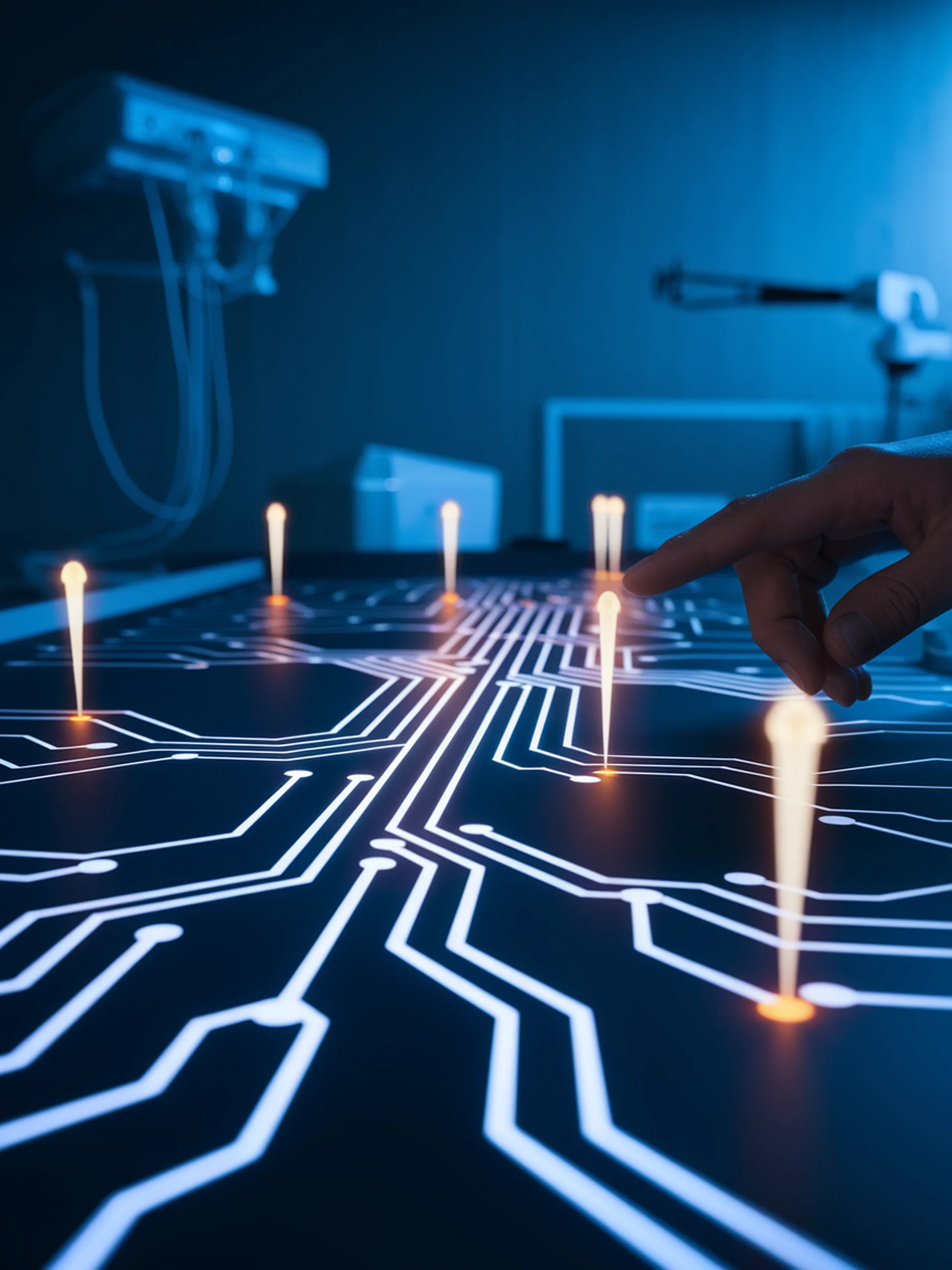
Confidence-Based LLM Routing
Enhancing AI reliability through self-assessment mechanisms
This research introduces a framework for large language models to assess their own confidence and route queries accordingly, improving safety and reliability in high-stakes environments.
- LLMs can be trained to generate special tokens that indicate their confidence level in responses
- High-confidence answers show significant performance advantages over unrouted responses
- Response routing (to experts or default behaviors) based on confidence signals improves overall system reliability
- Particularly valuable for security applications where preventing unreliable AI outputs is critical
Business impact: Organizations can implement more trustworthy AI systems that recognize their limitations and appropriately escalate uncertain cases, reducing risks in sensitive applications.