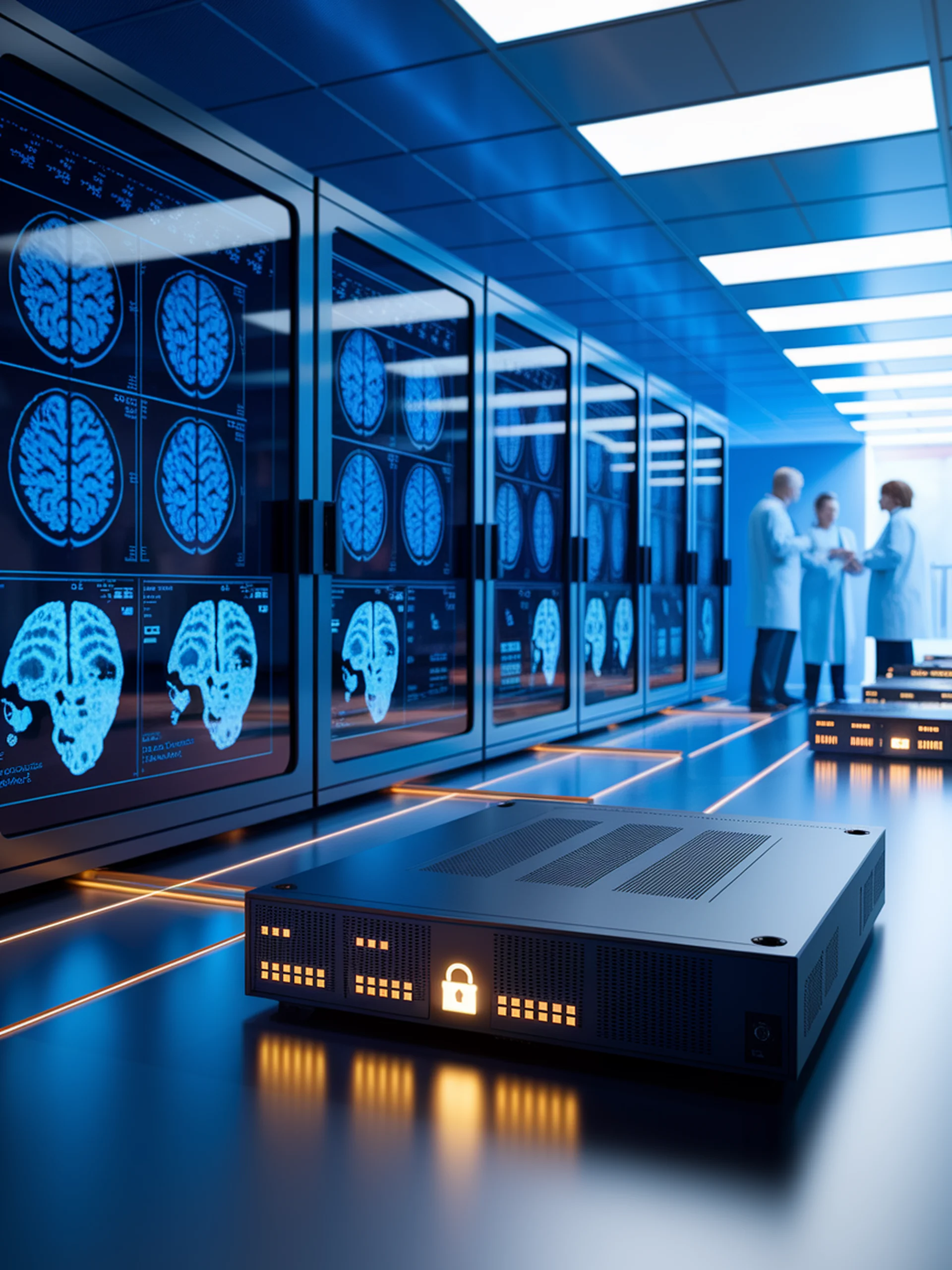
Federated CLIP for Medical Imaging
Adapting Vision-Language Models for Distributed Healthcare Applications
This research addresses the challenge of deploying large vision-language models (like CLIP) in distributed healthcare environments through a novel federated learning approach.
- Federated Adversarial Adaptation technique significantly reduces model size while maintaining performance
- Effectively handles data heterogeneity across different medical clients
- Demonstrates improved generalization performance on medical imaging datasets
- Preserves privacy by keeping sensitive medical data on local devices
This research enables healthcare organizations to leverage powerful vision-language models across distributed systems while addressing critical concerns around data privacy, computational efficiency, and performance in diverse medical contexts.