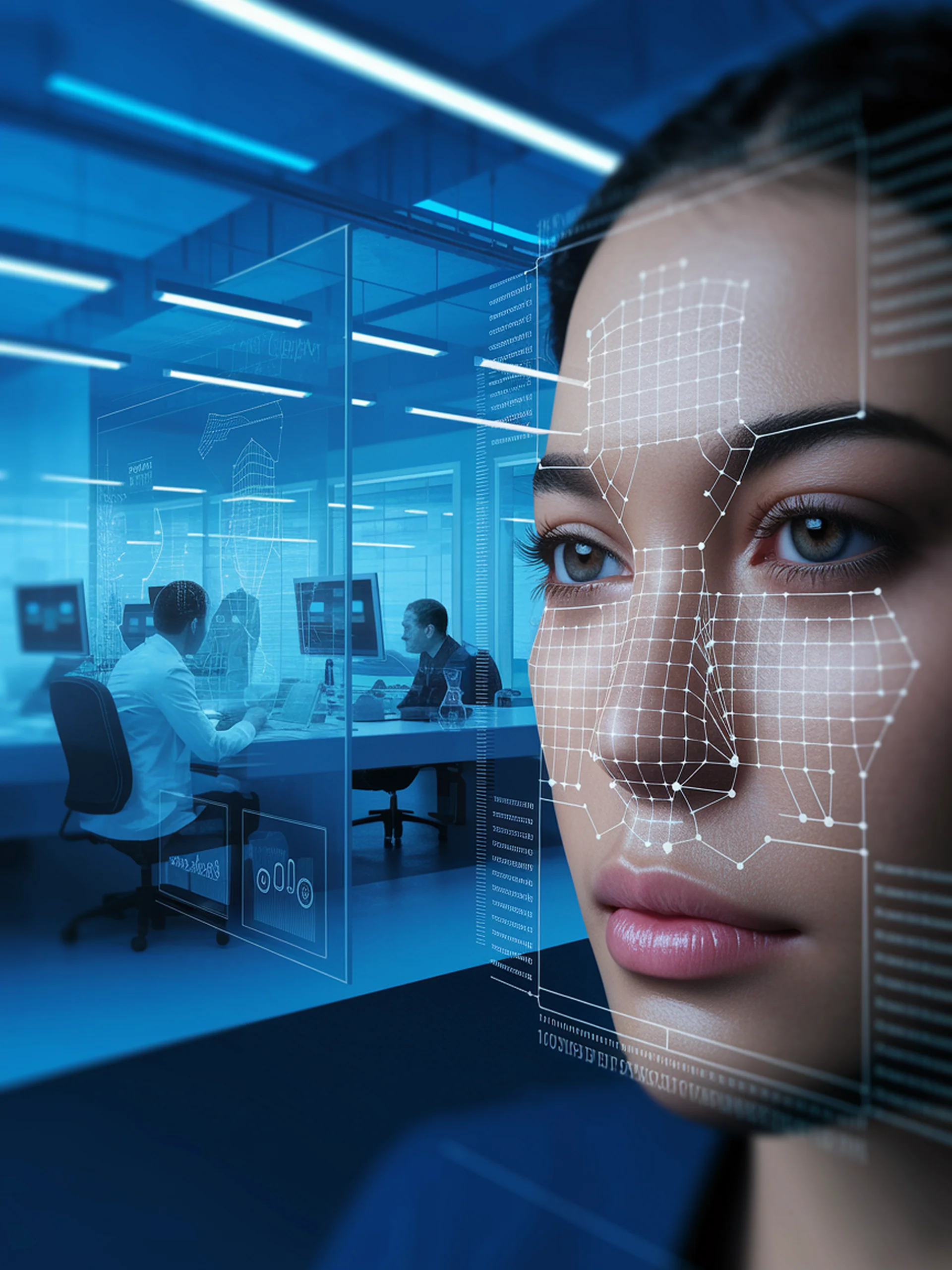
FaceBench: Evaluating Face Perception in AI Models
A hierarchical benchmark for assessing MLLMs' facial recognition capabilities
FaceBench introduces a comprehensive dataset to evaluate how well multimodal large language models (MLLMs) can perceive and analyze human faces across multiple attributes and difficulty levels.
- Creates a hierarchical facial attribute structure with five distinct viewpoints
- Enables systematic evaluation of MLLMs' face perception capabilities
- Identifies current limitations and biases in facial recognition technologies
- Provides a benchmark for security applications in biometric identification and authentication systems
This research is particularly valuable for security professionals developing facial recognition systems, as it establishes standardized metrics for evaluating how AI systems process and interpret facial data—critical for ensuring accurate and fair identification technologies.