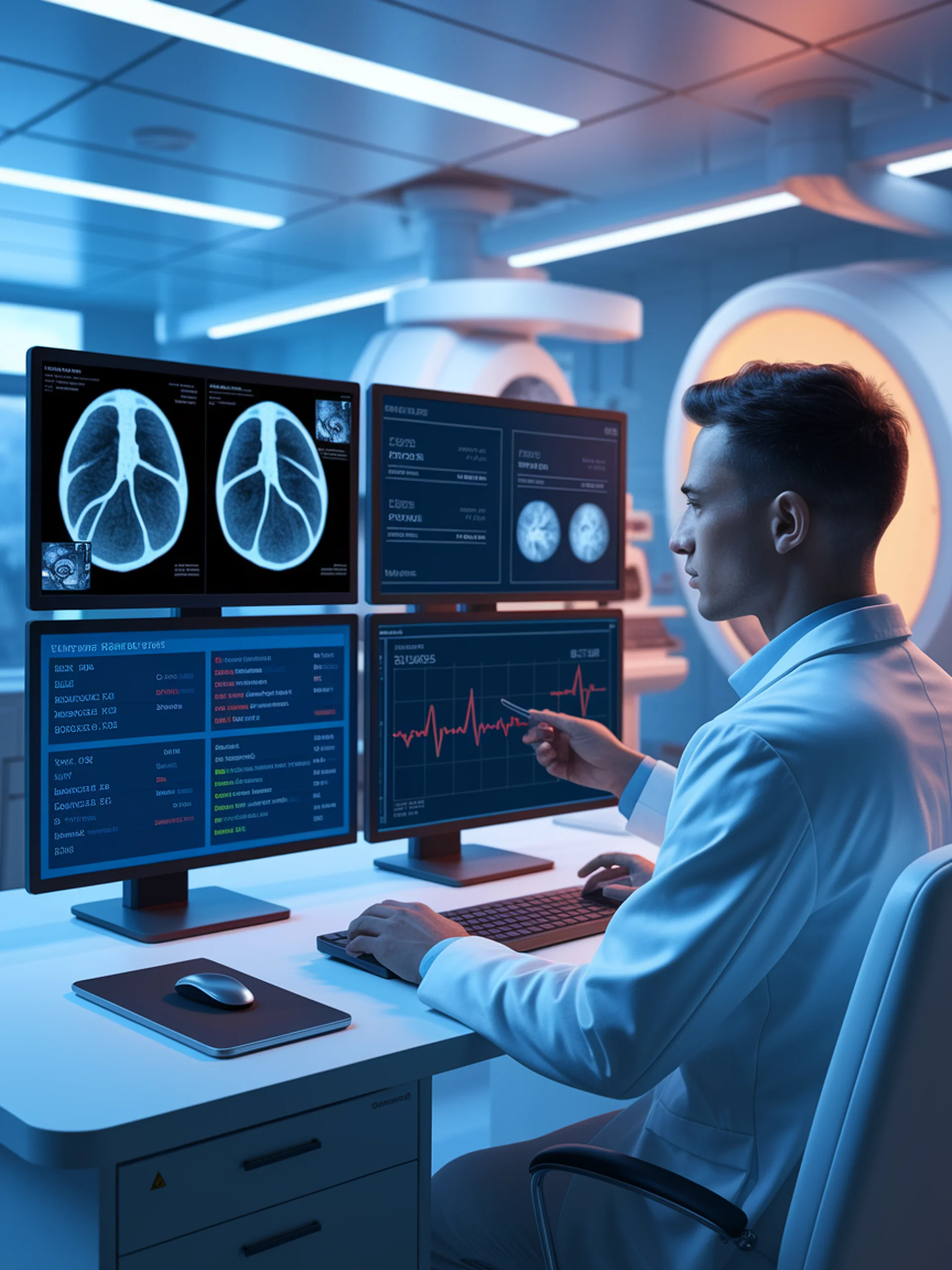
AI Streamlining Clinical Documentation
LLMs Show Promise for Automated Pulmonary Embolism Data Extraction
This research evaluates how large language models can automate the extraction of critical clinical data from radiology reports, potentially reducing manual abstraction workload in pulmonary embolism registries.
- GPT-4 achieved 87% accuracy in extracting key clinical concepts, outperforming smaller models
- Performance improved with temperature reduction and careful prompt engineering
- Model performance varied across different clinical variables, with better results on clearly documented concepts
- Zero-shot prompting proved effective, suggesting practical real-world application potential
This advancement could significantly improve medical registry efficiency, enhance research capabilities, and ultimately lead to better evidence-based pulmonary embolism care protocols.