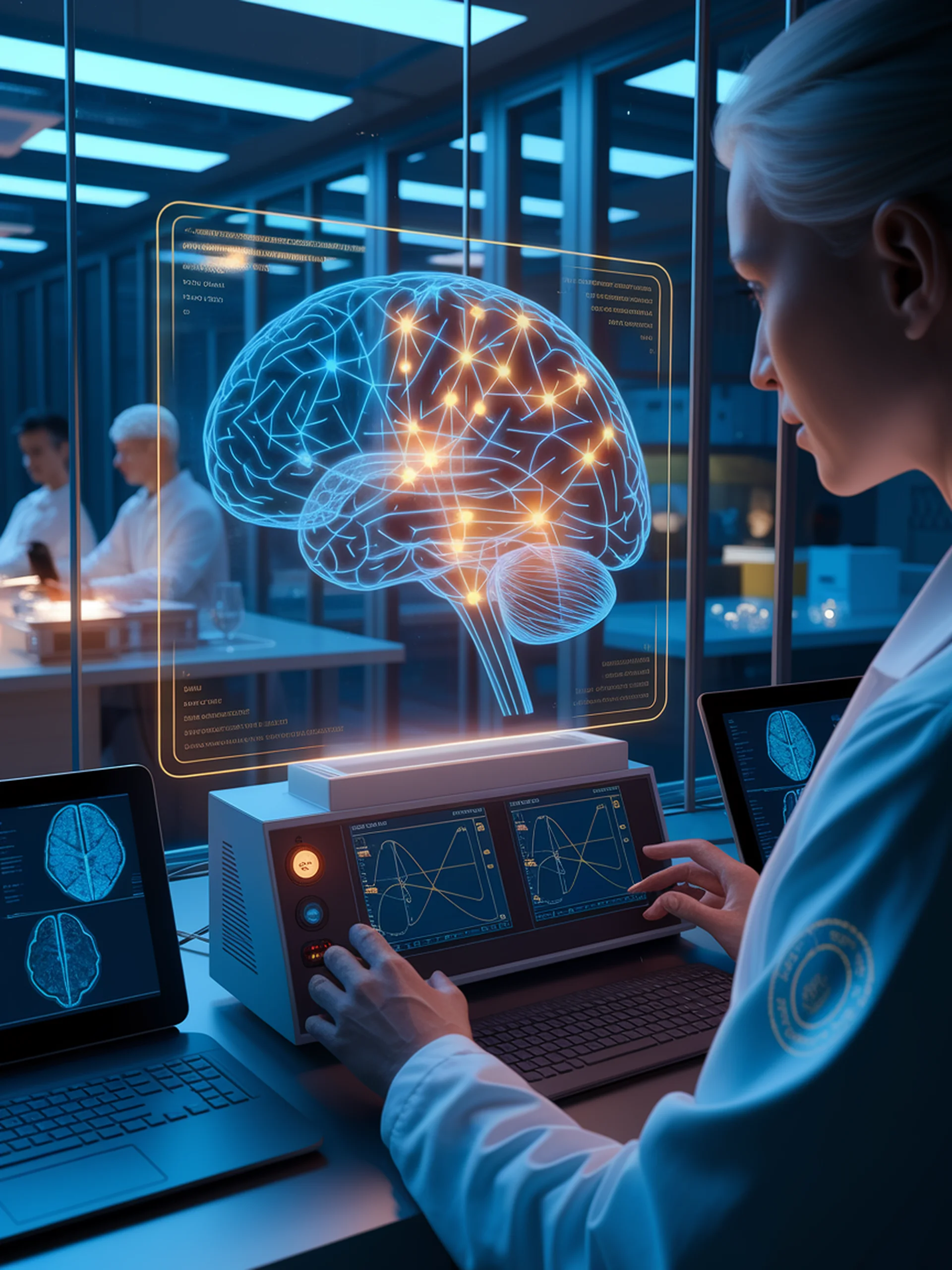
Overcoming Catastrophic Forgetting in LLMs
A Novel Hierarchical Regularization Approach
This research introduces a parameter-specific regularization technique that preserves general knowledge while fine-tuning LLMs for specialized domains.
- Hierarchical regularization at both layer and element levels prevents knowledge loss
- Calculates importance scores for individual model parameters to protect critical knowledge
- Demonstrates significant improvements in maintaining general capabilities while acquiring domain expertise
- Validated on medical applications with GPT-J and LLaMA-3 models
Why It Matters for Medicine: This approach enables the creation of specialized medical LLMs that retain broad knowledge while gaining domain expertise, potentially improving clinical decision support and medical research applications without requiring complete retraining.