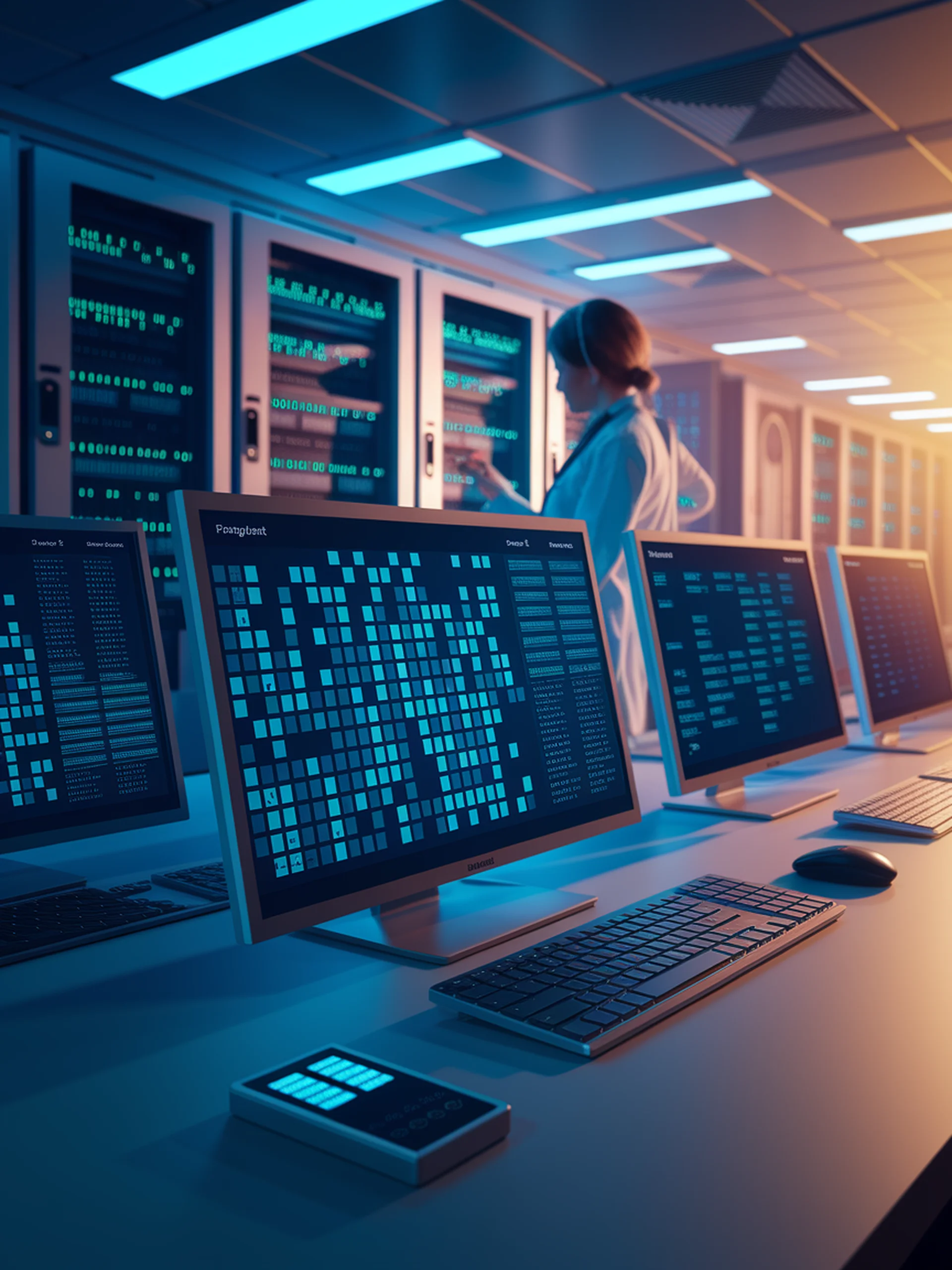
Rethinking Privacy in AI Decision Systems
New privacy paradigms for RL and LLMs in sequential decisions
This position paper proposes a fundamental reconceptualization of privacy for reinforcement learning systems in high-stakes domains like healthcare and security.
- Traditional privacy frameworks fail to protect temporal patterns and behavioral strategies in sequential decision-making
- Modern paradigms like Federated RL and RLHF in LLMs create new privacy vulnerabilities
- The paper advocates for domain-specific privacy measures that address the unique challenges of sequential AI systems
- Research highlights critical privacy gaps in current approaches to AI deployment in sensitive contexts
For security professionals, this work provides essential insights into emerging privacy threats as RL systems become more prevalent in critical infrastructure and sensitive applications.
Position Paper: Rethinking Privacy in RL for Sequential Decision-making in the Age of LLMs