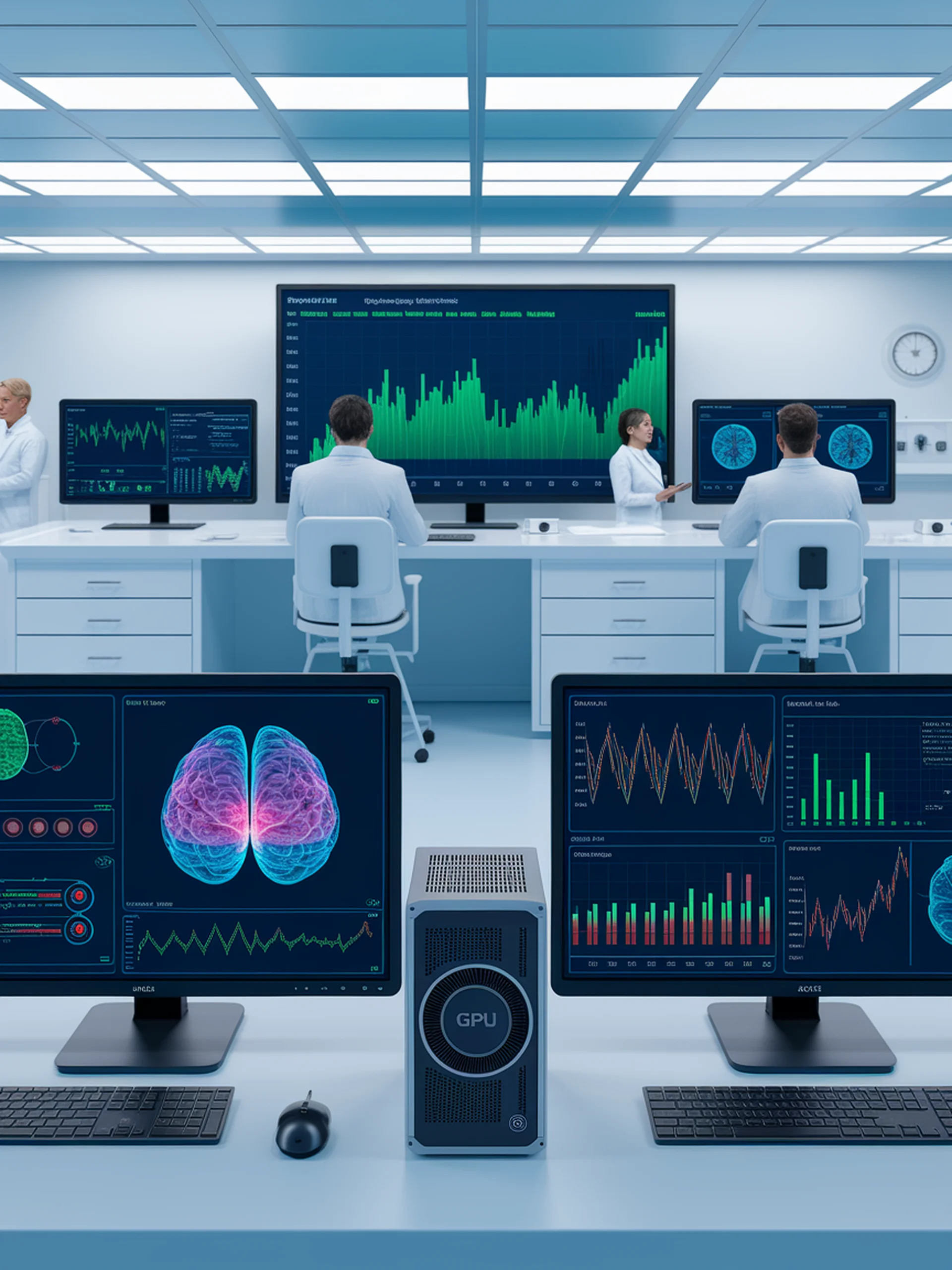
Efficient Clinical NLP at Scale
Hybrid approach reduces GPU demands while maintaining accuracy
This research introduces a hybrid NLP framework that makes advanced clinical text analysis accessible with lower computational requirements.
- Combines rule-based filtering, SVM classification, and BERT models to reduce GPU demands
- Achieved 95% accuracy in dementia identification with 75% reduction in computational resources
- Successfully deployed at scale across VA medical centers to analyze millions of clinical notes
- Demonstrates practical implementation for healthcare systems with limited computational resources
This innovation makes advanced NLP techniques accessible to more healthcare organizations, enabling widespread clinical text analysis for improved diagnosis and patient care without requiring expensive GPU infrastructure.