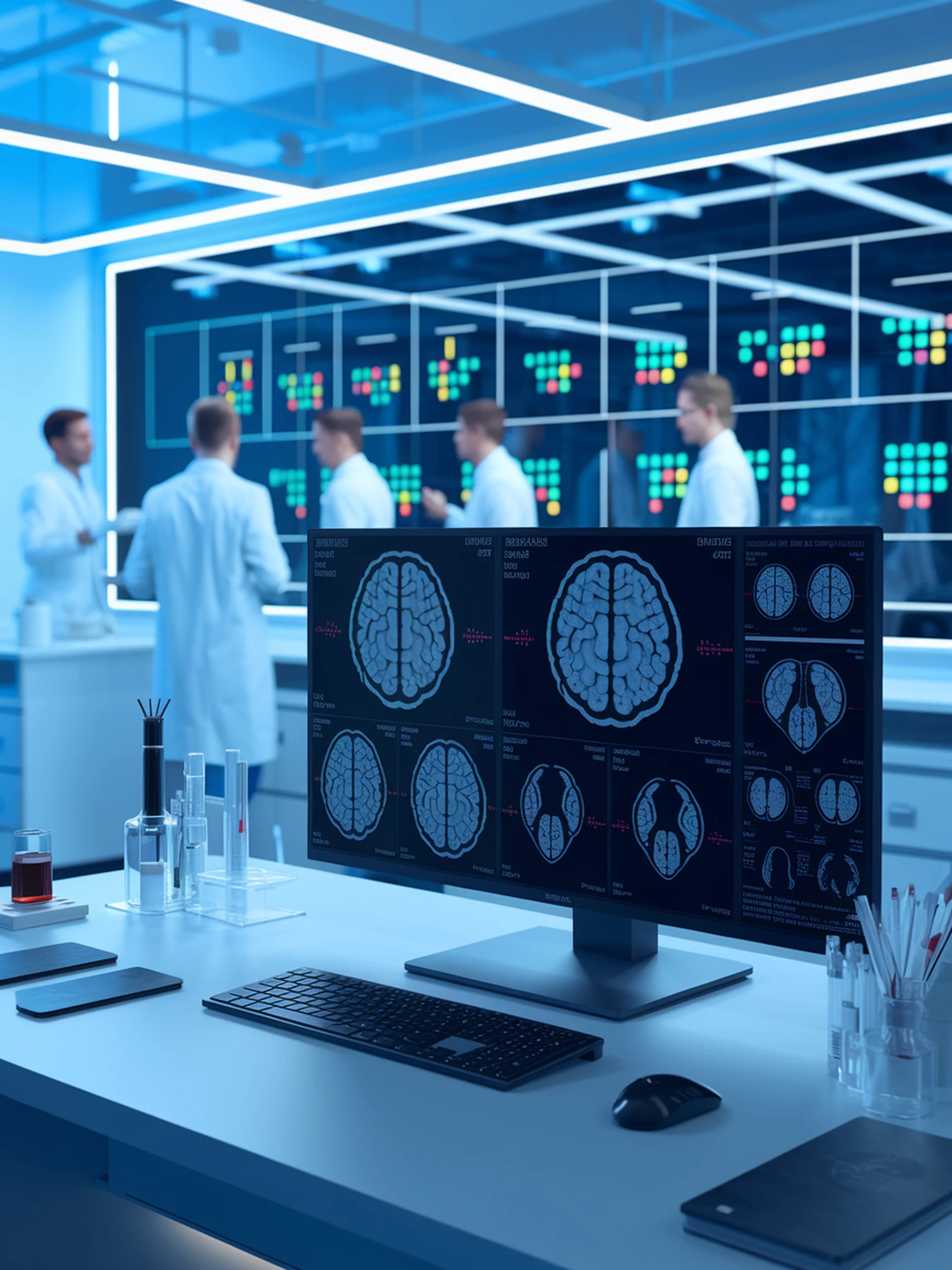
Enhancing Radiology AI with Uncertainty Awareness
Improving LLM accuracy in complex medical text extraction using agent-based approaches
This research introduces a novel uncertainty-aware framework that significantly improves the extraction of structured medical data from complex, non-English radiology reports.
- Achieved 95.4% accuracy on Hebrew Crohn's disease radiology reports using an open-source LLM (Falcon-7B)
- Developed a multi-agent system that assesses uncertainty and resolves complex cases through collaborative decision-making
- Demonstrated robust performance across reports from three different medical centers spanning 13 years
- Created system capable of detecting its own limitations and referring uncertain cases for human review
This innovation advances clinical research by enabling reliable extraction of structured data from unstructured medical texts, potentially accelerating discoveries while maintaining high diagnostic standards through appropriate AI-human collaboration.