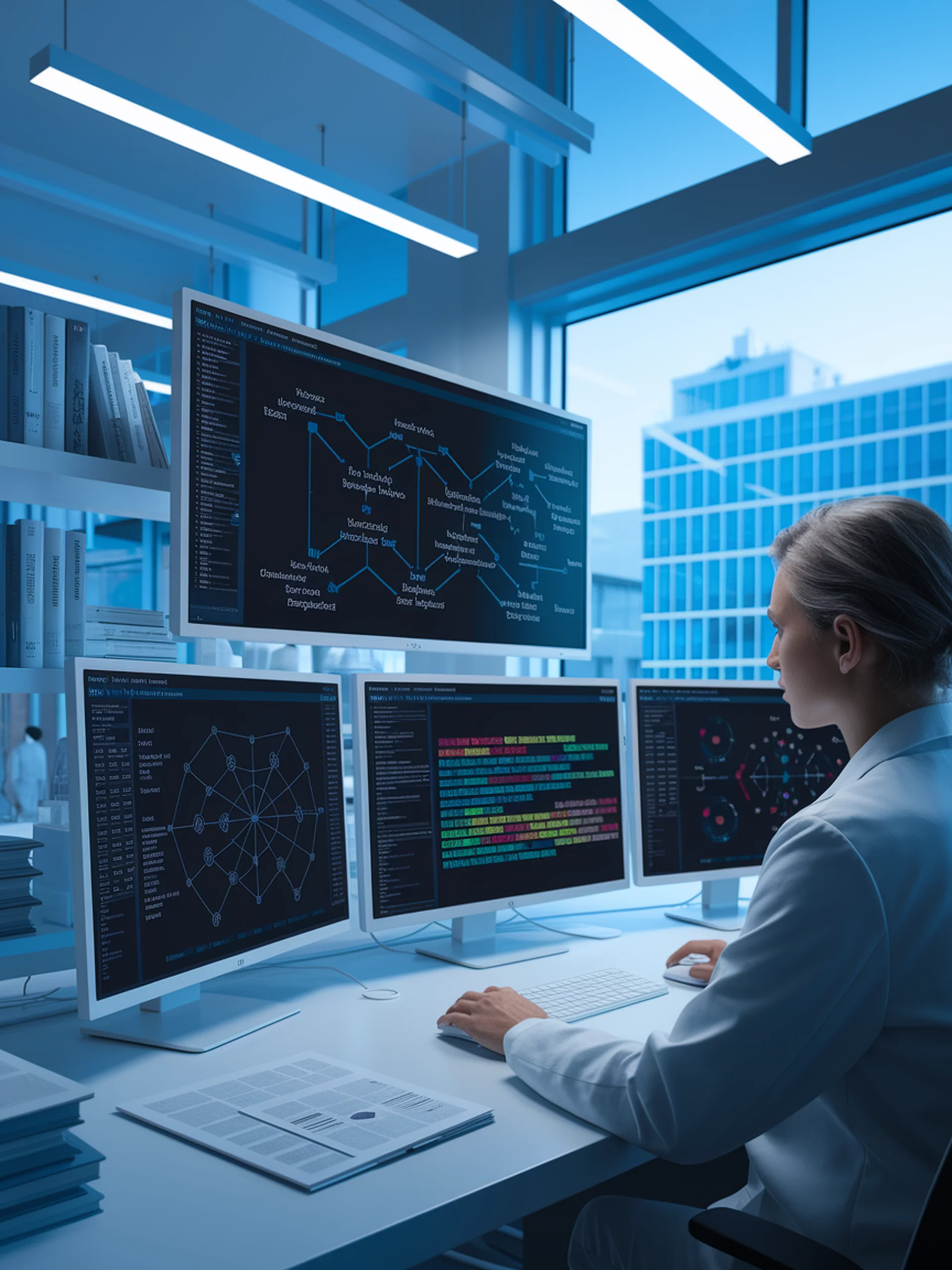
HILGEN: Enhancing Biomedical NER with Knowledge-Driven Data
Combining medical knowledge bases with LLMs for improved entity recognition
HILGEN introduces a novel approach that leverages both structured medical knowledge and LLMs to generate synthetic training data for biomedical named entity recognition tasks.
- Utilizes UMLS hierarchical structure to expand training with related medical concepts
- Employs GPT-3.5 to generate contextually-rich examples for rare medical entities
- Demonstrates significant performance improvements on multiple biomedical datasets
- Addresses the critical challenge of data sparsity in specialized medical domains
This research offers a practical solution for healthcare AI systems that need to accurately identify medical entities in clinical text, potentially improving clinical decision support, research, and medical information extraction.