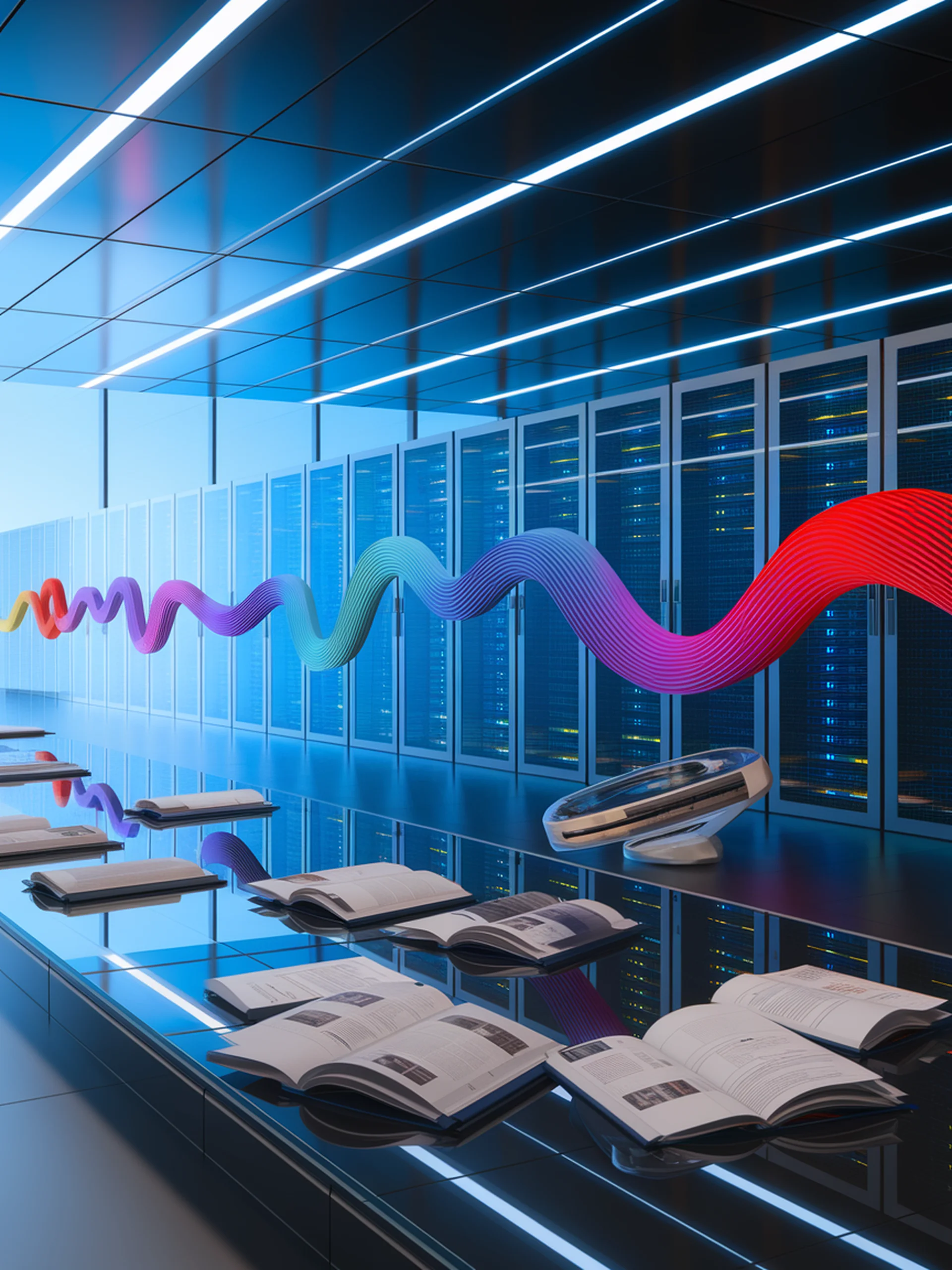
Tracking Topics Through Time
Advanced Analysis of Academic Journal Evolution Using Convex Optimization
This research introduces a two-stage dynamic topic analysis framework that enhances AI's ability to track how academic topics evolve over time, with superior consistency and interpretability.
- Employs convex non-negative matrix factorization to improve topic consistency across time periods
- Creates more interpretable and sparse topic representations compared to traditional methods
- Analyzes large-scale IEEE journal data to identify key topic trends across disciplines
- Enables better knowledge organization and discovery in academic research
For education stakeholders, this framework offers powerful tools to map curriculum relevance against emerging research trends, identify cross-disciplinary opportunities, and guide strategic research investment decisions.
Dynamic Topic Analysis in Academic Journals using Convex Non-negative Matrix Factorization Method