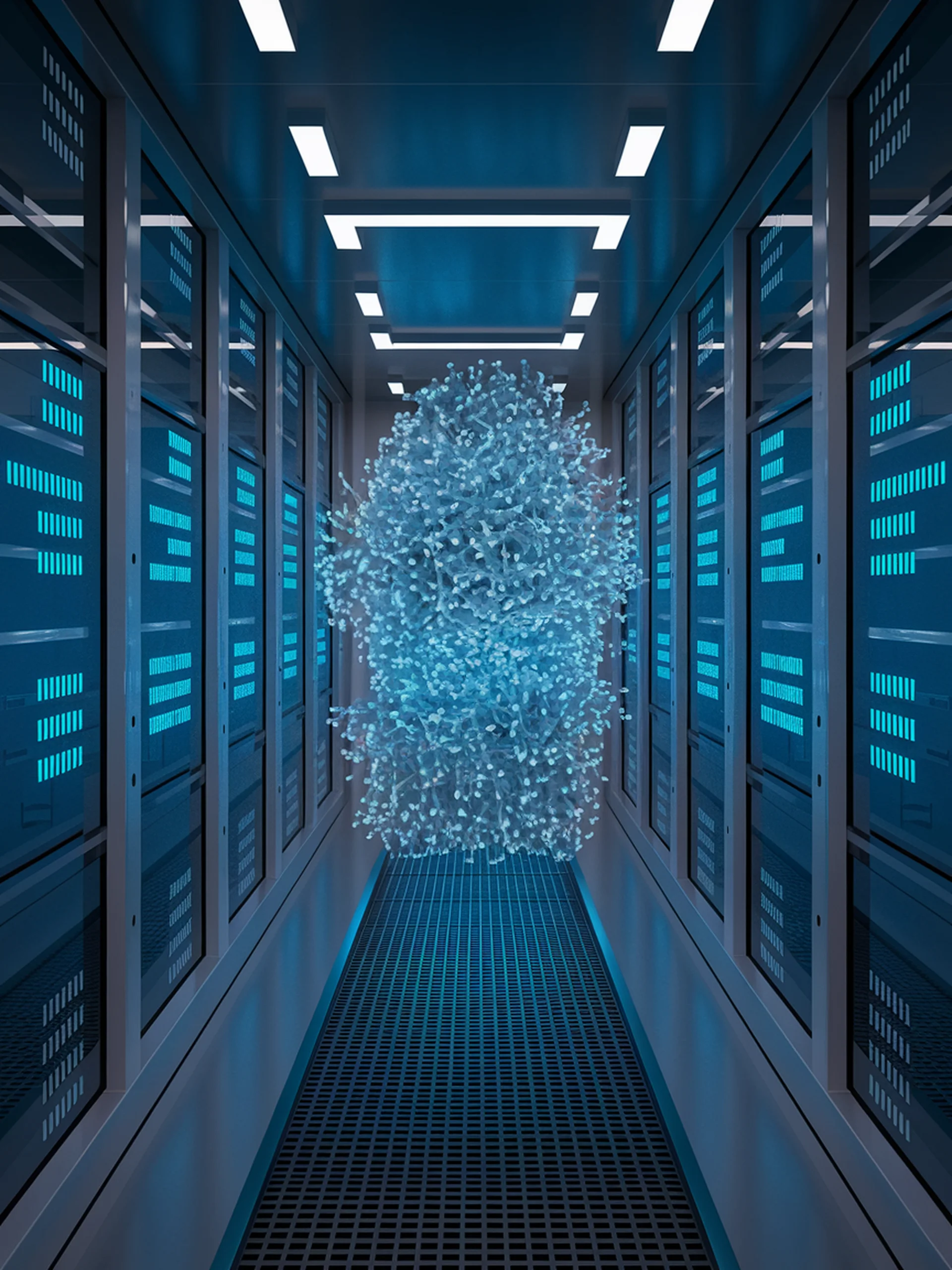
Enhancing Relation Extraction in Medical Data
A Novel Dual-Encoder Approach with Instance-Adapted Descriptions
This research introduces a dual-encoder architecture with instance-adapted predicate descriptions that significantly improves relation extraction in biomedical domains.
- Combines contrastive and cross-entropy loss for more effective fine-tuning of smaller encoder models
- Uses instance-specific predicate descriptions rather than fixed descriptions to better capture contextual relationships
- Demonstrates superior performance on biomedical relation extraction datasets
- Offers a more efficient alternative to large decoder-only language models
This advancement matters for medical data processing by enabling more accurate extraction of relationships between medical entities, supporting knowledge discovery and clinical decision-making applications.
Relation Extraction with Instance-Adapted Predicate Descriptions