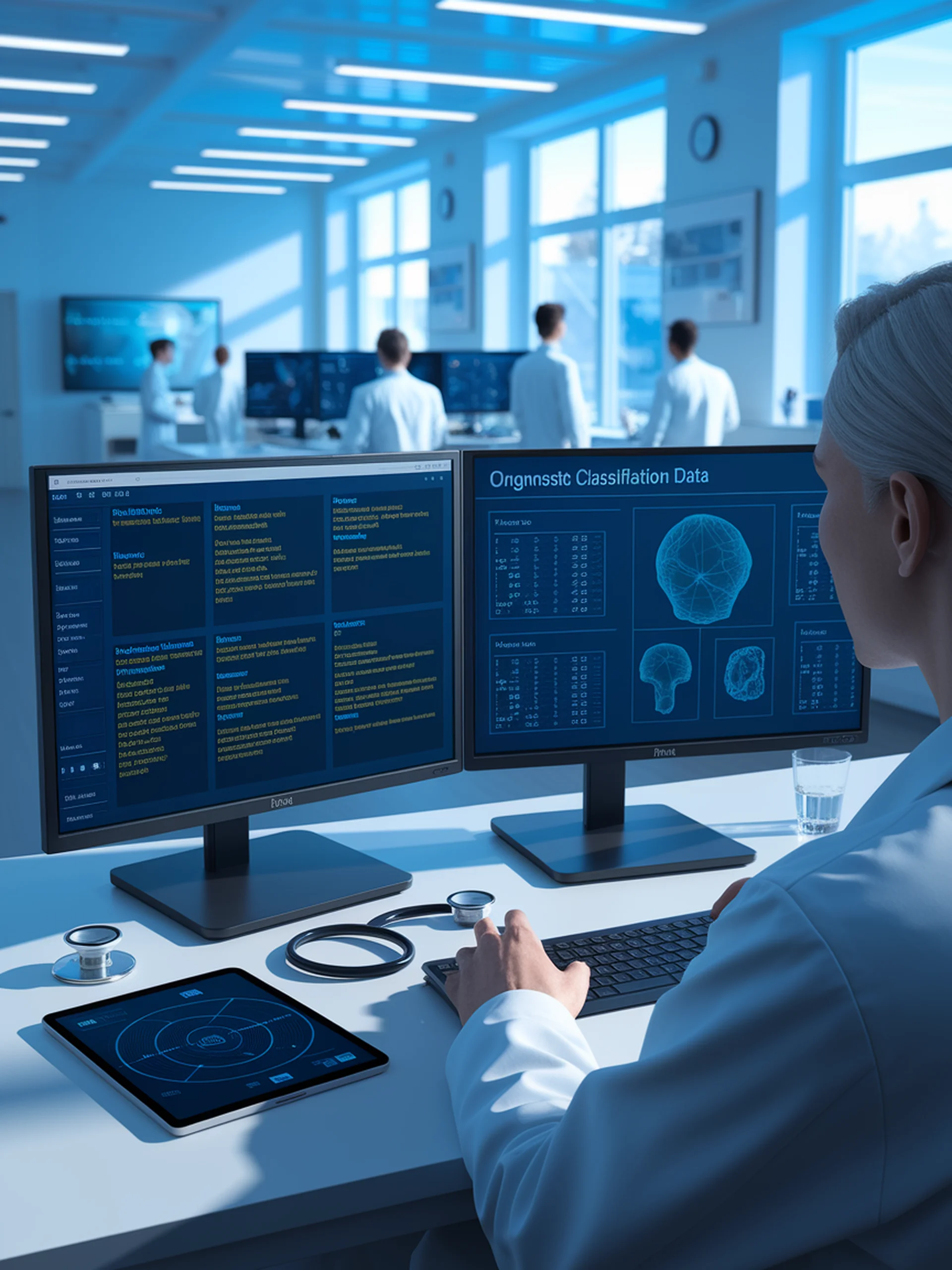
Enhancing Clinical Document Classification with Reasoning LLMs
Comparing reasoning vs. non-reasoning language models for medical diagnoses
This research evaluates how reasoning-enabled LLMs can improve the classification of clinical documents into standardized ICD-10 diagnoses, addressing challenges in healthcare information processing.
- Study compared 8 large language models: 4 with reasoning capabilities and 4 without
- Reasoning LLMs (like Qwen QWQ and Deepseek Reasoner) demonstrated superior performance in clinical document classification
- Models showed consistent accuracy across varying document complexities
- Zero-shot approaches achieved competitive results without requiring extensive training data
This research matters for healthcare because it offers a pathway to more efficiently process unstructured medical texts despite privacy constraints and limited annotated datasets, potentially improving diagnostic accuracy and workflow efficiency in clinical settings.
Original Paper: Can Reasoning LLMs Enhance Clinical Document Classification?