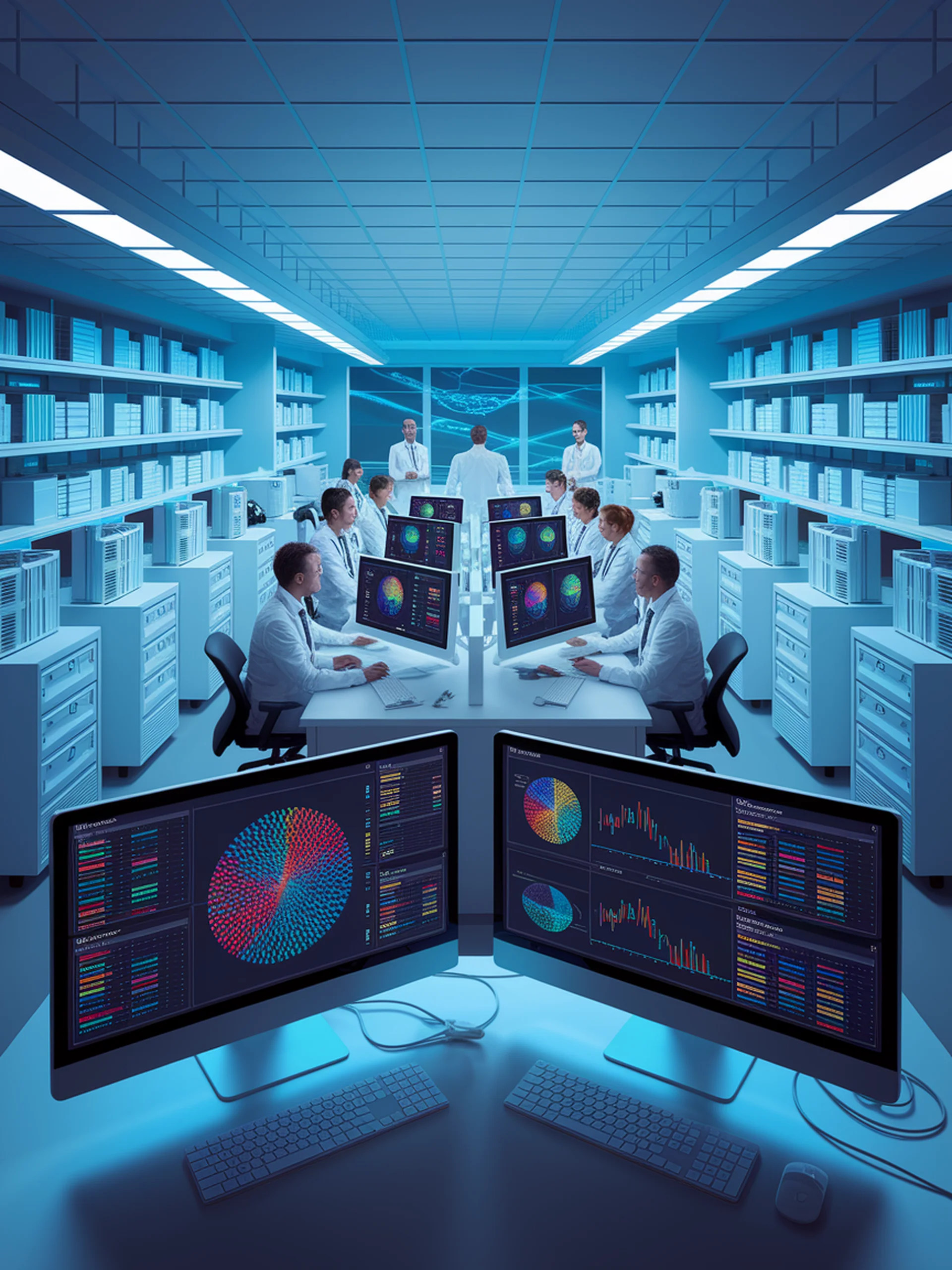
Enhancing Healthcare Text Classification with LLMs
Using ensemble learning to improve multi-label classification of medical narratives
This research introduces a novel framework that leverages ensemble learning with LLMs to tackle the complex challenge of classifying healthcare texts with multiple labels.
- Addresses the unique challenge of limited annotated healthcare text data
- Proposes QUAD-LLM-MLTC, an ensemble approach combining multiple Large Language Models
- Demonstrates superior performance compared to traditional machine learning approaches
- Enables more accurate automated categorization of medical narratives
This advancement matters for healthcare because it allows medical institutions to better organize, retrieve, and analyze the growing volume of unstructured clinical texts, improving information access and supporting clinical decision-making.