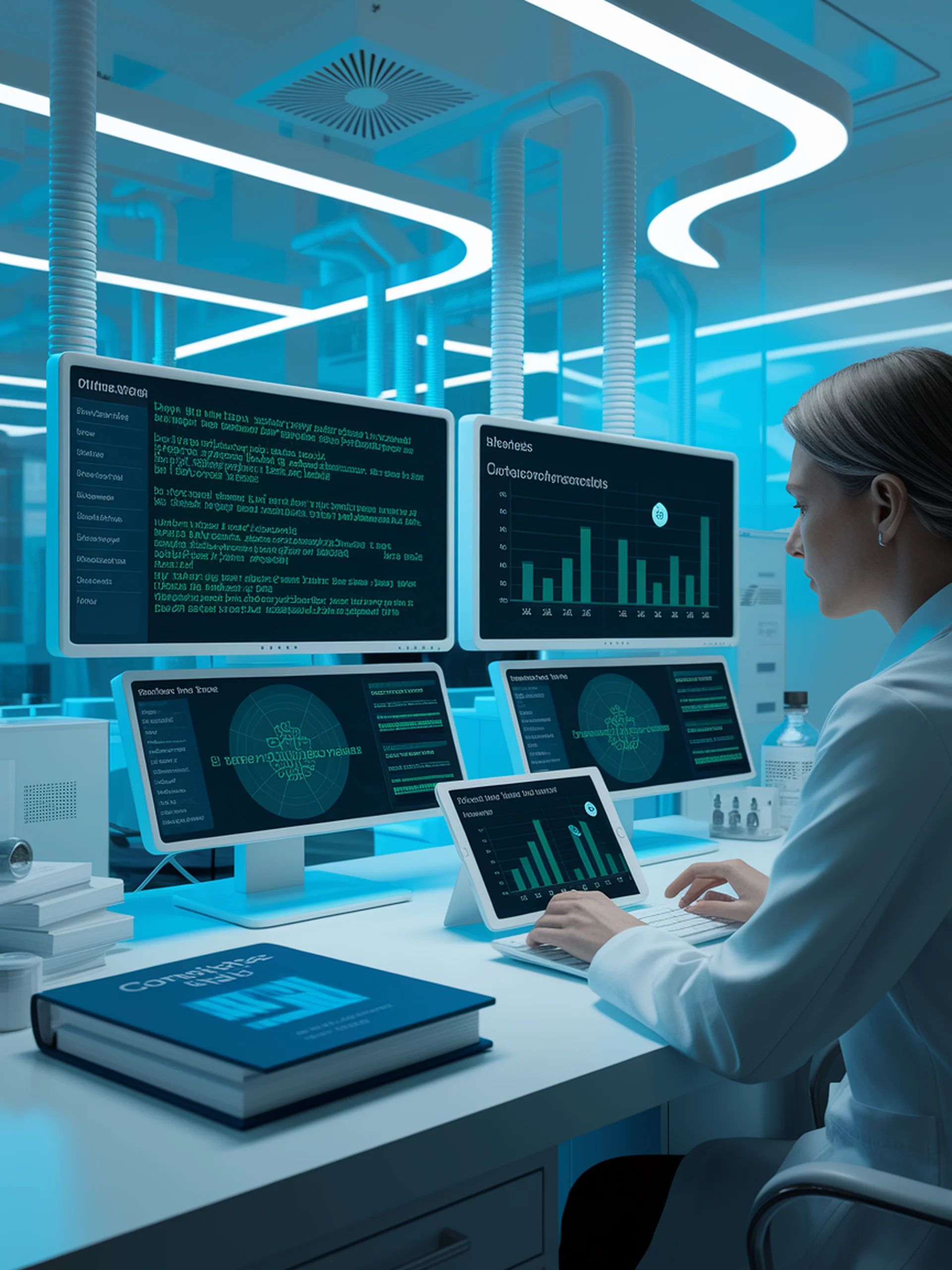
Boosting Clinical NLP Without Domain Expertise
Synthesized guidelines make LLMs better at medical information extraction
This research demonstrates how synthesized annotation guidelines can significantly improve large language models' performance in clinical information extraction without requiring deep domain knowledge.
- LLMs with synthesized guidelines outperform baseline few-shot approaches by 14.8% on clinical NER tasks
- The approach is knowledge-lite, eliminating the need for domain experts to create detailed guidelines
- Performance improvements are consistent across different model sizes and clinical datasets
- Guidelines synthesized by GPT-4 proved as effective as human-created guidelines
For healthcare organizations, this approach offers a cost-effective way to deploy advanced NLP solutions with minimal domain expertise requirements, potentially accelerating clinical data extraction and analysis workflows.
Synthesized Annotation Guidelines are Knowledge-Lite Boosters for Clinical Information Extraction